In our rapidly advancing digital age, the quality of video content plays a key role in user experience across numerous industries, from entertainment to education. This pursuit of premium video quality drives constant innovation and improvement in video processing techniques.
One such pivotal concept in this realm is stable diffusion, a quintessential method for enhancing video quality. This paper will serve to elucidate the intricate aspects of stable diffusion, elucidating its fundamental principles, application in video processing, and significance in delivering high-quality visual content.
Additionally, it will delve into thought-provoking discussions pertinent to stable diffusion algorithms, the challenges, limitations of the method, and its exciting future scope in the context of emerging technologies.
Contents
Fundamentals of Stable Diffusion
Fundamental Principles of Stable Diffusion in Video Processing: A Holistic Understanding
The realm of video processing has dramatically evolved over the past few decades, propelled by a fascination with human perception and continuous advances in digital technology. One of the critical components of this evolution is the stable diffusion process – a process intrinsically linked to image enhancement, edge detection, and noise reduction among other functionalities.
Stable Diffusion: An Overview
Stable diffusion in video processing is a mathematical model that assists in the analysis of changes in pixel intensities within an image or a sequence of frames. This technique applies the principles of diffusion — often associated with the passive spread of particles leading to equilibrium — to the realm of digital image and video processing.
This process, despite its seeming simplicity, is built upon several robust and intricate principles. Understanding these fundamentals can unravel the intricacies of how visuals are enhanced and optimized in the digital world.
Principle of Spatial Consistency
This principle posits that pixels in closer proximity to each other tend to have similar intensities. Thus, during diffusion, the transfer of intensity from a pixel to its neighboring pixel ensues along a path of less resistance, ensuring a stable, uninterrupted diffusion process.
Principle of Anisotropy
Anisotropy suggests that diffusion is not uniform in all directions. In image and video processing, this principle allows differentiation between features such as edges or textures. The diffusion coefficient can be adjusted according to the gradient magnitude in such a way that it decreases near edges, preserving them during diffusion.
Principle of Time-Dependent Diffusion
Stable diffusion also entails a dynamic, time-dependent process. This principle determines the impact of multiple iterations of diffusion, crucial for video processing where a temporal sequence of frames necessitates a cumulative diffusion effect.
Invariant Convexity Principle
The assumption of invariant convexity principle holds that a convexity or concavity present in the initial data will be preserved under diffusion. Consequently, such geometric properties within an image or video frame are maintained, ensuring stability in the diffusion process.
Principle of Local Variation
The process hinges on the idea of minimizing the variation of the intensity function locally, leading to the fundamental least squares method. By adopting this principle, stable diffusion assists in reducing noise and other anomalies.
As researchers and innovators concoct fresh techniques and technologies in video processing, the understanding and application of these fundamental principles remain paramount. After all, it is their enforcement that brings about the precision necessary for enhancements and optimizations, while also maintaining the stability, integral to the diffusion process.
These principles of stable diffusion live in each frame of a high-resolution video, an advanced AR interface, or a cutting-edge feature film. Unseen but invaluable, they continue to shape the digital landscapes we traverse daily, highlighting the profound potential and expansive horizons of video processing.
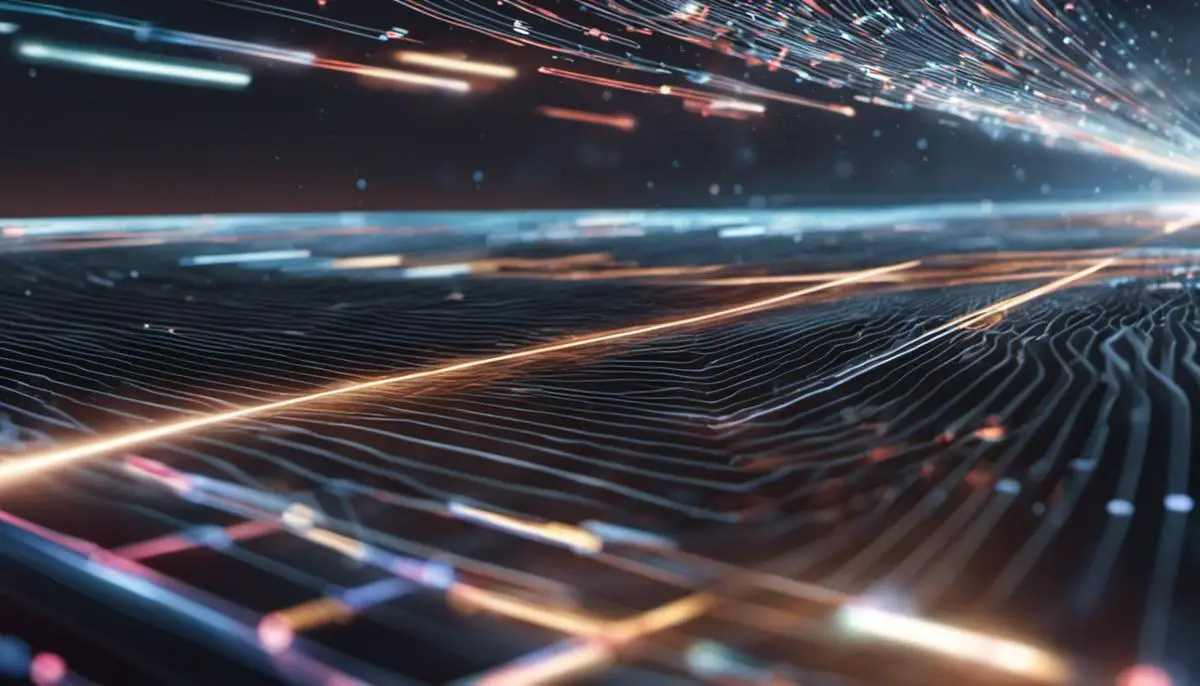
Role of Stable Diffusion in Video Processing
The fascinating role that stable diffusion plays in the realm of video processing has not gone without its due regard. Gearing towards the realm of performance enhancement, stable diffusion significantly progresses the precision, clarity, and smoothness associated with video processing tasks.
On a broader spectrum, the success of video processing pivots around a few valuable principles that have already been introduced, including spatial consistency, anisotropy, time-dependent diffusion, invariant convexity principle, and local variation. It would be remiss not to bridge these key elements to our topic of interest: the performance-enhancing contribution of stable diffusion.
Stable diffusion stands as a robust image descriptor due to its strong statistical properties. These properties encompass power-spectral measures, favorable de-noising features, and existence of solutions in transitory conditions. It is here where the crux of the performance enhancement resides, as these attributes contribute to the overall quality of video processing.
However, how does this occur? Let’s explore the intrinsic properties pertinent to stable diffusion. Notably, these properties do not divert from the laws of physics, enhancing the plausibility and credibility of the outcomes. An image, be it static or dynamic (video), comprises physical attributes manifesting into what we shall call the spatial domain. Stable diffusion respects the real-world constancies, thereby reducing the risk of any possible distortion.
The introduction of anisotropic diffusion, on the other hand, implies the dependence of diffusion on the image’s structure or content. Stable diffusion herein guarantees the non-distortion of predominant image or video structures. This quality makes it indispensable in applications such as edge preservation, a key factor often deserving prime focus in video processing.
Stable diffusion also acts as the primary powerhouse for noise suppression; one of its key features that significantly reflects in image and video processing efficiency. As noise is a common element affecting videos captured in various environments, the capabilities of stable diffusion underline the coveted enhancement in video processing performance.
Moreover, its impact is impeccably visible in the preservation of textures and structures even in the most challenging conditions. With the absence of stable diffusion, the video may lose its inherent textures during processing, leading to insufficient detail extraction. However, with the introduction of stable diffusion, texture conservation is remarkably attained, directly contributing to the performance of video streams.
One might surmise that the principles of invariant convexity and time-dependent diffusion add considerably to video processing enhancement. While time-dependent diffusion aids in maintaining smooth temporal transitions – an absolute necessity in video processing – invariant convexity assists in retaining the original shapes and dimensions, pivotal in conserving information during the processing phase.
Finally, the principle of local variation supports the concept of stable diffusion in amplifying performance. The local differences in frame sequences are conserved via a stable diffusion approach, resulting in an improved extraction and interpretation of image features and details.
In conclusion, the role of stable diffusion and its underlying principles in enhancing video processing performance remains not only pivotal but becomes integral. Whether in noise suppression, texture conservation, or edge preservation, stable diffusion brings a significant contribution to the realm of video processing, paving the way towards a clearer, smoother, and more reliable visual experience.
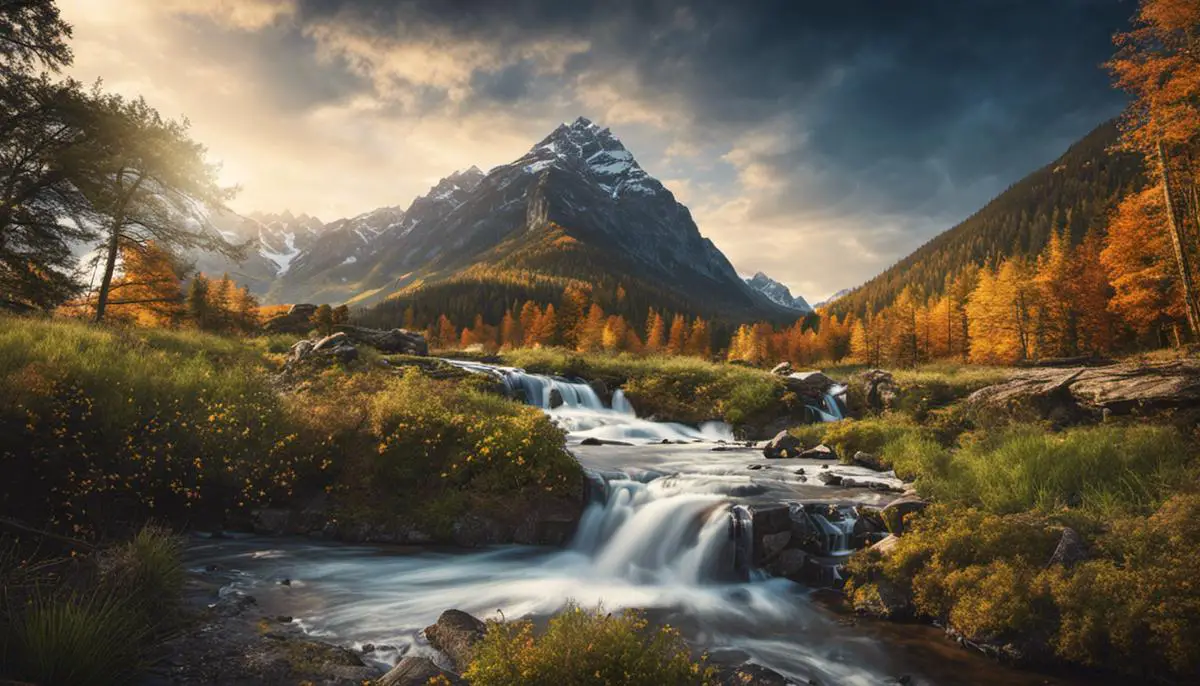
Stable Diffusion Algorithms
Building on the foundation of fundamental principles in stable diffusion, a crucial theory in video processing, we now delve further into the innovative algorithms and computational processes employed in this discipline. The heart of this subject matter lies in the effective management of data transfer, paramount to preserving structure and clarity in digitized imagery.
Integral to the fabric of this dance between mathematics and visual arts are algorithms such as Perona-Malik, Speckle Reducing Anisotropic Diffusion (SRAD), and Coherence-Enhancing Diffusion Filtering (CEDF). Each offering unique approaches brings forth qualities of time-dependent diffusion, invariant convexity, and local variation in presenting a refined and polished visual output with diminished distortions.
The Perona-Malik Algorithm, named after its developers, represents an exemplary figure in the arena of anisotropic diffusion. Through a careful and systematic approach to data diffusion, it allows for variance in the diffusion coefficient, thereby optimally preserving the structural integrity of image edges. This algorithm thus echoes the principles of anisotropy and local variation by differentiating between vital and trivial information, favoring lesser diffusion in areas of high contrast and more in less significant regions.
Meanwhile, the Speckle Reducing Anisotropic Diffusion (SRAD) algorithm leans on the principle of spatial consistency. With a design rooted in the degradation of noise or speckle, SRAD also simultaneously maintains image details, purely by exploiting the speckle statistics of the image itself. The SRAD algorithm admirably showcases stable diffusion’s power in noise suppression and texture conservation.
Coherence-Enhancing Diffusion Filtering (CEDF) further contributes to this field’s dynamics, focusing predominantly on the enhancement of flow-like structures in an image. This algorithm emphasizes the importance of invariant convexity in stable diffusion, filtering noise and unwanted details while preserving and enhancing significant structures. This algorithm thus emphasizes the field’s pursuit for crystal-clear visual coherence.
Algorithms such as these, and the computational processes that underpin them, are pillars supporting the vast edifice of stable diffusion. On traversing this landscape from spatial consistency to local variation, the discovery of these computational joys brings us face-to-face with the very artistry of mathematics, etched in the fine lines of our digital world.
As we understand these operational tools better and dig deeper into this wellspring of information, a new perspective on this discipline begins to crystallize. The potential for discovery and innovation in stable diffusion is infinitely exciting, the implications resonating far beyond video processing, reaching across disciplines and sectors to a horizon that extends as far as our curiosity dares to dream.
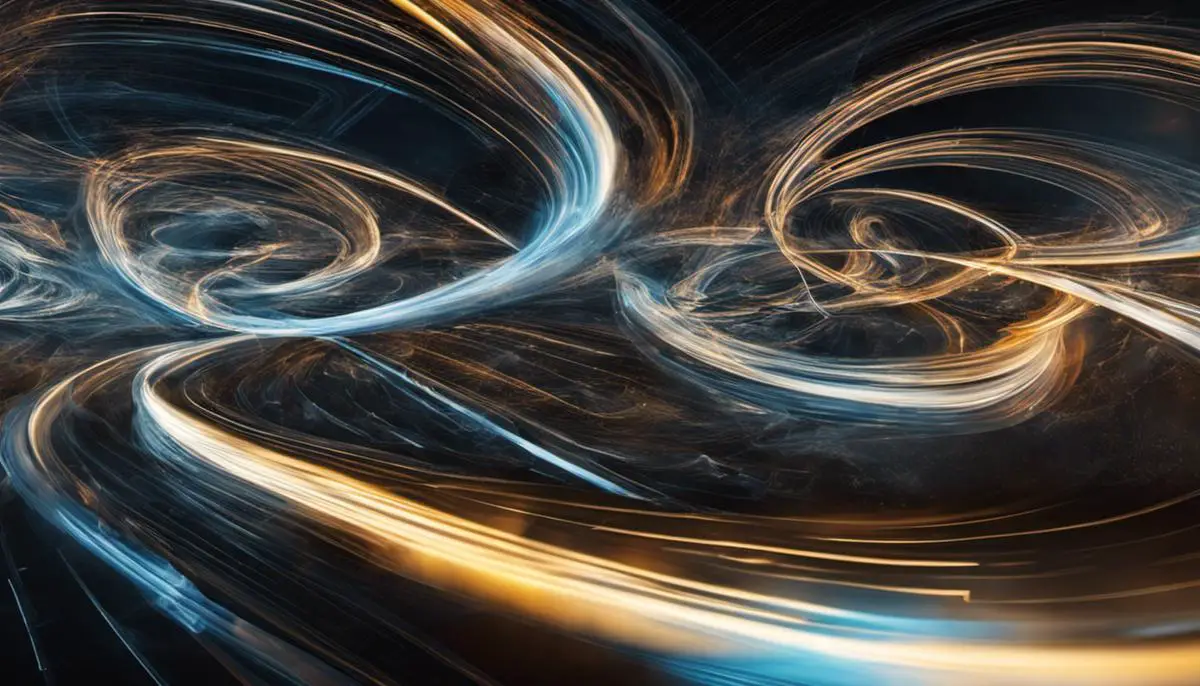
Challenges and Limitations of Stable Diffusion
Despite the vast merits and extensive utilization of stable diffusion in video processing, challenges and limitations persist in its application, thereby opening up avenues for in-depth research and technological advancements. These challenges mainly arise due to inherent complications in perfectly exemplifying spatial, temporal, and perceptual aspects of image data within the constraints of mathematical and computational systems.
A significant hurdle pertains to processing speed. Efficient real-time execution of stable diffusion algorithms remains elusive. The complexity and computational cost associated with stable diffusion algorithms, especially during real-time video processing, often lead to temporal inconsistencies in resultant frames. An immediate requirement lies in establishing less computationally intensive algorithms that maintain the quality of video processing outputs.
Moreover, the identification and accurate demarcation of the boundaries within an image or video, despite considerable advancements in anisotropic diffusion techniques, remain problematic. Further improvements in methodologies for maintaining edge sharpness and detail while removing noise are necessary.
Another challenge is the poor handling of textures in stable diffusion processes. Often, the objective to reduce noise and preserve significant structures in an image leads to the inadvertent blurring of intricate textures. Emerging solutions focus on adaptive schemes that account for varying local statistics or multiscale approaches, but their full integration remains an ongoing research interest.
The lack of a universal diffusion function stands as another limitation. The impact of the diffusion function on the processing outcome necessitates the choice of an optimal diffusion function for different image content and requirements. The absence of a universally recognized diffusion function compels the use of trial and error, increasing processing time and complicating automation.
Historically, stable diffusion techniques have been less effective in high-noise environments. The utility of time-dependent diffusion and invariant convexity principles have offered some respite, but in conditions with an elevated noise level, the noise suppression capacity of such techniques often falls short.
Lastly, the application of stable diffusion in color video processing poses a unique challenge. The process typically involves separate diffusion processes for each color channel, leading to color artifacts due to inter-channel inconsistencies. While several strategies towards color video processing using stable diffusion have been proposed, none has emerged as a definitive solution.
Overcoming these challenges necessitates broader perspectives, inclusive research, advanced technology, and continued dedication. The development of innovative algorithms and computational processes that consider the aesthetic, content-specific, and temporal dynamics of videos will result in improved video processing experiences. The era of technological evolution demonstrates a promising future to rectify the limitations of stable diffusion in video processing, and continual exploration of this line of research carries exceptional potential.
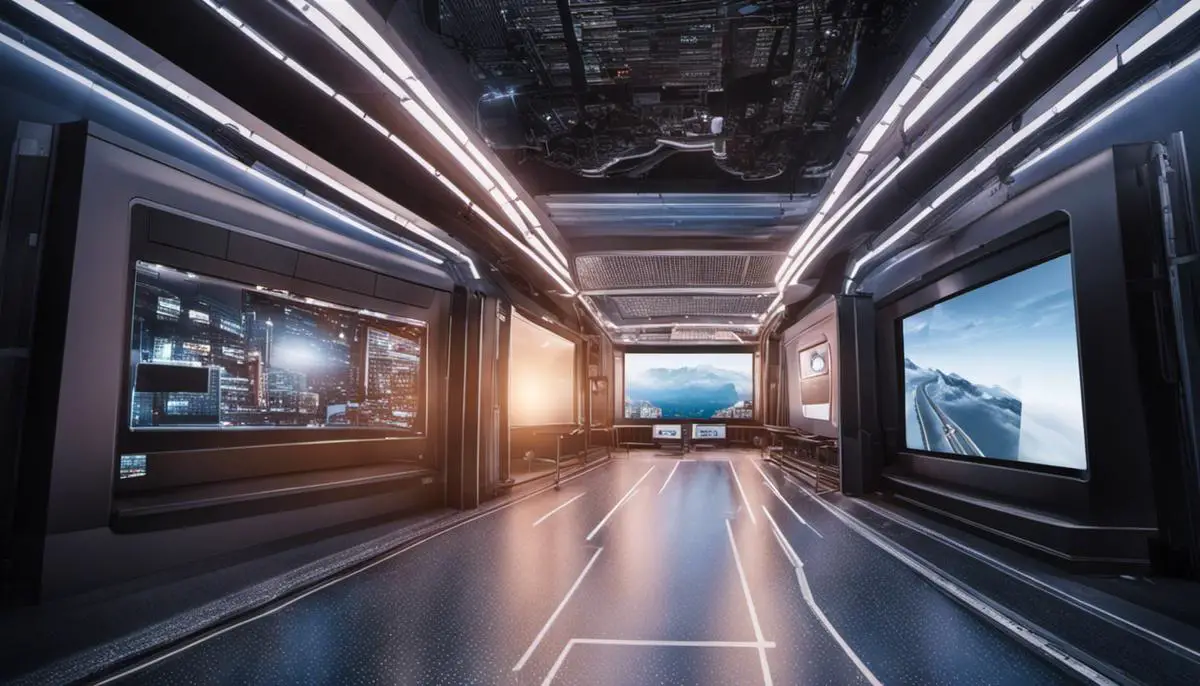
Future of Stable Diffusion in Video Processing
The Future of Stable Diffusion in Video Processing: Ambitions and Challenges
Inevitably, the landscape of video processing is adapting, growing, and innovating at a break-neck pace. Stable diffusion remains central to this evolution, championing quality enhancements, detail preservation, and noise reduction. As we cast eyes towards the future, it is feasible to envisage an arena forever shaped by the progression and optimization of stable diffusion techniques.
High-resolution footage and ever-developing video architecture herald new opportunities for further development of sophisticated stable diffusion algorithms. Resolution advancements extend the detail-retention capabilities of stable diffusion, fostering even clearer images. This technological escalation not only promises new heights in visual clarity but also promotes the expansion of stable diffusion theory with expansive, multi-faceted data layers.
The integration of Artifical Intelligence (AI) and Machine Learning (ML) paradigms in stable diffusion offers exciting prospects. Current developments focus on adaptive systems capable of learning and evolving to enhance video processing performance. AI and ML-based stable diffusion algorithms could outperform traditional counterparts, providing flexible and advanced solutions to video noise suppression, distortion reduction, and texture conservation challenges.
The growing impact of stable diffusion is not confined to video processing. Other fields, such as computer-aided design, virtual reality, and mobile imaging, are exploring their own arenas with tools borrowed from stable diffusion techniques. The versatility and adaptability of stable diffusion fill our arsenal with robust utilities ready for deployment in digital frontiers yet to be explored.
Despite these prospective advancements, the road ahead is not without its obstacles. Processing speed presents a significant challenge, particularly in real-time applications. Increasing computational efficiency without sacrificing quality remains a priority to counteract these challenges. Moreover, stable diffusion techniques must continue to improve in the detection and delineation of boundaries within images and videos. This capability is crucial for producing images of unprecedented precision and clarity.
Texture interpretation remains an Achilles heel for stable diffusion, particularly in videos with complex or intricate textures. Extending the domain of stable diffusion to accommodating complex textures may call for fresh mathematical approaches, underpinning a new generation of algorithms.
The triumph of stable diffusion in color video processing is hampered by inherent difficulties in color noise detection and removal. Improved noise profiles and advanced algorithmic structures could provide an impetus to overcome this challenge.
The face of stable diffusion is not set in stone, and neither is its future. The strength of its promise depends on the persistence of our investigations, the inclusiveness of our quests, and the innovativeness of our solutions. Reinforcements in the form of advanced technology, superior algorithms, novel mathematical approaches, and a firm commitment to research can usher in a new epoch for stable diffusion.
The future of stable diffusion extends far beyond a blur of algorithms and computations. It introduces us to a realm where the elegance of visual processing blends seamlessly with scientific rigor and mathematical finesse, offering a symphony of clarity, precision, and beauty.
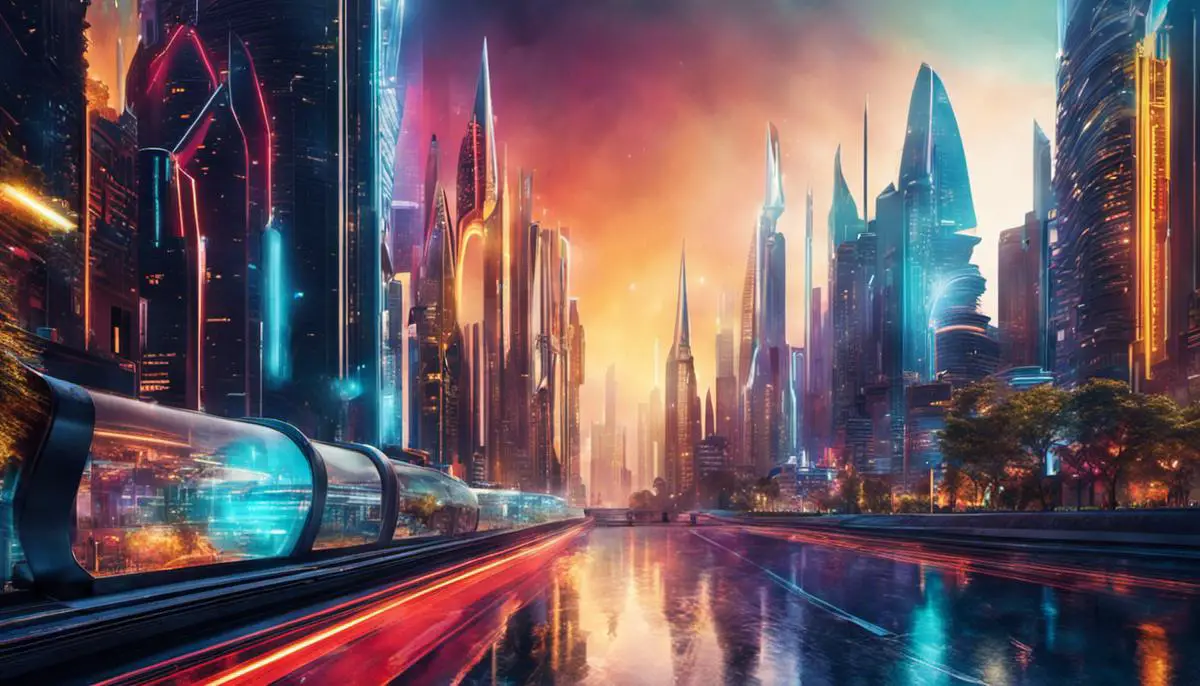
As the digital foundation of our world continues to evolve, the application and understanding of stable diffusion in video processing will undoubtedly become increasingly important. The marriage of stable diffusion with emerging technologies such as deep learning and artificial intelligence is pushing the boundaries of video quality to new frontiers.
The challenges present today will yield to the relentless exploration of solutions, paving the way for more sophisticated, yet user-friendly applications. In the ever-changing arena of video processing, the ongoing refinement and innovative application of stable diffusion consistently promise to transform the way we perceive and interact with the digital realm.
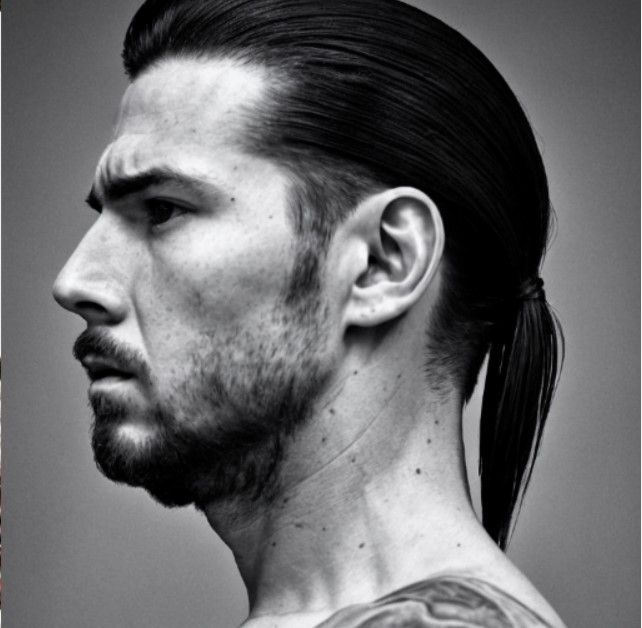
Emad Morpheus is a tech enthusiast with a unique flair for AI and art. Backed by a Computer Science background, he dove into the captivating world of AI-driven image generation five years ago. Since then, he has been honing his skills and sharing his insights on AI art creation through his blog posts. Outside his tech-art sphere, Emad enjoys photography, hiking, and piano.