The visual quality of digital content holds a significant weight in the realms of media consumption, professional graphics work, and the enhancement of personal multimedia. Real-ESRGAN emerges as a vanguard technology in this space, offering remarkable capabilities in image upscaling through the power of advanced artificial intelligence. At its core, Real-ESRGAN is not merely a tool for increasing pixel counts but a sophisticated method for breathing new life into visuals that are bound by their low resolution. By delving into the mechanics of Real-ESRGAN, we uncover the synergy between groundbreaking deep learning algorithms and the ever-expanding demand for visual excellence across various industries. This exploration sets the stage for a comprehensive understanding of an innovation that is swiftly redefining the landscape of image processing and quality enhancement.
Contents
- 1 Understanding Real-ESRGAN
- 2 Technological Foundations of Real-ESRGAN
- 3 Real-ESRGAN vs. Traditional Upscaling Methods
- 4 Practical Applications of Real-ESRGAN
- 5 Implementing Real-ESRGAN
- 5.1 Getting Started with Real-ESRGAN: A Guide for Developers and Enthusiasts
- 5.2 Installation and Setup
- 5.3 Real-ESRGAN’s GitHub Repository
- 5.4 Understanding Real-ESRGAN’s Command Line Interface
- 5.5 Experimenting with Pre-trained Models
- 5.6 Creating Custom Datasets
- 5.7 Training Real-ESRGAN
- 5.8 Exploring Upscaling Projects
- 5.9 Continuous Learning and Collaboration
- 6 The Future of Real-ESRGAN and Image Upscaling
Understanding Real-ESRGAN
Real-ESRGAN: Revolutionizing Image Upscaling with AI
In the digital age, image clarity is crucial. From gamers to graphic designers, everyone demands high-resolution visuals. Enter Real-ESRGAN, the cutting-edge AI-powered technology that’s redefining image upscaling. This innovative tool doesn’t just size up images; it transforms them.
Traditionally, upscaling makes images bigger but often at the cost of quality, leaving them blurry or pixelated. Real-ESRGAN changes that game. It uses Enhanced Super-Resolution Generative Adversarial Networks (ESRGAN), a fancy term for AI that learns from data to produce incredibly sharp and detailed upscaled images.
Here’s how it works: Real-ESRGAN takes low-resolution images and runs them through a neural network—think of it as a brainy computer program. This network has been trained on a mountain of image data, learning how to predict and fill in missing details with remarkable accuracy. The output? Images that are upscaled with enhanced textures and clarity that traditional methods can’t match.
Why is this exciting? For starters, Real-ESRGAN can breathe new life into old photos, game textures, and videos without the manual hassle. Photographers can enhance their shots without a reshoot. Game developers can upgrade graphics without redrawing every frame. Everyone gets to enjoy cleaner, crisper images.
Real-ESRGAN isn’t just about pretty pictures; it’s also super accessible. With just a few clicks, users can upscale images right from their computer. No advanced graphics skills required.
In summary, Real-ESRGAN is AI-powered magic for images, providing a no-brainer solution to the age-old problem of how to make pictures look fantastic even at large sizes. It’s smarter, it’s faster, and it sets a new standard for image upscaling. No need to settle for less—Real-ESRGAN is here to push visual boundaries.
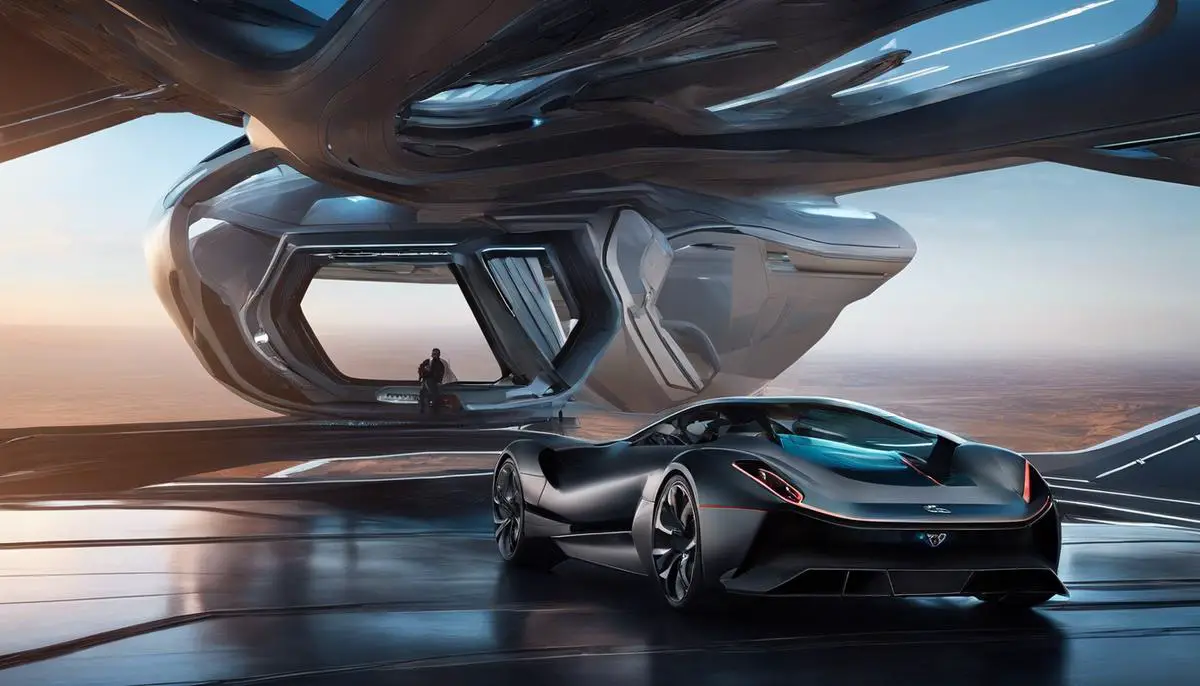
Technological Foundations of Real-ESRGAN
Diving deeper into what operates under the hood of Real-ESRGAN, it’s crucial to grasp the advanced AI and machine learning principles that endow this technology with its prowess.
Real-ESRGAN is built upon a framework that utilizes a newer, more potent iteration of Generative Adversarial Networks, known as ESRGAN. These networks consist of two main components, the generator and discriminator, each of which serves a critical function in the image upscaling process.
At its core, the generator’s role is to produce high-resolution images from low-resolution inputs. It’s not simply stretching the pixels; it’s intelligently filling in detail where none existed. Here’s where machine learning flexes its muscles. The generator is a convolutional neural network (CNN), a kind of network adept at handling image data. This CNN is composed of many layers, where each layer focuses on learning different aspects of the image – some might recognize edges, while others interpret textures or patterns.
Meanwhile, the discriminator is the quality checker. It compares the generated images against a set of high-resolution reference images. It’s a ruthless critic, trained to differentiate between the artificial and the real deal. Whenever the discriminator detects an image that doesn’t make the grade, it sends feedback to the generator. This is key as it keeps the system honest and ever-improving.
A principle known as perceptual loss comes into play here. Instead of just looking at whether each pixel is in the right place, perceptual loss encourages the system to ensure the overall structure, context, and texture of the image matches up closely with that of high-quality images. This means images aren’t just upscaled; they are reconstructed with a focus on high fidelity and realism.
Real-ESRGAN also employs self-attention mechanisms, a concept borrowed from transformer models, which are a big deal in language processing AI. These mechanisms enable the model to focus on important features in an image, no matter where they are located. Think of it like having a sharper eye for detail, spotting nuances and intricacies that make all the difference between a bland photo and a striking image full of life.
Finally, since the world never stops taking pictures or generating images, Real-ESRGAN can’t be static either. Thankfully, this tool is founded on the principle of continuous learning. It’s trained on a diverse dataset that’s regularly updated, allowing it to not only improve over time but also to adapt to new types of images and artifacts. This ensures that Real-ESRGAN stays relevant and efficient, regardless of the evolving digital landscape.
Real-ESRGAN isn’t just upscaling; it’s a sophisticated interplay of advanced machine learning techniques that push the boundaries of what AI can achieve with imagery. It’s accessible to everyone and preemptively changes the game for photographers, gamers, designers, and technophiles eager to bring past graphics into the crystal-clear focus of today’s digital standards.
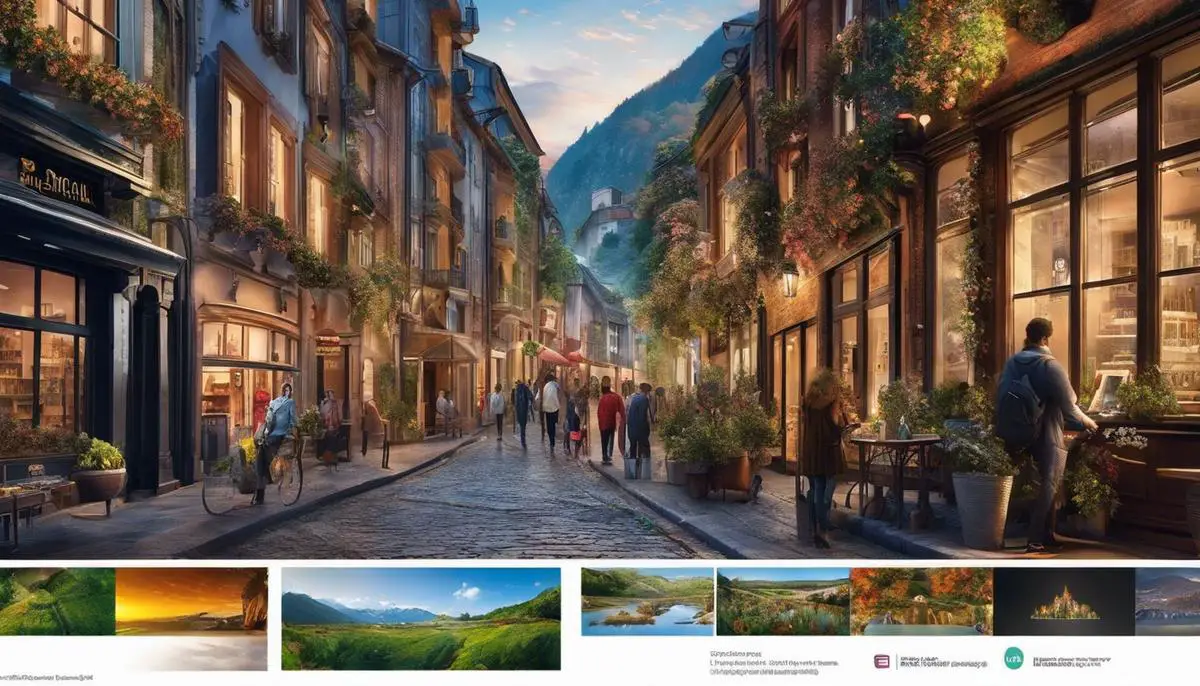
Real-ESRGAN vs. Traditional Upscaling Methods
Real-ESRGAN: The Future of Crisp, Enhanced Visuals
In the realm of image upscaling, Real-ESRGAN stands tall, but what exactly makes it outshine its classical counterparts? The answer lies in its adept handling of texture, detail, and fidelity.
Where traditional upscaling techniques falter—often producing blurred edges and visible artifacts—Real-ESRGAN excels. Its AI-driven engine interprets and reconstructs images, simultaneously addressing both macro and micro aspects. This ensures that details, which would typically be washed out, are now brought to life with exceptional clarity.
The gritty details of texture reproduction are where Real-ESRGAN flexes its superiority. In environments like gaming, where textures can make or break the immersive experience, Real-ESRGAN provides a breath of fresh pixels. The AI doesn’t just scale; it understands the texture and smartly enhances it to suit the high-resolution output.
Edge restoration is one of the most notable game-changers with Real-ESRGAN. Neglecting edges often leads to images that look like they’ve been sawed off and slapped together haphazardly. Real-ESRGAN, on the other hand, treats edges with the finesse they deserve, translating to images that are true to life.
Another victory for Real-ESRGAN is in the reduction of visual noise. Where regular upscaling might inadvertently amplify unwanted noise, Real-ESRGAN sidesteps this entirely. Its smart algorithms precisely distinguish between noise and detail, a critical discernment that preserves the integrity of the original image while elevating its quality.
Versatility is central to Real-ESRGAN’s design. It isn’t tethered to just one type of content or media. Whether it’s historical photographs or the latest anime production, Real-ESRGAN adapts seamlessly, proving its robustness and flexibility across various applications.
Finally, Real-ESRGAN isn’t a static tool; it’s dynamic. It improves continuously as it encounters more data and scenarios. Unlike classical upscaling methods that stagnate over time, Real-ESRGAN is a learning entity, consistently evolving to deliver better results.
In conclusion, Real-ESRGAN isn’t just about making images larger; it’s about making them better. With attention to detail, texture fidelity, noise reduction, and adaptive learning, it transcends classical upscaling boundaries. The journey of an image from pixelation to perfection is complex, but with Real-ESRGAN, it’s a path well illuminated.
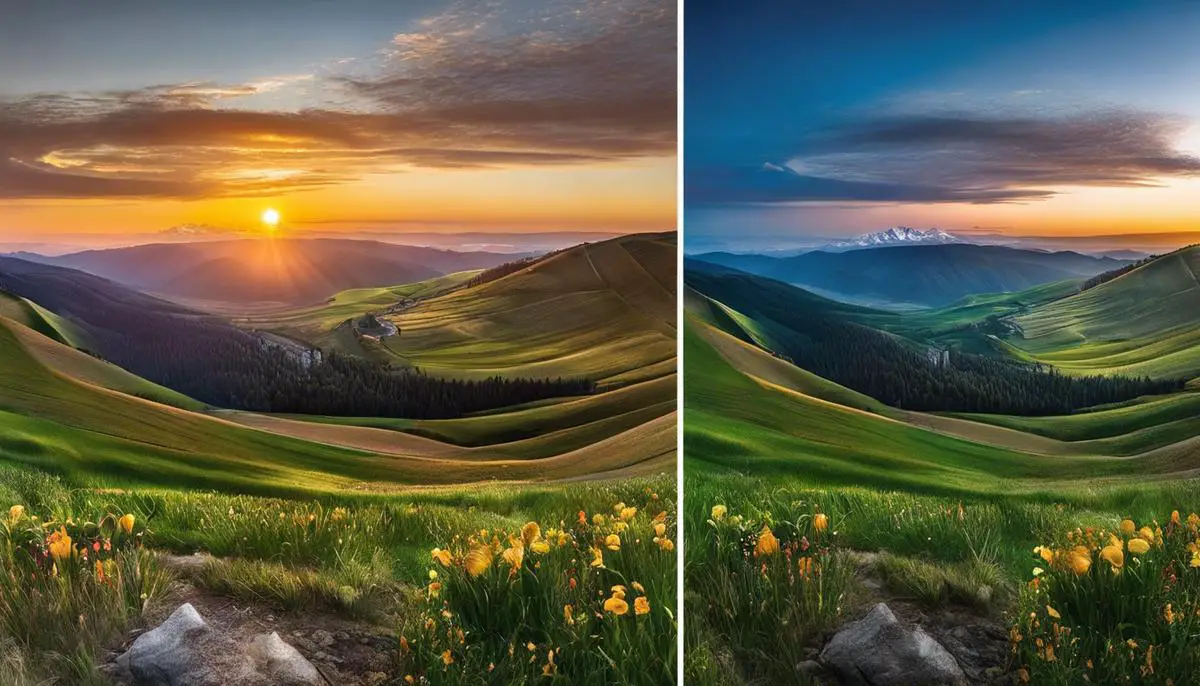
Practical Applications of Real-ESRGAN
Real-ESRGAN: Revolutionizing Real-World Visuals One Pixel at a Time
Visual clarity isn’t a luxury anymore; it’s a necessity. In a world where details can make or break an experience, Real-ESRGAN isn’t just another piece of tech—it’s a game changer. The real-world applications of this powerful tool are excitingly diverse and go well beyond touching up a vintage family photo or giving your favorite video game a graphics boost.
Ever browsed an online store, only to be turned off by the low-res product images? That’s where Real-ESRGAN swoops in, transforming e-commerce platforms to virtual showrooms with crystal-clear imagery. Retailers can now automatically upscale their inventory visuals without costly photo shoots for every item. The result? Enhanced customer confidence and reduced return rates driven by a clear, accurate understanding of products.
In the realm of real estate and architecture, what used to involve labor-intensive artwork now sees a speedy makeover. Property listings, oftentimes featuring outdated or lackluster photos, are being digitally remastered to bring out the true charm of homes, making virtual tours almost as good as walking through the door. Architecture firms employ Real-ESRGAN to convert old blueprints into detailed, high-res digital files, streamlining design processes and historical restoration projects.
Let’s not ignore the world of digital libraries, museums, and archives. Historians and archival technicians use Real-ESRGAN to breathe new life into aged documents, maps, and artworks, preserving and sharing our collective heritage with stunning clarity. From academia to ancestry research, the implications are vast—unlocking the past through pixels preserved in high definition.
When it comes to law enforcement and surveillance, clarity can mean the difference between solving a case and a dead end. Improved facial recognition and license plate reading through enhanced frame quality is a boon for security and justice. Real-ESRGAN plays a crucial role in these areas, making it possible to extract actionable information from previously unusable footage.
In media and entertainment, production crews are upscaling archived footage to meet today’s HD standards, offering audiences a refreshed look at classic shows and movies without rerunning the entire filming process. The technology also assists in VFX work, enabling artists to create intricate effects with fewer resources, thus pushing the envelope of cinematic imagination while keeping budgets in check.
Lastly, the scientific and medical fields are not left out. From detailed views of satellite imagery for climate research to improved diagnostic quality of medical scans, the applications are profound. Real-ESRGAN is enabling better data analysis, fostering new discoveries, and enhancing patient outcomes.
As tech enthusiasts, seeing Real-ESRGAN tackle real-world problems and enhance various industries with its prowess is nothing short of thrilling. It’s not just about having sharper images; it’s about unlocking potential, enhancing experiences, and, in many cases, improving quality of life. That’s the power of advanced AI and machine learning—Real-ESRGAN isn’t just working harder; it’s working smarter, continuously learning, and recalibrating for an increasingly detailed world.
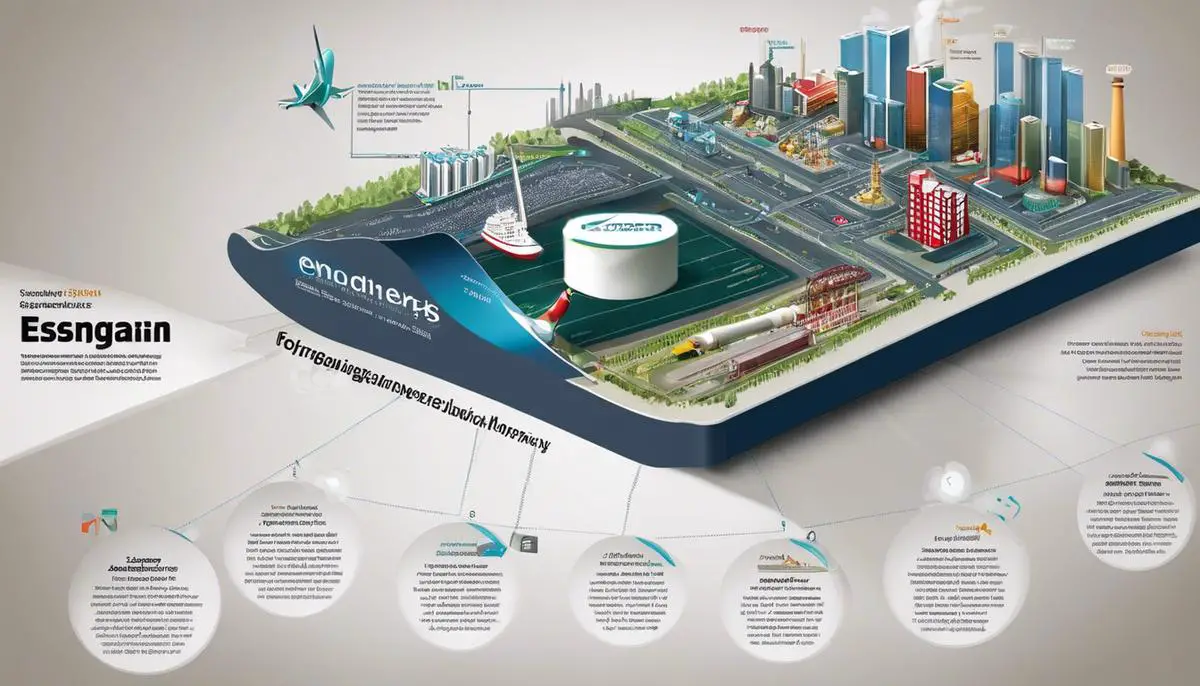
Implementing Real-ESRGAN
Getting Started with Real-ESRGAN: A Guide for Developers and Enthusiasts
Diving into Real-ESRGAN requires a hands-on and problem-solving mindset. Here’s a roadmap for developers and tech enthusiasts eager to harness this powerful image upscaling tool:
Installation and Setup
First things first, grab the pre-requisites. These are Python 3, PyTorch, and some additional dependencies like ‘cv2’ and ‘numpy.’ Installation guides for PyTorch are plentiful online, and the Real-ESRGAN GitHub repository has comprehensive instructions on setting up the framework.
Real-ESRGAN’s GitHub Repository
Start by cloning the official Real-ESRGAN repository. It’s where active development happens, and it’s a gold mine of updated code, pre-trained models, and example commands. Keep it bookmarked, as it’s going to be a frequent go-to.
Understanding Real-ESRGAN’s Command Line Interface
Command-line aficionados, rejoice! Real-ESRGAN thrives on command-line inputs. Tinker around with the example commands and observe the output. Change parameters like the upsampling factor or noise reduction level and see the magic happen.
Experimenting with Pre-trained Models
Jump into upscaling right away by downloading pre-trained models provided in the GitHub repository. Test them on a variety of images to get a feel for what Real-ESRGAN can do. Pay attention to how these models tackle different textures and patterns.
Creating Custom Datasets
For those itching to train new models, creating a diverse and high-quality dataset is key. Collect images that represent the sort of quality and variety you’re aiming for. The more comprehensive the dataset, the more capable the model becomes.
Training Real-ESRGAN
Now, brace for the most intense part. Training a model is resource-intensive but entirely worth the effort. Utilize a high-power GPU if possible, and watch as Real-ESRGAN learns from the dataset. Monitor the training process and adjust the hyperparameters to perfect the model.
Exploring Upscaling Projects
Start unleashing Real-ESRGAN’s potential. Work on practical projects like revamping a vintage family photo album or optimizing visuals for a personal website. Share progress on tech forums, and don’t be afraid to ask for community input.
Continuous Learning and Collaboration
Keep the learning wheels spinning. Follow advancements in ESRGAN technology and partake in collaborative projects. Open-source communities appreciate contributions, and Real-ESRGAN’s evolution thrives through collective intelligence.
Real-ESRGAN is only the beginning of an upscaling revolution that blends creativity with technical prowess. Join forces with this cutting-edge technology, and get ready to redefine the boundaries of image quality enhancement.
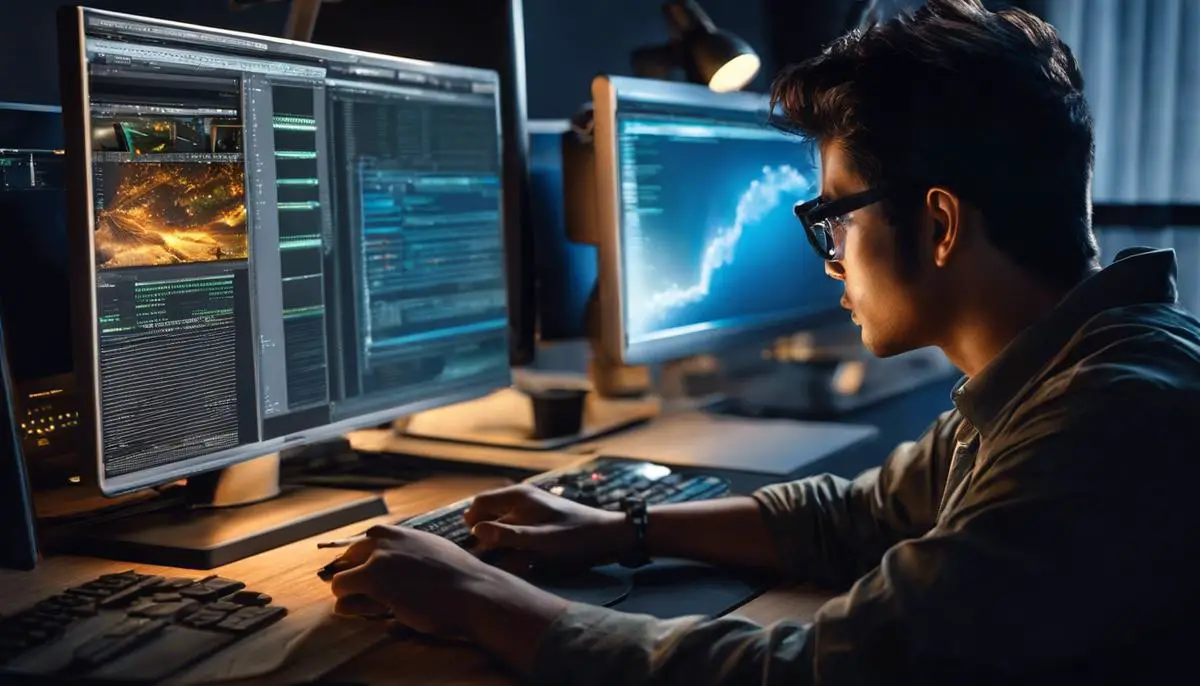
The Future of Real-ESRGAN and Image Upscaling
The future is undeniably bright for Real-ESRGAN and the broader field of image upscaling. With its robust AI framework and continuous learning capabilities, Real-ESRGAN is poised to lead the charge in transforming how we enhance digital images.
One exciting prospect is the incorporation of Real-ESRGAN into real-time applications. Imagine streaming services dynamically upscaling content to match the viewer’s screen resolution without pre-processing videos – this could fundamentally change the way we experience media.
Another development on the horizon is the integration of Real-ESRGAN with mobile devices. As smartphone cameras become increasingly powerful, the demand for on-device image enhancement will soar. Real-ESRGAN’s technology could be optimized for mobile chipsets, allowing users to capture and immediately enhance photos with unprecedented detail.
The realm of 3D visualization also stands to benefit greatly. With augmented and virtual reality (AR/VR) gaining traction, the need for high-resolution textures is paramount. Real-ESRGAN will likely contribute to more immersive and realistic AR/VR environments by improving texture details in real-time, setting a new standard for virtual experiences.
In e-commerce, the current applications of Real-ESRGAN are just scratching the surface. Future iterations could offer solutions tailored for live commerce platforms, enhancing product visuals in real time as hosts showcase them on streams, thereby driving engagement and sales.
Further advancements may see Real-ESRGAN becoming more user-friendly and accessible to non-technical users. Although its roots are in complex AI models, future versions could feature intuitive graphical user interfaces, allowing anyone from graphic designers to hobbyist photographers to upscale images with a few clicks.
On the academic front, Real-ESRGAN may well aid in research across various disciplines. Machine learning algorithms could be trained on enhanced images to extract data with greater accuracy, potentially unlocking new insights in fields like astrophysics and biology.
Last but not least, as the community around Real-ESRGAN grows, so does the variety of creative and unexpected applications. From restoring vintage video games to generating high-resolution art, the open-source nature of Real-ESRGAN invites a future shaped by collaboration and innovation.
In summary, as technology marches forward, the applications and capabilities of Real-ESRGAN are set to expand. The technology is already redefining the limits of what can be achieved with image upscaling, and there’s no telling what the next breakthrough may be. What is certain is that Real-ESRGAN and similar technologies will continue to evolve, driven by a community that thrives on pushing boundaries and enhancing the visual elements of our digital world.
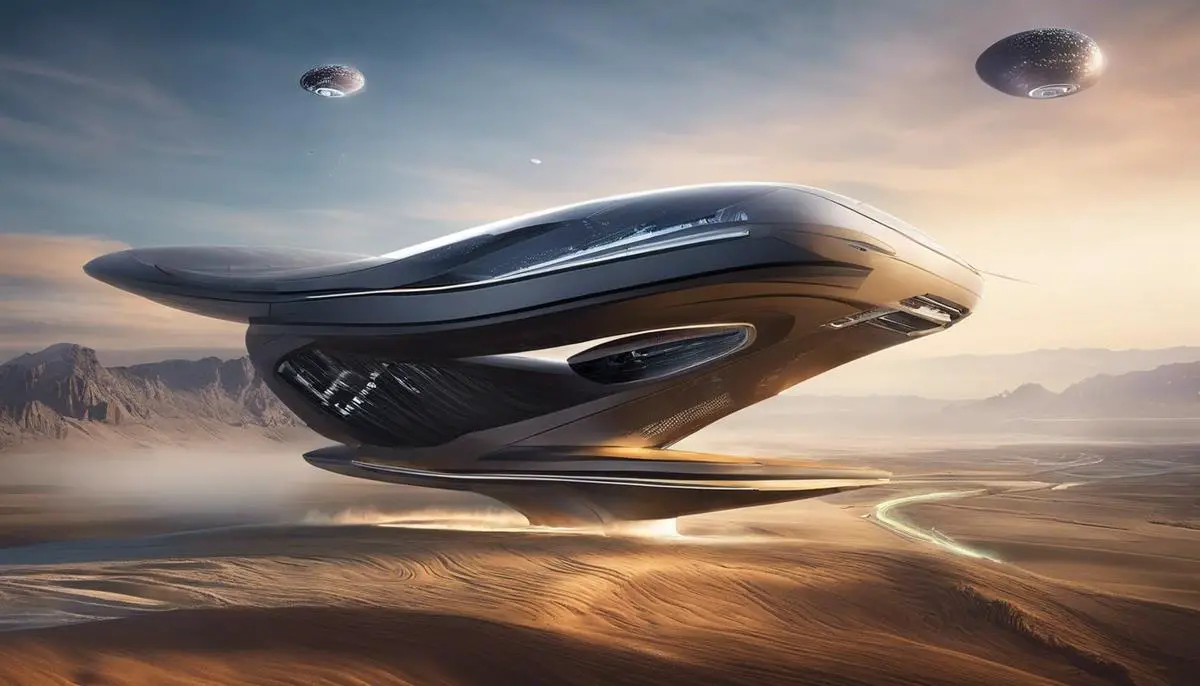
As we stand on the cusp of a new era in digital image processing, Real-ESRGAN presents itself as both a herald and a testament to the power of intelligent technology in transforming the visual experiences of tomorrow. The integration of such advanced upscaling techniques in various sectors promises not only to revolutionize how we interact with digital content but also to raise the bar for visual quality and accessibility. With the anticipated advancements and the increasing adaptability of Real-ESRGAN alongside emergent tech, the potential for enriched multimedia environments is boundless. The journey through the realms of Real-ESRGAN not only encourages us to reimagine the possibilities within image rendering but also to participate in a future where the lines between the virtual and the real continue to blur with stunning clarity and detail.
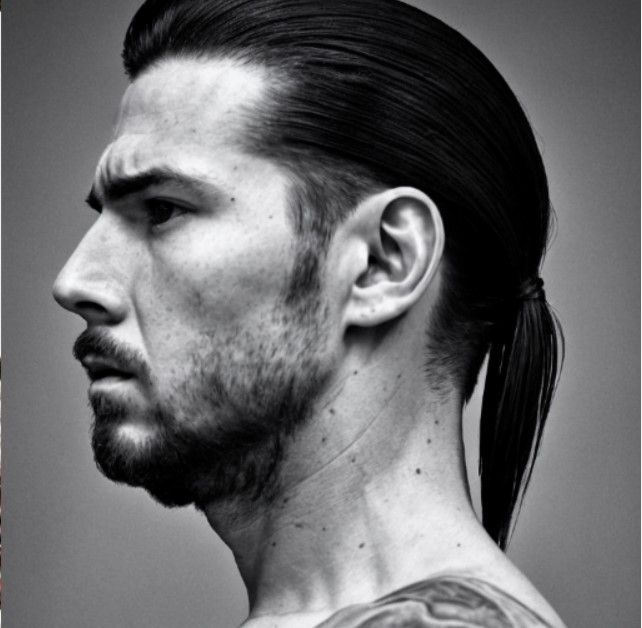
Emad Morpheus is a tech enthusiast with a unique flair for AI and art. Backed by a Computer Science background, he dove into the captivating world of AI-driven image generation five years ago. Since then, he has been honing his skills and sharing his insights on AI art creation through his blog posts. Outside his tech-art sphere, Emad enjoys photography, hiking, and piano.