The pursuit of generating high-quality images often encounters the challenge of computational limitations- LDM. However, a groundbreaking technique called Latent Diffusion Models (LDMs) has emerged, offering a solution to these computational barriers. In this article, we will explore the magic of LDMs and how they are revolutionizing the field of AI Imagery by breaking through these computational barriers and unlocking new possibilities for high-quality image generation.
Contents
The Challenge of Computational Limitations
AI Imagery tasks, such as image synthesis, inpainting, and super-resolution, often require extensive computational resources. Traditional approaches struggle to generate high-quality images due to the complexity of these tasks, leading to lengthy training times and resource-intensive computations. These computational barriers restrict the scalability and efficiency of image generation, hindering advancements in the field.
Enter Latent Diffusion Models (LDMs)
LDMs have emerged as a game-changing technique, addressing the computational limitations in AI Imagery. The magic of LDMs lies in their ability to optimize computational resources without compromising image quality. By training in the latent space of pretrained autoencoders, LDMs retain exceptional quality and flexibility while significantly reducing the computational requirements. This breakthrough allows for high-quality image synthesis even with limited resources, unlocking new horizons in AI Imagery.
Efficiency without Sacrificing Quality
LDMs excel in achieving the delicate balance between computational efficiency and image quality. By leveraging the principles of Stable Diffusion, LDMs preserve intricate details, capture underlying patterns, and enhance visual fidelity while minimizing computational burdens. This efficiency translates into faster training times, quicker inference, and the ability to generate high-quality images in a resource-efficient manner.
Enabling Large-Scale Image Generation
One of the remarkable aspects of LDMs is their capacity to enable large-scale image generation. Traditionally, generating high-resolution images with fine details was a computationally demanding task. However, LDMs break through these barriers by leveraging their latent space modeling and sequential denoising autoencoders. This enables the generation of visually stunning, high-resolution images without requiring excessive computational resources.
Expanding Possibilities in AI Imagery
The magic of LDMs extends beyond computational efficiency. By breaking through these barriers, LDMs open up new possibilities in AI Imagery. They empower researchers, artists, and enthusiasts to explore more complex and creative image synthesis tasks, such as layout-to-image generation, text-to-image synthesis, and beyond. LDMs unleash the potential for generating diverse and visually compelling images with reduced computational constraints.
The Future of LDMs
As LDMs continue to advance and evolve, they hold the key to overcoming computational barriers in AI Imagery. Ongoing research and innovation in LDMs aim to further optimize their efficiency, expand their capabilities, and push the boundaries of image synthesis. With the magic of LDMs, we can expect a future where high-quality, visually stunning images are accessible to a wider audience, driving advancements in various domains of AI Imagery.
Conclusion
The magic of LDMs lies in their ability to break computational barriers and revolutionize AI Imagery. By optimizing computational resources without sacrificing image quality, LDMs unlock new possibilities for high-resolution image generation, efficient image modification, and creative exploration. As researchers and enthusiasts continue to harness the power of LDMs, we can look forward to a future where the magic of AI Imagery is accessible to all, transforming the way we create, perceive, and interact with visual content.
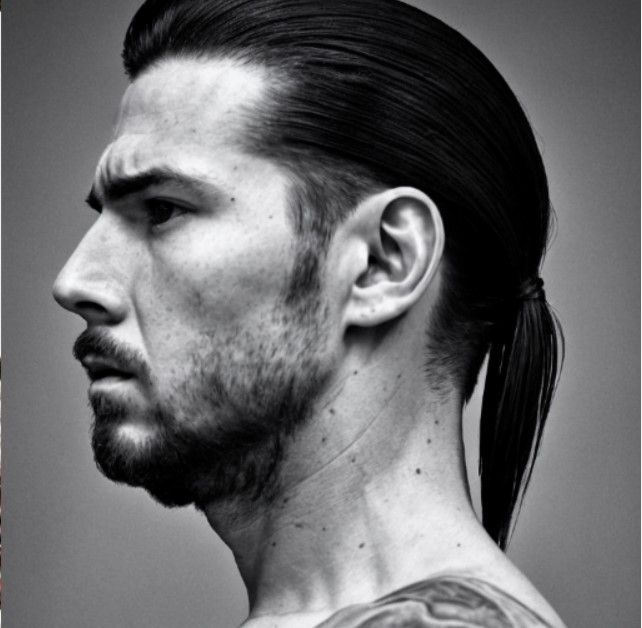
Emad Morpheus is a tech enthusiast with a unique flair for AI and art. Backed by a Computer Science background, he dove into the captivating world of AI-driven image generation five years ago. Since then, he has been honing his skills and sharing his insights on AI art creation through his blog posts. Outside his tech-art sphere, Emad enjoys photography, hiking, and piano.