The realm of digital imaging is perpetually evolving, leveraging countless technological innovations to refine the way images are captured, manipulated, and presented. One such critical component propelling this progressive journey is the concept of stable diffusion.
Acting as a linchpin to higher quality image processing, stable diffusion fosters our ability to reduce noise and preserve edge details in digital images. Beginning with an exploration of digital imaging’s core tenets, this paper dives deeply into the foundations of stable diffusion, elucidates its mathematical underpinnings, and explicates the conditions necessary for stability.
Furthermore, it provides practical demonstration of its applications and its potentially transformative incorporation in the sphere of digital imaging.
Contents
Fundamentals of Digital Imaging
As the world continues to digitize, the field of digital imaging has become inseparable from many aspects of our technological engagement, including the intricate process known as stable diffusion. By examining the basic concepts of digital imaging and how they intersect with the stages of stable diffusion, one can gain a profound understanding of their critical interconnection.
Digital imaging, in essence, is the creating, processing, and manipulation of images utilizing computer algorithms. It underpins an extensive range of applications spanning across industries such as medical diagnostics, remote sensing, entertainment, and defense systems – to name but a few.
A fundamental component of digital imaging is the conversion of continuous images into numerical representations – a process coined as digitization. This step involves an image being broken down into minuscule elements named pixels, which are individually processed and analyzed using computer algorithms. The result is a digital image comprised of an enormous matrix of pixels, each bearing a unique numerical value that defines color, intensity or depth, allowing for a multitude of manipulations.
Stable diffusion, on the other hand, is a derivative of the principle of diffusion wherein substances move from areas of high concentration to areas of lower concentration until equilibrium is reached. This process is prevalent in numerous natural phenomena and scientific fields, one of which is digital imaging. Stable diffusion, as the name suggests, denotes a controlled method of diffusion that minimizes artifacts and distortions, yielding a more accurate, optimized image output.
In the context of digital imaging, stable diffusion underlies the task of image filtering, a crucial technique that amplifies or reduces specific features of a digital image. It leverages the statistical distribution of pixel values to identify and minimize noise, enhance edges, and increase contrast.
A closer look at the process reveals that stable diffusion employs differential equations to calculate the appropriate balance between image smoothing (reduction of details) and enhancement (preserving edges or features). This fine balance ensures the diffusion process does not overly distort the intended features and maintains the integrity of the image.
As a case in point, anisotropic diffusion, a specialized form of stable diffusion, stands as a paradigm of this application. It uses spatial information, and, relatedly, the concentration levels of pixel values within the image to perform selective smoothing. This strategy ensures that image details – such as edges – are preserved, producing an output image that maintains the fidelity of the original.
The intersection of digital imaging with stable diffusion showcases a marriage of physical principles with computational technology, a union yielding transformative dividends across a myriad of industries and research domains. Through an understanding of digital imaging fundamentals, scientists and engineers harness the power of stable diffusion to optimize image quality, precision, and clarity – thereby unlocking the bountiful potential that the digital age affords.
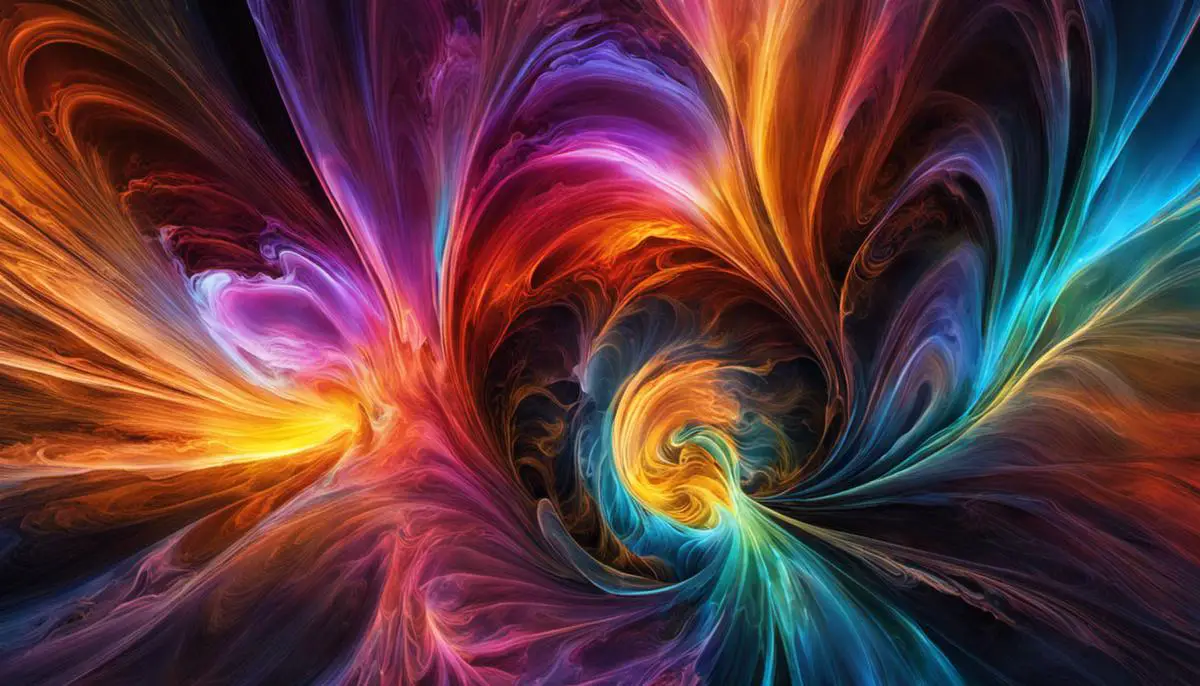
Understanding Stable Diffusion
To delve deeper into the subject of stable diffusion and digital images, it’s imperative to understand the characteristics of stable diffusion and its correlation with digital imaging. Notably, stable diffusion is an integral step in image processing, known for preserving the sharpness of digital images, while mitigating noise interference. In the realm of digital imaging, stable diffusion targets the objective of achieving an equilibrium, such that the image does not become excessively blurred or excessively noisy.
The application of stable diffusion in digital imaging exhibits the properties analogous to the dispersion of molecules in a fluid under osmotic pressure. This mathematical correlation with physical phenomena is where stable diffusion shines, delineating the gradients of images based on the intensity values while maintaining the structural attributes of the image. The sequence of diffusion process directs toward a steady-state solution, creating consistency and stability.
The convergence of stable diffusion in digital imaging relies heavily on an operator called the Laplacian. This second-order derivative operator permeates the image’s pixel intensity, serving as a kernel to the diffusion process that shapes the image.
In the domain of image filtering, stable diffusion stands apart from commonplace techniques due to its adaptability and sensitivity to the local gradient of an image. Hence, it attributes its name – “stable” – as it converges to an image that is neither over-smoothed nor unduly exaggerated in terms of features.
Advancing beyond this, the realm of anisotropic diffusion, also recognized as a specialized form of stable diffusion, equips digital imaging with superior dimensionality. By functioning in the direction of lowest intensity gradient, it sustains the edge boundaries which are key to the interpretability of the image content. This selective smoothing process amplifies the contrast between different regions, enhancing the visibility of intricate details.
The implications of stable diffusion for digital imaging stretch beyond the realms of theoretical understanding. With a profound impact on numerous industries and research domains, it stands as a cornerstone for innovations in fields such as medical imaging, remote sensing, computer vision, and more. Paving way for more precise interpretations and decisions, stable diffusion unequivocally marks a transformative stride in the scientific community’s endeavor to excavate deeper into the canvas of knowledge.
Lastly, acknowledging the symbiotic relationship between digital imaging and stable diffusion opens avenues to further exploration and research, enabling us to refine and deepen our mastery of this fascinating and consequential subject.
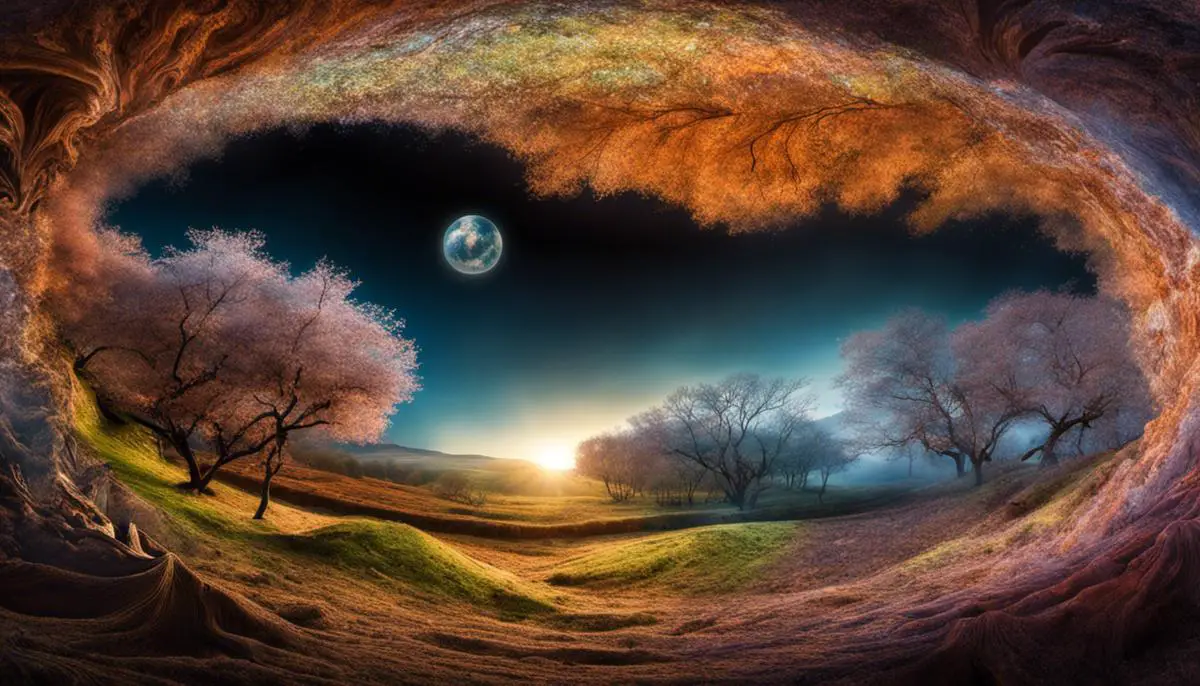
Application of Stable Diffusion in Digital Imaging
Following the core principles and applications of stable diffusion in digital imaging, it’s essential to delve deeper into the nuances. Understanding how stable diffusion aids in the preservation of image sharpness and the mitigation of noise interference is one such crucial facet.
The stable diffusion process provides a systematic approach in the delicate thriller of balancing image detail importance and noise reduction, without materially compromising the details.
Stable diffusion in the domain of digital imaging bears profound similarity to the natural diffusive process, where molecules in a fluid disperse under osmotic pressure. It is an insightful parallel that supplies an intuitive understanding of the process – in this case, image pixels being the equivalent of molecules, and the grayscale intensity functioning akin to the concentration gradient.
The Laplacian operator plays a pivotal role in the framework of stable diffusion, serving as an essential tool in image filtering. It functions as a mathematical expedient, highlighting changes or gradients within an image, which digitally signifies an “edge” or a stark shift in intensity.
These edge transitions are fundamental to explaining the relationship between an image’s various components. The Laplacian operator, through stable diffusion, helps to detect these critical boundaries, maintaining the image’s structural integrity.
An intriguing aspect of stable diffusion is its adaptability and heightened sensitivity to local image gradients. Rather than uniformly applying the same diffusion process across an entire image, the diffusion coefficient will be modulated based on the local image gradient, performing algorithms that account for differentiation in pixel intensities. In simpler terms, this means an image of a bustling cityscape will be treated quite differently than an image capturing the subtle gradients of a still-life painting.
The specialized form of stable diffusion, anisotropic diffusion, emphasis further on the preservation of edge boundaries and the enhancement of details within an image. It ingeniously maintains the clarity of structural edges, and fine-tunes the visibility of details that would be lost otherwise due to overlapping intensities or pixel distortions.
Importantly, stable diffusion finds paramount significance in sectors that heavily rely on clear, accurate imaging, such as medical imaging, remote sensing, and computer vision. The ability of stable diffusion to aid in more precise interpretations and decisions resonate profoundly in these fields. For example, in medical imaging, sharp and exact images are invaluable, affecting diagnosis accuracy and treatment efficacy.
Ultimately, the symbiotic relationship between digital imaging and stable diffusion has ushered a new realm of research and exploration, as each development in one domain opens a doorway to further application and innovation in the other. As our visual world continues to convert into digital formats, the role of sophisticated processes like stable diffusion becomes even more vital, enriching the digital imaging pioneers’ toolbox and sparking unprecedented investigations and findings in the visual representation’s captivating universe.
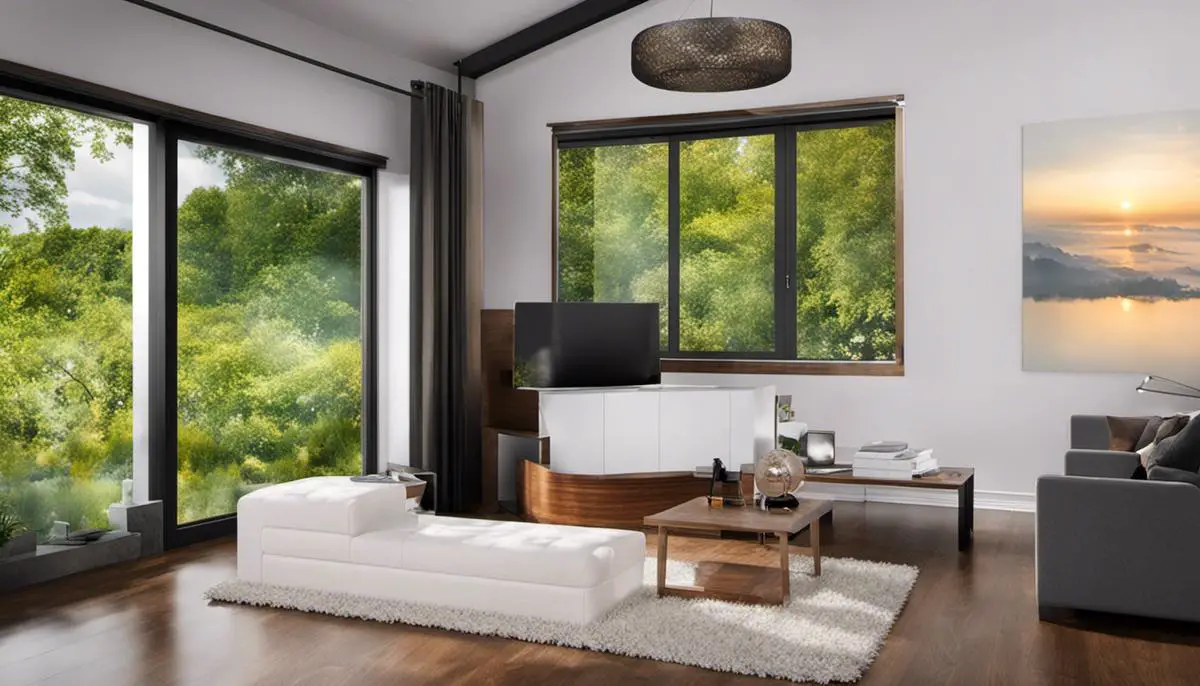
Advancements and Future Possibilities
Building upon the foundation established previously in the discussion of stable diffusion, and considering recent advancements in the field, a fascinating realm opens up for digital imaging. New methodologies and interpretations are emerging, built on enhancing diffusion algorithms and incorporating superior mathematical models to advance this unique field, thereby setting a bright path for future research endeavors.
A significant milestone involves the advent of stochastic resonance, a non-linear phenomenon pertaining to random signals. In the context of digital imaging and stable diffusion, stochastic resonance holds great potential. The inclusion of ‘noise’ in the imaging process can paradoxically augment the clarity of the features within an image. Application of stochastic resonance dexterity in balanced fusion between signal and noise has proven to be instrumental in enhancing digital image detection and extraction of intricate details.
Another evolution involves Machine Learning, particularly Deep Learning and Convolutional Neural Networks (CNN). CNNs have found particular utility in image classification and recognition tasks, drawing principles from stable diffusion. The architecture which imitates neural processes of the visual cortex fundamentally incorporates the iterations of smoothing and enhancing images, synonymous with the dynamics of stable diffusion.
Progressing in the same direction is the multi-scale modeling that explores stable diffusion. By adjusting diffusion parameters per the scales of operation, such as spatial or temporal, it offers a nuanced understanding of the digital image. Resolving granular details without distortion is now achievable with the effective implementation of multi-scale models.
Divergent growth in stable diffusion also exists in the form of edge-enhancement diffusion (EED). Derived from anisotropic diffusion, EED emphasizes edge boundaries, segments image regions, and enhances the contrast of the visualized data. This methodology could potentially revolutionize the way images are processed for a multitude of applications, including diagnostic imaging in healthcare, surveillance in security industries and pattern recognition in computer vision.
In the realm of mathematical models, Fractional Order Anisotropic Diffusion (FOAD), a recently ventured field, is garnering significant attention. The inclusion of fractional calculus in diffusion models facilitates the ability to manipulate diffusion rates as required, offering the level of adaptability to meet the demands of diverse photonic applications.
These advancements, alongside continuous improvements and explorations in the field, are presenting avenues unimagined, further solidifying the indispensable role of stable diffusion in reshaping digital imaging. The onward journey of stable diffusion is elevated by the integration of contemporary computational technologies and methodologies.
From the synthesis of mathematical prowess and technological proficiency, arises an architectural masterpiece – the future of stable diffusion in digital imaging. The ambit expands expeditiously, illuminating untapped avenues, thereby inviting passionate endeavors to unravel the secrets bound within each image pixel. The exhilarating ride through the cosmos of stable diffusion offers an unmatched scientific expedition for every curious mind. Indeed, the chronicle of the digital world continues.
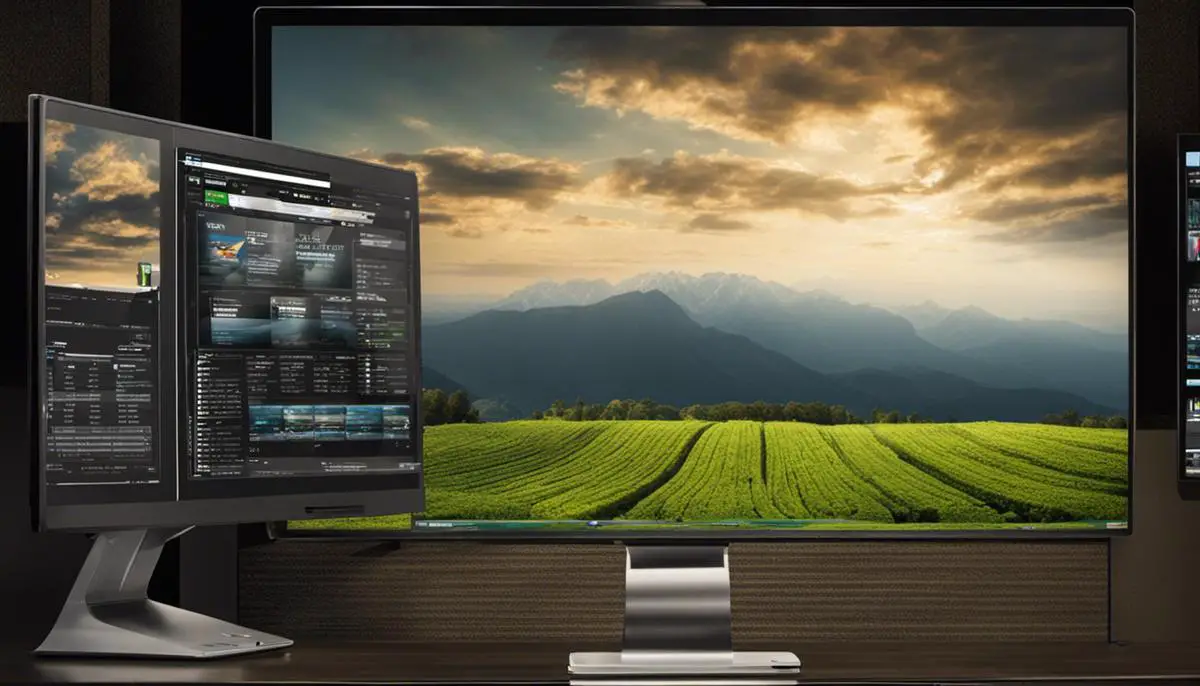
As we surge forward in the digital era, stable diffusion remains a key player in shaping the future trajectory of digital imaging. The potential utilization of computational technologies and machine learning paints a promising picture of how stable diffusion techniques could be enhanced.
Advancements in this domain could imply higher quality imaging, clearer visuals, and an elevated user experience, underlining its imperative role in driving digital imaging’s future possibilities. The journey has only begun, as we stand poised at the precipice of more efficient and impactful image-processing breakthroughs, fueled by the power of stable diffusion.
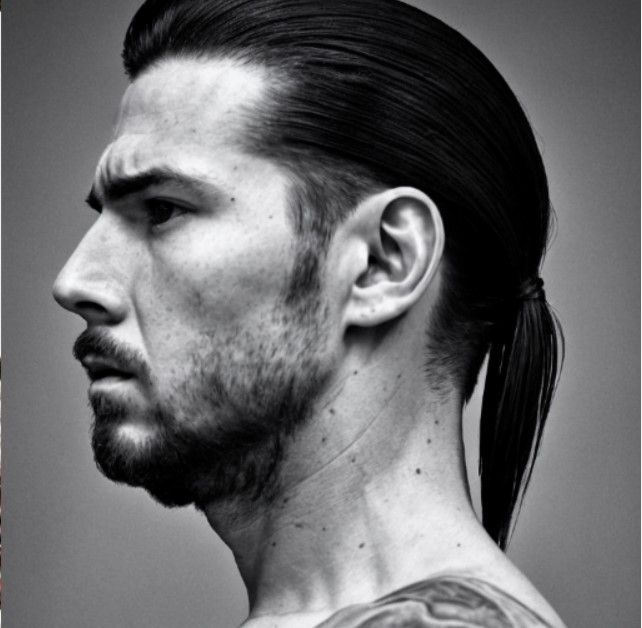
Emad Morpheus is a tech enthusiast with a unique flair for AI and art. Backed by a Computer Science background, he dove into the captivating world of AI-driven image generation five years ago. Since then, he has been honing his skills and sharing his insights on AI art creation through his blog posts. Outside his tech-art sphere, Emad enjoys photography, hiking, and piano.