When considering the digital world we inhabit, the significance and magnitude of high-resolution imaging cannot be overstated. It impacts a variety of industries, from entertainment to healthcare, recognizing its importance in enhancing user experiences, providing accurate medical imaging, and creating realistic video graphics. The combination of High-Resolution Image Synthesis and Machine Learning offers mind-boggling potential, yielding superior image quality that conforms closer to reality. This essay delves into this union, offering an insightful understanding of High-Resolution Image Synthesis, an introduction to Machine Learning and its association with image synthesis, the specific techniques that make it possible, the challenges encountered and envisaged future of this rapidly growing field.
Contents
Overview of High-Resolution Image Synthesis
The world abounds in visuals. Every moment, images flood screens and fill pages, providing an arena to communicate, express, and impress like never before. At this juncture, the field of High-Resolution Image Synthesis assumes a paramount role. Understanding its essence and significance to our modern digital world is nothing less than embarking on an exhilarating journey through the realm of virtual representation and expression.
High-Resolution Image Synthesis, in essence, stands at the fore of innovative technology, dealing with the creation of high-quality, comprehensive, and detailed virtual representations based on various inputs. It combines computational power with the essence of creativity, leading to the creation of almost true-to-real-life images. It can breathe life into static inputs, create new images from complex data or modify existing ones, thereby expanding the realms of digital visualization beyond comprehension.
This diagnosis into High-Resolution Image Synthesis would be incomplete without an understanding of Generative Adversarial Networks (GANs). Unleashing the power of AI and Machine Learning, GANs consist of two neural networks – the ‘Generator‘ and ‘Discriminator‘. The Generator suggests visual patterns, while the Discriminator assesses their authenticity. Together, they conceive and refine high-resolution images that are detailed, nuanced, and virtually indistinguishable from real-world images.
Providing more than just a feast for the eyes, the application potential of High-Resolution Image Synthesis is extensive and influential. From creating photorealistic images for entertainment and advertising to strengthening research in areas like organic chemistry and materials science, the impact is unparalleled. In the field of medicine, generating high-resolution images of organs, tissues, or cells can potentially aid in more accurate diagnoses and treatments. Meanwhile, in the arena of virtual reality, higher-resolution image synthesis can ensure an immersive and more convincing experience.
The importance of High-Resolution Image Synthesis in today’s digital world is also accentuated by recent technological advancements. With the rapid development of virtual and augmented reality, the need for detailed, lifelike, and engaging content is paramount. High-Resolution Image Synthesis, armed with its unique ability to bring abstract concepts, inaccessible scenarios, and imaginative visions realistically to life, becomes a cornerstone for future digital development.
To dwell on the role and influence of High-Resolution Image Synthesis is to appreciate the blend of science, technology, creativity, and innovation. As it opens the door to a new era of digital visualization, it’s not merely about viewing the world through a screen, but rather redefining the very premise of what’s real and what’s recreated in the digital sphere. It offers a novel realm of understanding, expressing, and interacting with the world, thereby transforming the essence of communication, expression, research, and, indeed, life as we know it.
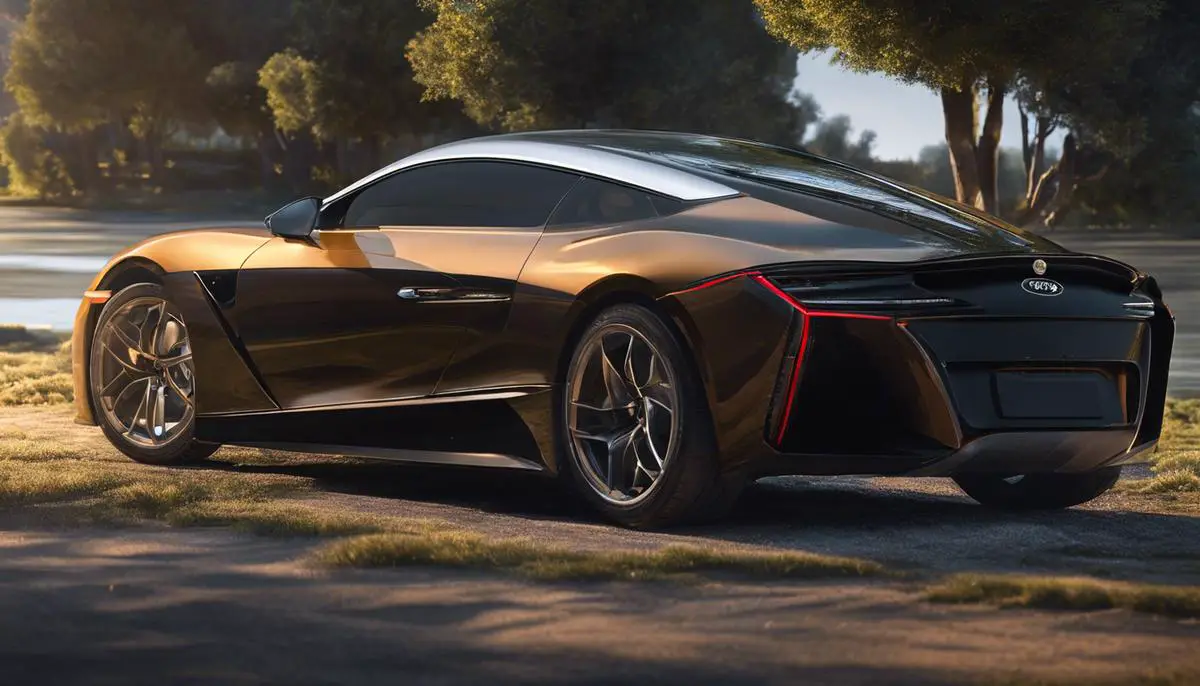
Machine Learning: An Introduction
Unveiling the driving force behind High-Resolution Image Synthesis introduces us to Machine Learning – a branch of Artificial Intelligence (AI), with the capability to analyze, interpret, and understand vast amounts of data in moments. Epitomizing automated decision-making, Machine Learning is the reason why an array of applications, from email spam filters to autonomous vehicles, function with an uncanny intuition that mimics human intelligence.
Machine Learning can be envisaged as an unsupervised student gathering knowledge independently. It laps up data, identifies patterns, then even uses those patterns to make predictions about new data. The extraordinary feat is that it accomplishes this without being explicitly programmed to perform these actions.
Inherent to Machine Learning is its subset – Deep Learning. Akin to a high-powered microscope analyzing microscopic organisms, Deep Learning enables us to see and process more layers of data and detect more patterns. It unpacks the granular compositions of data through artificial neural networks, modeled after the human brain.
Here is where the bond between Machine Learning and High-Resolution Image Synthesis strengthens. Deep Learning inspires a specialized sort of Machine Learning model known as Generative Adversarial Networks (GANs), already discussed in the first part. They form the backbone for replicating or creating High-Resolution images.
In the GAN system, we find two neural networks – the generator and the discriminator, pitching against each other in a captivating game. The generator evolves initial sets of data, essentially faking images, then the discriminator’s mission is to identify whether these images are real or falsified. As this process iterates, the generator curates data so skillfully that it fools even the discriminator, resulting in remarkably convincing, high-resolution imagery.
Machine Learning’s contribution to High-Resolution Image Synthesis transcends pixel-sharp photos or lifelike graphics. In a broader context, Machine Learning provides the capability to personalize the virtual experiences and realize the potential to tailor digital representations for individuals, fulfilling diverse needs and aspirations.
From healing, aiding scientific research, and orchestrating digital advertising campaigns to sketching animated characters or constructing virtual worlds, the possibilities are only limited by imagination. Machine Learning and High-Resolution Image Synthesis not only deliver strikingly rich visualizations but open a gateway to a new era of creativity and innovation.
In our relentless quest for advancement, humans have crafted machines that learn. And it is through this advanced learning, machines are transforming pixels into an intricate mosaic of High-Resolution Image Synthesis, opening the door to endless possibilities.
Machine Learning Techniques in Image Synthesis
Among the key techniques employed in Machine Learning for High-Resolution Image Synthesis, several stand out due to their effectiveness and innovative approach. Techniques such as Perceptual Loss Function, Super-resolution Convolutional Neural Networks (SR-CNN), and Conditional Generative Adversarial Networks (Conditional GANs) have emerged as pioneers in this fascinating domain.
Unlike traditional loss functions, Perceptual Loss Function aims to measure perceptual differences, providing far better High-Resolution Image Synthesis outcomes. It acknowledges not just pixel-to-pixel discrepancies but also the broader features and correlations that exist within an image. It delves deeper, prioritizing character over exact pixel values, thereby contributing to more striking, detailed images.
The Super-resolution Convolutional Neural Network (SR-CNN) technique fits seamlessly into the narrative of Image Synthesis. This technique elevates the essence of High-Resolution Image Synthesis by upgrading lower resolution images into high-resolution versions, aided by the convolutional network architecture. This giant step in the direction of producing finer images is a testament to the power of Machine Learning.
Taking a leap forward, the implementation of Conditional Generative Adversarial Networks (Conditional GANs) has pushed the envelope in High-Resolution Image Synthesis. Conditional GANs are adept at synthesizing high-quality images from the input data by imparting conditional constraints to both generator and discriminator. This careful maneuvering of conditions enables the generation of more explicit, customized images, extending the horizons of Machine Learning in this field.
In the data-scarce realm of High-Resolution Image Synthesis, techniques like Transfer Learning have come to the rescue. By successfully applying learned knowledge from one task to another, it conserves precious resources, while simultaneously improving the end-result. This sharing of intelligence, nature-inspired, signifies the adaptability and versatility of Machine Learning techniques in High-Resolution Image Synthesis.
In conclusion, prosper with an invigorated drive to push boundaries, constantly challenge the status quo, and innovate continuously, Machine Learning’s role in High-Resolution Image Synthesis rungs the gamut from familiar, dependable techniques to groundbreaking, novel applications. The ongoing exploration and enhancements in Machine Learning aim to understand the often overlooked subtleties of images, thereby enabling the crafting of increasingly intricate, engaging, and high-resolution representations.
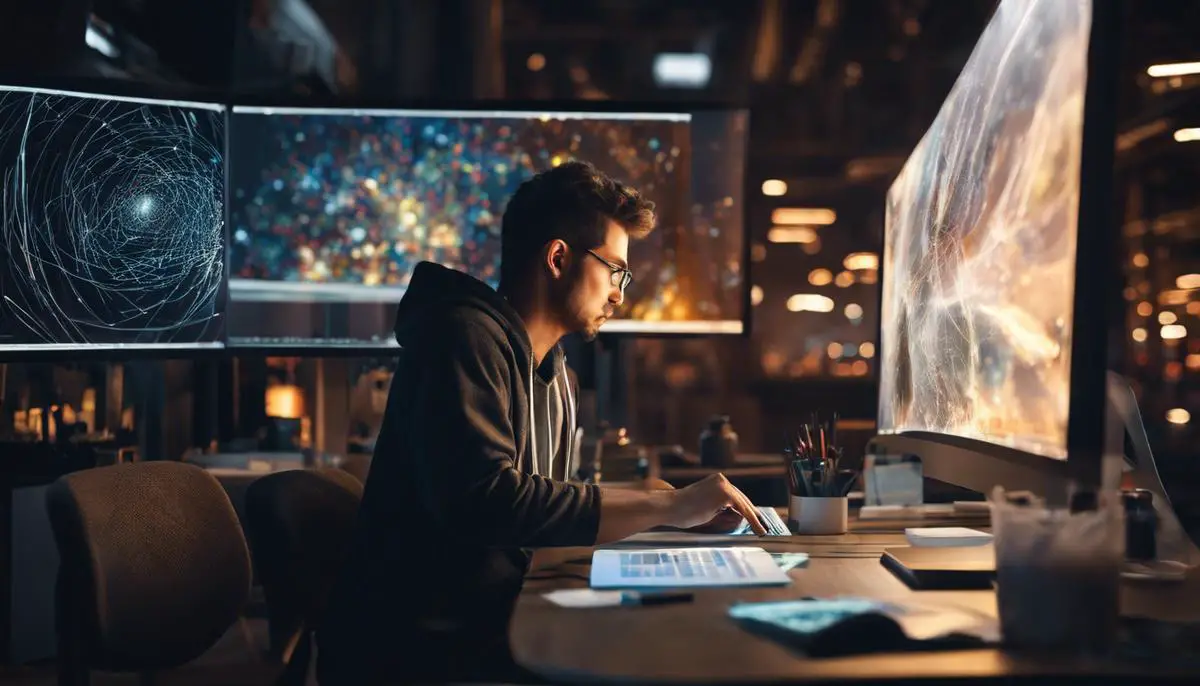
Challenges and Solutions in ML-based Image Synthesis
Influencing the realism and finesse of generated high-resolution images is not without formidable obstacles, and understanding these challenges propels closer to comprehensive solutions. Considerations such as computing requirements, the Limitation Principle, and the capacity to calibrate the fine-grained details can significantly impact the quality and resolution in Machine Learning-driven High-Resolution Image Synthesis.
High-resolution image synthesis demands intensive computational power, forming one of the primary obstacles in its successful integration with machine learning. The training process for GANs and CNNs to produce high-resolution images necessitates state-of-the-art hardware, with an inclination towards Graphical Processing Units (GPUs) over traditional Central Processing Units (CPUs). Although advancements in technology continually push these boundaries, the demand for computational power remains a limiting factor.
A critical aspect of the image generation process is dictated by the Limitation Principle. This principle emphasizes the trade-off encountered when generating a high-resolution image. GANs can produce high-quality images, but due to their design, improving the quality of one aspect may impede the generated image’s overall plausibility. This balance of quality and realism is a significant challenge encountered in high-resolution image synthesis, and poses as a continual complexity in refining GANs’ design and functionality.
Appreciating the intricate detailing within an image implicates yet another challenge. For a machine-learning model to generate a high-resolution image, the model must comprehend and interpret the fine details present in the dataset. In many instances, the model may have difficulties discerning relevant features or characteristics, leading to flawed or unsatisfactory image outputs. The model’s capacity to comprehend these subtle characteristics plays a critical role in the precision and quality of a generated high-resolution image.
In the journey to solve these challenges, numerous techniques emerge to the forefront. Experts engage Transfer Learning, using pre-trained models to streamline computations and expedite learning. Similarly, the application of Super-Resolution Convolutional Neural Networks (SRCNNs) and Conditional GANs increases the accuracy and quality of generated images. With SRCNNs, low-resolution images can be transformed into high-resolution counterparts, while Conditional GANs add a conditional aspect which can refine outputs towards specific, desired results.
Moreover, modifications to the perceptual loss function have shown promise. Rather than purely focusing on pixel-to-pixel loss, the function now considers losses at a feature level. This amendment enhances the image generation quality, thereby tackling the Limitation Principle challenge and allowing for a greater level of detail and resolution in output images.
The exciting fusion of scientific inquiry, computational power, and artistic creativity in the realm of high-resolution image synthesis expands beyond traditional boundaries. As challenges in integrating Machine Learning with Image Synthesis become understood, the potential in entertainment, medicine, virtual reality, and research continues to escalate. This convergence of analytical and creative capabilities heralds untold horizons in the sphere of digital innovation, painting vibrant visions of the future.
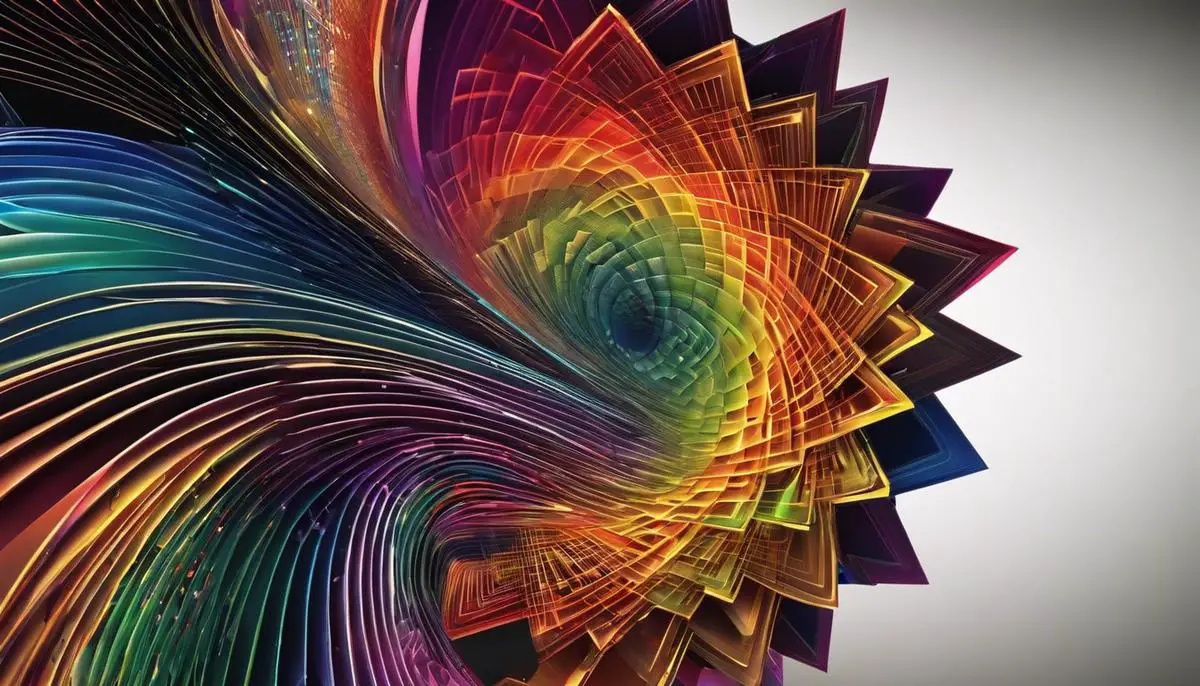
Future of Machine Learning in High-Resolution Image Synthesis
Predicted Future of Machine Learning In High-Resolution Image Synthesis
From an analytical perspective, the future appears ripe with promising advancements in the application of Machine Learning (ML) in high-resolution image synthesis. Facilitating the generation of high-quality images, ML offers the potential to invigorate various sectors, from entertainment and advertising to medicine and virtual reality.
Foremost, an aspirational scenario exists where Machine Learning not only reshapes image synthesis but also the broader landscape of digital media. Bias in Algorithms, regarded as an essential challenge in Machine Learning, could find a solution in advancements of high-resolution image synthesis. By creating more lifelike virtual representations, the realm of possibility expands into eliminating distortions that impedance the depiction of reality in synthesized images.
Another exciting prospect is the development of machine learning models that can further decipher complex data layers. Enhanced deep learning algorithms have the potential to unearth intricate patterns undetectable today, which could result in an unmatched level of precision and refinement in high-resolution images. Imagine the transcendent aesthetic experience offered by digital media art, revealing unseen textures and shades, or the enhanced efficacy of medical imaging, revealing previously undetectable health issues
Moreover, the application of foundational machine learning techniques like Transfer Learning and Conditional Generative Adversarial Networks (Conditional GANs) continues to abound with potential. These established methods form the basis for groundbreaking applications in high-resolution image synthesis, facilitating cross-modal and cross-domain interpretations and transformations.
Yet, the road ahead is not without its challenges. As the caliber of synthesized images becomes increasingly high, so do the computational requirements necessary for image generation. This significant challenge, though formidable, could trigger the development of new technologies and techniques to handle these computing needs sustainably. For instance, novel ways of optimizing Graphical Processing Units (GPUs) and advanced research in managing computing resources could pave the way forward.
In connecting these threads of potential and challenge, one may envision a future where machine learning forms the cornerstone of high-resolution image synthesis, merging analytical power with creative potential. Advancements in pattern detection, model learning, and data interpretation could shape unprecedented levels of detail and authenticity in digital representations. And while we grapple with the computational challenge, the hurdles may spur innovation in technology management and resource allocation.
Postscript, despite the formidable challenges, the future of Machine Learning in high-resolution image synthesis is promising. Its broad range of applications promises a profound impact not only on the digital world and technological advancements but also on our perceptions and experiences of reality. A harmonious blend of computational power, creativity, algorithmic advances, and increased GPU functionality can certainly turn seemingly insurmountable challenges into opportunities. The trajectory of this area of expertise is undoubtedly as exciting as it is formidable to meander and overcome.
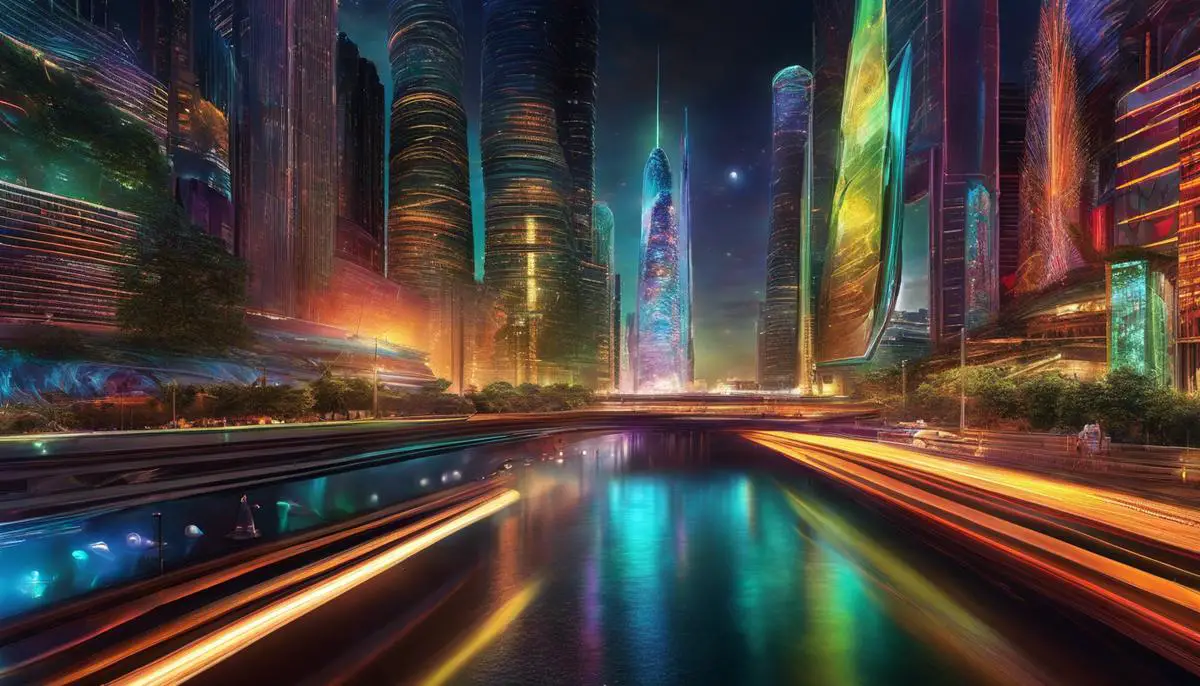
With technology making giant strides, there is no ceiling to the possibilities that High-Resolution Image Synthesis combined with Machine Learning can unleash. The progress seen so far is impressive, and the future looks even more promising, as we move toward designing and developing more advanced machine learning models capable of producing high-resolution images that were previously considered only as figments of wild imagination. Keeping an eye on the emerging trends and continuous research in this field is quite imperative. We stand on the cusp of breakthrough advancements that have the potential to revolutionize numerous industries, hiking up the importance of gaining depth in this topic.
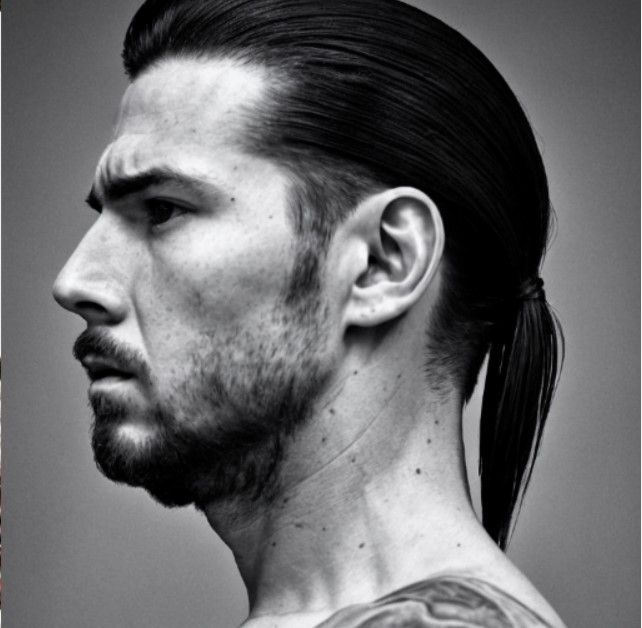
Emad Morpheus is a tech enthusiast with a unique flair for AI and art. Backed by a Computer Science background, he dove into the captivating world of AI-driven image generation five years ago. Since then, he has been honing his skills and sharing his insights on AI art creation through his blog posts. Outside his tech-art sphere, Emad enjoys photography, hiking, and piano.