In the field of AI Imagery, the ability to generate visually stunning and realistic images is a significant goal. Diffusion Models (DMs) have emerged as a powerful technique for image synthesis, but their capabilities have been further elevated with the introduction of Cross-Attention Layers. In this article, we delve into the transformative impact of Cross-Attention Layers on Diffusion Models, exploring how they enhance the power and flexibility of image generation.
Contents
Understanding Diffusion Models (DMs)
Diffusion Models have revolutionized image synthesis by decomposing the image formation process into sequential applications of denoising autoencoders. These models excel in capturing intricate details and generating visually compelling images. However, their original formulation operated primarily in pixel space, limiting their flexibility and conditioning capabilities.
The Power of Cross-Attention Layers
Cross-Attention Layers have emerged as a groundbreaking addition to Diffusion Models, transforming them into powerful image generators. These layers introduce the ability to incorporate conditioning inputs, such as text or bounding boxes, into the synthesis process. By integrating Cross-Attention Layers into the model architecture, Diffusion Models become flexible and adaptable, capable of generating images conditioned on diverse inputs.
Enhanced Conditioning and Flexibility
With Cross-Attention Layers, Diffusion Models can now generate images based on a wide range of conditioning inputs. Whether it’s text descriptions, semantic segmentation maps, or other spatial cues, Cross-Attention Layers allow the model to attend to specific regions of the input and incorporate the desired information into the synthesis process. This enhanced conditioning capability enables fine-grained control over the generated images, opening up new possibilities for creative exploration.
Enabling High-Resolution Synthesis
One of the remarkable advantages of Cross-Attention Layers is their ability to facilitate high-resolution image synthesis. Diffusion Models, powered by Cross-Attention Layers, can generate images with intricate details and realistic textures at resolutions that were previously challenging to achieve. The convolutional manner in which Cross-Attention Layers operate allows for efficient and scalable high-resolution synthesis, pushing the boundaries of image generation.
Applications and Future Directions
The integration of Cross-Attention Layers into Diffusion Models expands their applications across various domains of AI Imagery. From unconditional image generation to tasks like inpainting and super-resolution, these enhanced models deliver exceptional results. Researchers are continuously exploring novel architectures and conditioning mechanisms to further improve the capabilities of Diffusion Models with Cross-Attention Layers, promising exciting advancements in the field of image generation.
Conclusion
Cross-Attention Layers have transformed Diffusion Models into powerful image generators, revolutionizing the capabilities of AI Imagery. By incorporating conditioning inputs and enabling high-resolution synthesis, these enhanced models offer fine-grained control over image generation and open up new creative possibilities. As researchers and practitioners continue to harness the power of Cross-Attention Layers, we can expect further advancements in the field of AI Imagery, pushing the boundaries of image generation and delivering visually stunning results.
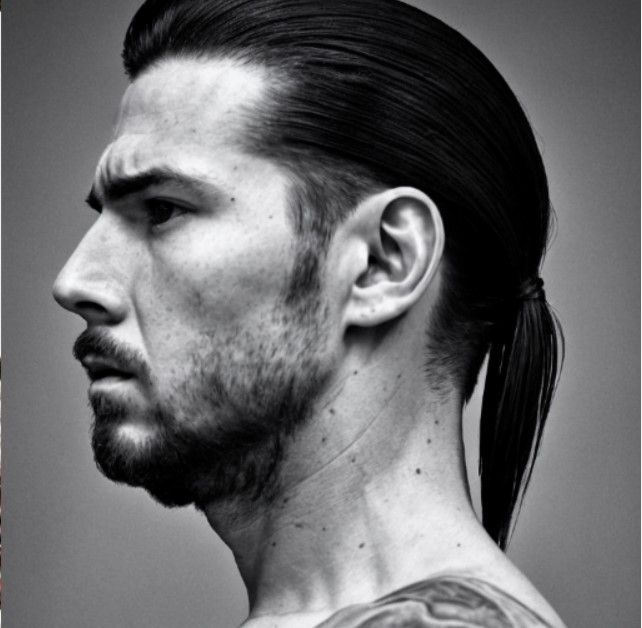
Emad Morpheus is a tech enthusiast with a unique flair for AI and art. Backed by a Computer Science background, he dove into the captivating world of AI-driven image generation five years ago. Since then, he has been honing his skills and sharing his insights on AI art creation through his blog posts. Outside his tech-art sphere, Emad enjoys photography, hiking, and piano.