3D image processing and the art of stable diffusion coalesce into an intricate space of technological marvel. The marriage of these sophisticated concepts has led to an unprecedented advancement in how we perceive and process depth and detail in images, thereby elevating our digital interaction to a whole new level.
From the raw understanding of stable diffusion, to employing it in image processing techniques and contending with the inherent challenges, an in-depth journey into this realm introduces its paramount significance. Furthermore, as we sit in the cusp of a 3D revolution in a variety of sectors, it is interesting to unwrap the potential influence of stable diffusion in future applications.
Contents
Stable Diffusion
Stable Diffusion and its Profound Influence on 3D Image Processing
Despite the ubiquity of three-dimensional (3D) images in our everyday lives, from medical scans to video games, it’s remarkable how few understand the intricate processes that go behind their creation and refinement. Of these multifarious processes, one specific procedure holds a level of importance that is unmatched – stable diffusion. This remarkable technique stands, quietly unassuming, at the core of an array of 3D imaging activities.
In the field of computational mathematics and image processing, stable diffusion is a wholly important and exploratory domain that has been researched profusely over the past few decades. It’s a fundamental concept derived from diffusion, a generic term that refers to the process by which particles in a system move from areas of high concentration to areas of low concentration over time, seeking equilibrium.
Stable diffusion is employed significantly in a nonlinear model of diffusion termed ‘Perona-Malik diffusion’ – a model that effectively bridges the concepts of heat conduction and edge detection in image processing. However, while conventional diffusion processes can inadvertently blur edges and fine details in images, stable diffusion manages to circumvent these pitfalls, maintaining the integrity and clarity of these salient image features.
By utilizing the principles of stable diffusion, researchers and engineers can heighten the precision and quality of 3D image processing. The primary method to achieve this is through the creation of a precise balance between the rates of diffusion within the image. The basic principle is that one maintains a higher diffusion rate in homogeneous regions to reduce noise, while simultaneously preserving a lower diffusion rate at the edges to maintain image detail.
The bearing of stable diffusion on 3D image processing is enormous and far-reaching. In medical imaging, for example, stable diffusion permits medical professionals to look at the anatomical structures of the human body with unprecedented accuracy and clarity, thereby significantly improving diagnostic and treatment initiatives. In the arena of animation and video gaming too, stable diffusion techniques impart images with a crispness and lifelike quality that is inherently superior to those created with conventional methods.
In the proceeding years, as technology continues to proliferate and we embark further into the realms of advanced 3D imaging, the role of stable diffusion and its profound influence on image quality will only augment. With the increasing reliance on 3D imaging across industries and a contiguous evolution of stable diffusion techniques, we can look forward to an even greater surge in image quality, precision, and lifelikeness in the future.
This pathway of thought represents an intriguing and promising branch of scientific inquiry. By no means is it complete – the work to refine the process of stable diffusion and further optimize its implementation in 3D image processing is ongoing. Such is the beauty and tenacity of scientific exploration.
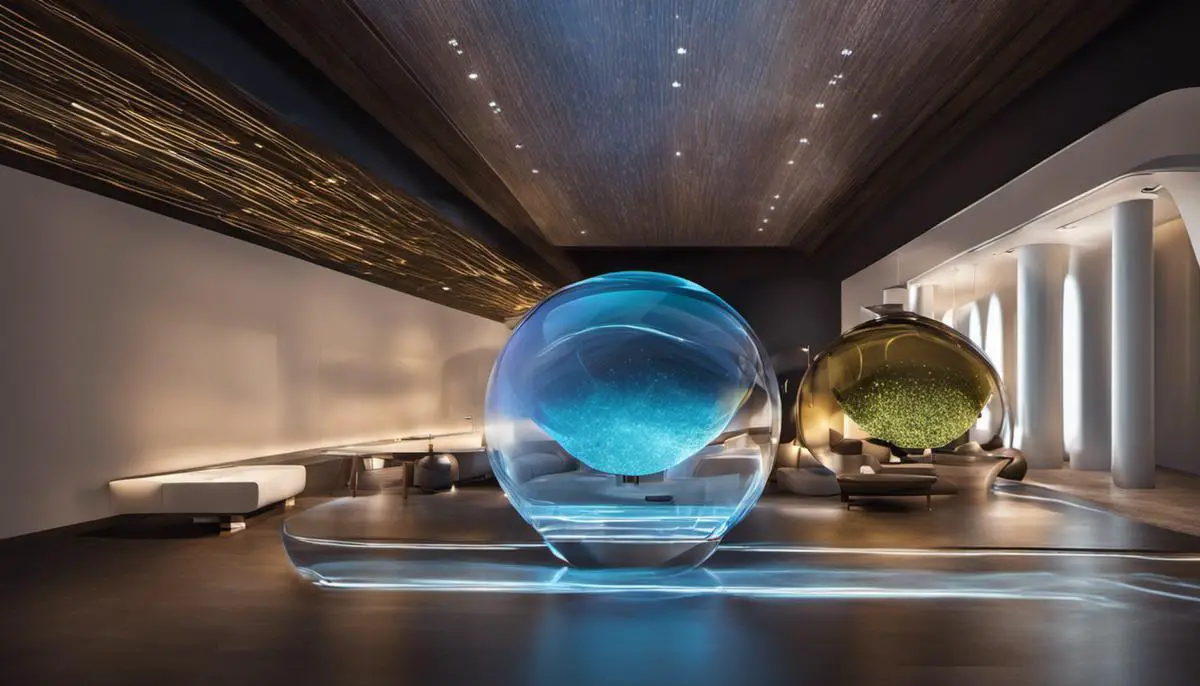
3D Image Processing Techniques
The article now delves into various techniques utilized in three-dimensional (3D) image processing, and how these methods, particularly, benefit from the application of stable diffusion. The term “stable diffusion” invokes an idea of order and constancy, an element paramount in the realm of computational mathematics and 3D image processing.
A fundamental technique used in 3D image processing involves filtering, which is compellingly intertwined with stable diffusion. Processing filters employ this approach to eliminate noise contamination. Median and Gaussian filters are common examples that make use of stable diffusion to improve image quality, realizing an accurate rendering of 3D images.
Additionally, stable diffusion emerges as a pivotal component in feature extraction within 3D image processing. This technique enables distinguishing entities based on their definitive characteristics, including edges, contours, and textures. In this context, stable diffusion offers consistent and precise extraction regardless of variance in image patterns, thus lending enhanced credibility to the results.
In volume rendering, another irrefutable technique in 3D image processing, stable diffusion plays an essential role. This method translates a 3D image onto a two-dimensional projection, an undertaking that typically requires intensive computation. The consistent nature of stable diffusion ensures such a transformation is accurate and smooth, preserving essential image features while minimizing computational errors.
Segmentation is another technique in 3D image processing that profits enormously from stable diffusion. Segmentation method partitions a digital image into different regions or multiple objects for further analysis or rendering. Dealing with complex and varied image patterns, stable diffusion upholds uniformity and precision in the segmentation process, thus improving the overall quality and accuracy of objects being segmented.
There are multiple pathways where stable diffusion can contribute to improving anisotropic diffusion methods, potentially advancing computational efficiency. As research progresses, it is even encapsulating neural network methodologies and machine learning algorithms, offering a fascinating insight into how stable diffusion could shape future developments in 3D image processing.
Lastly, with the evolution of virtual reality and augmented reality technologies, stable diffusion becomes the cornerstone, ensuring precise and dynamic rendering of virtual environments. As these technologies become omnipresent in various sectors, like simultaneous localization and mapping (SLAM) systems, stable diffusion’s application in 3D image processing will only gain more traction.
In conclusion, stable diffusion has embedded itself in the heart of 3D image processing techniques. It provides a quantifiable improvement to task execution, enhancing computational reliability while gracing the field with possibilities for future advancements. However, the true scope of stable diffusion remains boundless, with further exploration paving the way to unearth its potentials fully.
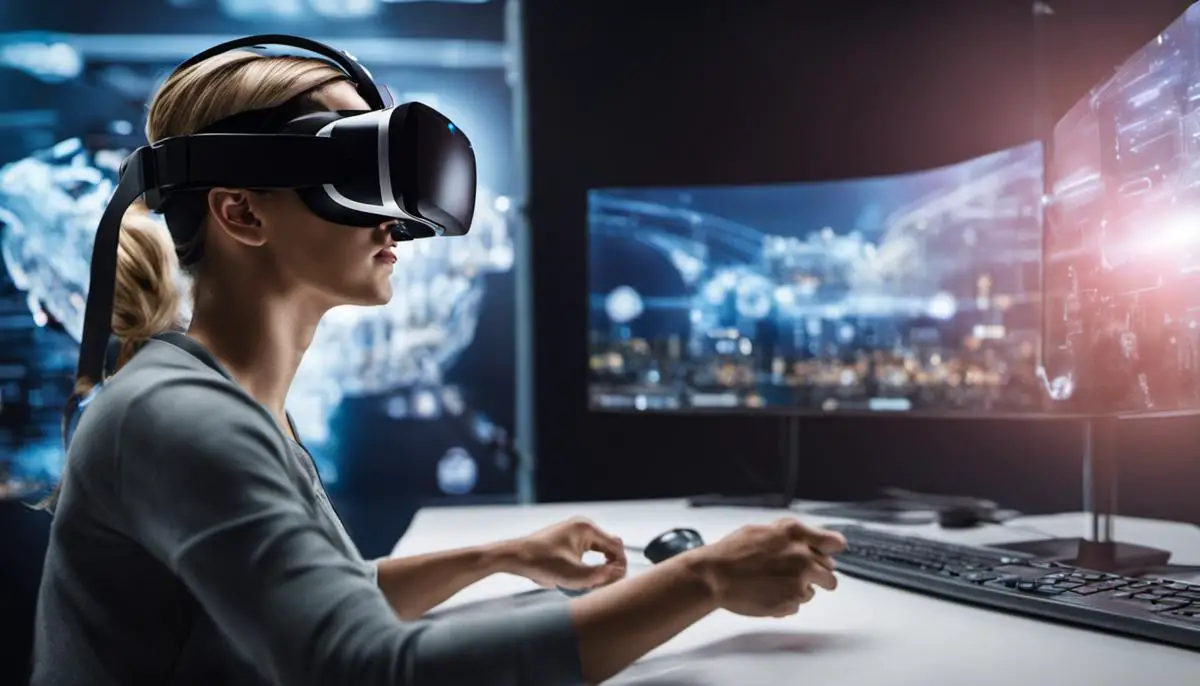
Challenges in 3D Image Processing with Stable Diffusion
Addressing the Challenges in 3D Image Processing with Stable Diffusion
In the progressive field of 3D image processing, it is customary to encounter a cascade of challenges that demand innovative strategies and advanced solutions. One poised area of intense research and development is the stable diffusion technique, employed in intricate processes ranging from filtration to volume rendering. The journey ahead, though paved with hurdles, is rich with discovery and advancement. These potential barriers should not be viewed as restraints but as platforms upon which new heights of technological sophistication can be reached.
When considering challenges in 3D image processing with stable diffusion, an immediate difficulty emerges in managing high computational demands. These are inherently linked with the detailed execution of algorithms associated with diffusion processes in three dimensions. These extensive calculations not only require substantial amounts of computational power, but also significantly elongate processing periods, making real-time 3D image processing a weighty task.
Furthermore, the precision required in stable diffusion techniques constitutes a spirited challenge. 3D imaging inherently involves a drastic increase in data complexity over simpler 2D imaging techniques. When this complexity couples with the precise control needed to balance the diffusion process, it births a challenging environment for algorithm formulation and application.
This precision is not strictly a mathematical concern; it is pivotal in the various arenas where stable diffusion is applied. For instance, in medical imaging, a minute lapse of precision could be the difference between a correct diagnosis or a possible misinterpretation.
Another hurdle one cannot ignore is the difficulty posed by noise present within 3D images. Unlike 2D images, where noise presents itself usually on an individual layer, noise in 3D images can manifest across multiple layers, creating a more sophisticated problem. With stable diffusion techniques, a delicate balance must be upheld to limit noise interference while preventing the over-smoothing of vital image data.
Alas, the challenge is not limited to practical application, but extends to teaching and learning. The integration of machine learning models into stable diffusion methods magnifies the learning curve for those new to the field. The inherent complexity of understanding and applying machine learning techniques couples with the intricacies of stable diffusion, forming a potentially formidable barrier to entry.
Nevertheless, the state-of-current limitations should not overshadow the value and potential stable diffusion brings to 3D image processing. These challenges, though considerable, serve as beacons guiding the direction of research, instigating advancements and innovating new methodologies.
Indeed, current and future innovators in this field should perceive these struggles as opportunities. Higher computation demands may drive the creation of hardware specifically designed for 3D image processing. The precision and noise issues can usher in next-level algorithms and models, while education-focused initiatives can simplify and democratize the approach to learning these complex techniques.
Ultimately, overcoming these challenges broadens our understanding and application of stable diffusion in 3D image processing, opening a pathway towards remarkable future developments in this electrifying field.
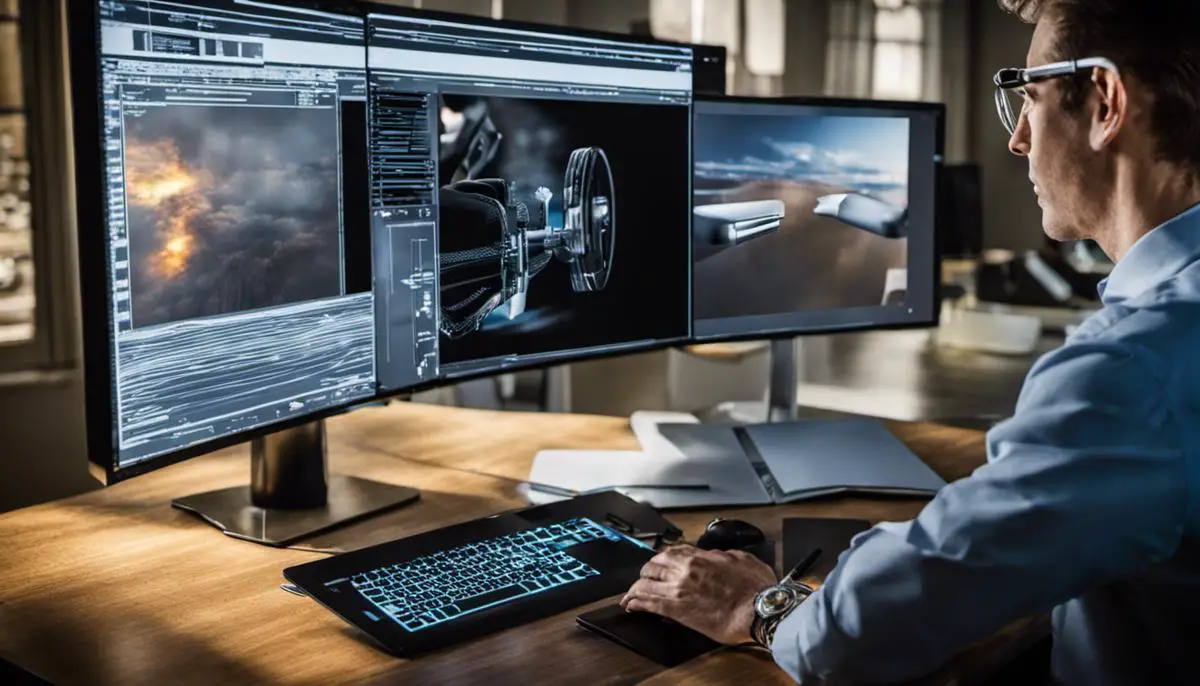
Emerging Trends and Future Applications
Recognizing the vibrancy they bring to our understanding of the world, three-dimensional (3D) images have inspired novel methods of processing beyond traditional techniques. One such advancement is the application of stable diffusion, a phenomenon that has punctuated a surge of new developments in this field. Discerning both present and future trends, it becomes evident that the potential impact of stable diffusion on future applications is immense and far-reaching.
One emerging trend is the incorporation of stable diffusion techniques into super-resolution imaging. These are sophisticated imaging techniques that overcome the traditional resolution limit set by the diffraction of light. As these images contain a higher density of information, processing can be demanding. Stable diffusion provides a more efficient method for processing these images, maintaining the highest quality while minimizing the computational load.
Another exciting trend is integrating stable diffusion methodologies with modern, predictive analytical models. An example lies within the realm of deep learning techniques, where stable diffusion has shown promising potential to robustly handle high volumes of layer and parameters. With further refinements, it could become an integral part of training neural networks for image processing applications.
Moreover, the exponential increase in volume data generated, particularly within healthcare and life sciences, has necessitated the development of dedicated algorithms for smooth and stable diffusion. Such continuous scale-space representations, enabled by stable diffusion, secure greater precision in identifying image features and in creating more accurate 3D reconstructions.
Yet, it is not without challenges. As with any rapidly progressing innovation, computational demands, precision requirements, and noise interference pose obstacles to the widespread adaptation of these technologies. The specialization in hardware and the required knowledge to manipulate such algorithms have limited their application within a wider user base.
However, it is evident that tackling these obstacles is not just promising but also probable. The prospect of hardware advancements aimed at catering to the high computational demands is an exciting frontier. Likewise, innovations driven by the intersection of different research areas, such as genetic algorithms and machine learning, can offer solutions to balance the diffusion process more accurately and handle the noise interference efficiently.
On another front, education and democratization of these complex learning processes, by launching simplified computational mathematics and programming modules, can open the field to a larger pool of innovators. Furthermore, expansive opportunities still linger for the application of this technology in virtual and augmented reality, creating advancements that could dramatically transform our interaction with the digital world.
In essence, the potential impact of stable diffusion in 3D image processing is beyond a singular application or industry. As we venture deeper into the scientific study of this phenomena, it provides the platform for a seamless blend of computational mathematics with image processing techniques, enforcing resilience in data analysis and expanding the frontiers of human knowledge. Thus, stable diffusion in 3D image processing is not just an emerging trend but a pathway to creating a more precise, efficient, and visually rich future.
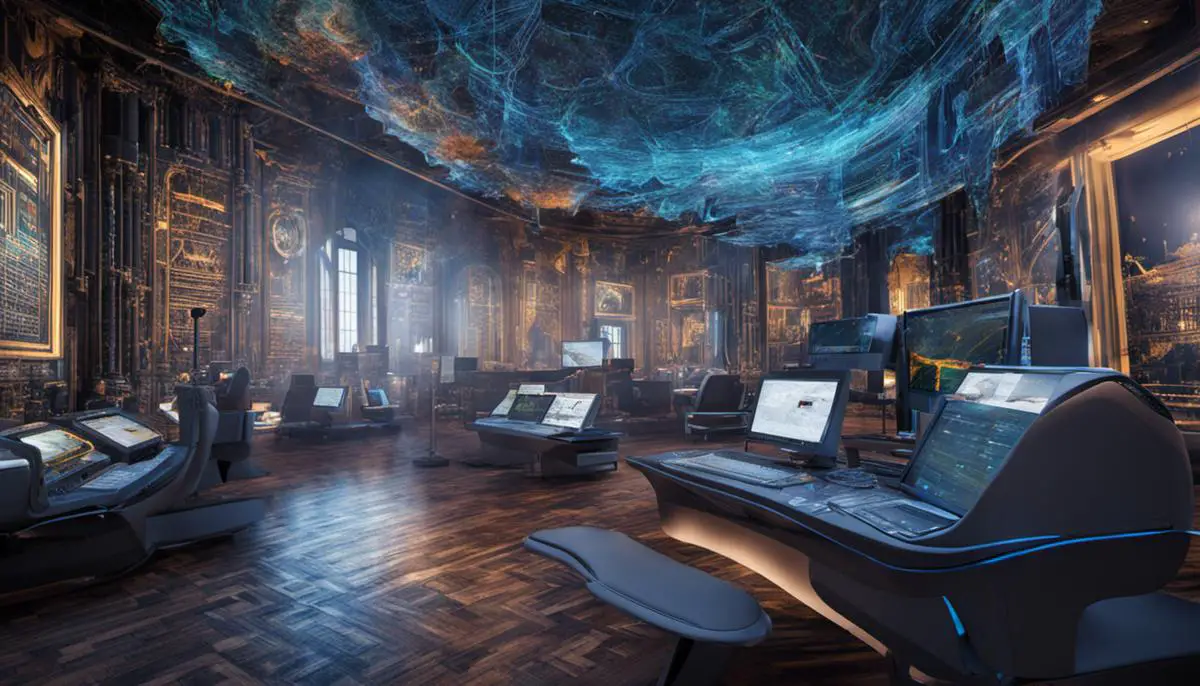
Applied in the realm of 3D image processing, stable diffusion is not only driving innovation but also uncovering a wealth of uncharted possibilities. While the journey from theory to practical application might be strewn with complexities, the promise of efficiency enhancement and transformation in our interaction with digital mediums is a compelling incentive.
The inherent challenges only shed light on areas that require more focused research and development. As emerging trends continue to take shape, be it in healthcare, entertainment, or beyond, the integration of stable diffusion offers exciting prospects that are worth watching for.
Therefore, a thorough exploration of stable diffusion and 3D image processing offers invaluable insights for innovators, technologists, or anyone driven by the passion to understand this field.
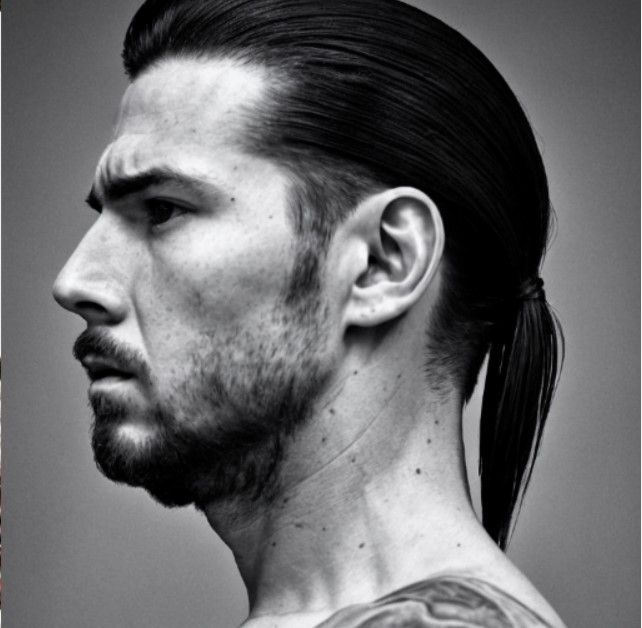
Emad Morpheus is a tech enthusiast with a unique flair for AI and art. Backed by a Computer Science background, he dove into the captivating world of AI-driven image generation five years ago. Since then, he has been honing his skills and sharing his insights on AI art creation through his blog posts. Outside his tech-art sphere, Emad enjoys photography, hiking, and piano.