Stable Diffusion Research has emerged as a groundbreaking field at the forefront of AI Imagery, revolutionizing the way we approach high-resolution image synthesis. The exploration and development of Stable Diffusion techniques have opened new horizons in the generation of visually stunning and realistic images.
By decomposing the image formation process into sequential applications of denoising autoencoders, Stable Diffusion Research has provided unprecedented capabilities in suppressing semantically meaningless information and enhancing overall visual fidelity. In this article, we delve into the exciting realm of Stable Diffusion Research, exploring its principles, advancements, and the transformative impact it has had on the field of AI Imagery.
Contents
- 1 High-Resolution Image Synthesis: The Power of Latent Diffusion Models (LDM) for AI Imagery
- 2 Power of Sequential Application: Denoising Autoencoders
- 3 Performance and Efficiency: LDMs as Game-Changers
- 4 Stable Diffusion Research: Perceptual and Semantic Compression with Latent Diffusion Models (LDMs) for Efficient Image Generation
- 5 Stable Diffusion Research: Perceptual and Semantic Compression with Latent Diffusion Models (LDMs) for Efficient Image Generation
- 6 Image Samples from LDMs Trained on Diverse Datasets: The Power of Latent Diffusion Models
- 7 Inference Speed vs Sample Quality: Analyzing LDMs with Varying Compression Levels on CelebA-HQ and ImageNet Datasets
- 8 Latent Diffusion Models (LDMs) in Action: Layout-to-Image Synthesis and Text-to-Image Generation
- 9 Generalization of Stable Diffusion Latent Diffusion Models (LDMs) for Spatially Conditioned Tasks: High-Quality Semantic Synthesis of Landscape Images
- 10 Super-Resolution from ImageNet 64 to 256: Comparing LDM-SR and SR3 on ImageNet-Val
- 11 Qualitative Results: Image Inpainting Evaluation
- 12 Qualitative Results: Object Removal with our Big, w/ FT Inpainting Model
- 13 Convolutional Samples from the Semantic Landscapes Model: Fine-tuned on 512×512 Images (Section 4.3.2)
- 14 Reestablishing Coherent Global Structures in Landscape Generation: Leveraging L2-Guiding with Low-Resolution Images
- 15 Effect of Latent Space Rescaling on Convolutional Sampling for Semantic Image Synthesis on Landscapes
- 16 Training Progress of Class-Conditional LDMs on ImageNet Dataset for Stable Diffusion Research
- 17 Generalization of LDM-BSR for Upsampling Arbitrary Inputs
- 18 Qualitative Superresolution Comparison: LDM-SR vs. Baseline Diffusion Model in Pixel Space
- 19 Convolutional Samples from the Semantic Landscapes Model: Fine-tuned on 512×512 Images
- 20 Generalization of LDM for Spatially Conditioned Tasks: Semantic Synthesis of Landscape Images
- 21 Leveraging Semantic Maps for Generalization to Larger Resolutions: Expanding the Capabilities of LDMs
- 22 Random Samples from LDM-8-G on the ImageNet Dataset: Exploring High-Quality Outputs
- 23 Exploring Random Samples from LDM-8-G on the ImageNet Dataset: Unveiling High-Quality Outputs
- 24 Captivating Random Samples from LDM-8-G: Exploring ImageNet’s Diversity
- 25 Captivating Random Samples from LDM-4: Exploring CelebA-HQ’s Beauty
- 26 Exquisite Random Samples from LDM-4: Discovering the Beauty of FFHQ Dataset
- 27 Breathtaking Random Samples from LDM-8: Unveiling LSUN-Churches’ Grandeur
- 28 Exquisite Random Samples from LDM-4: Unveiling LSUN-Bedrooms’ Splendor
- 29 Exploring Nearest Neighbors: Finding the Similarities in CelebA-HQ Images
- 30 Preliminary Conclusions of Stable Diffusion Research
- 31 Frequently Asked Questions about Stable Diffusion Research
- 31.1 Q1: What is Stable Diffusion?
- 31.2 Q2: What are Latent Diffusion Models (LDMs)?
- 31.3 Q3: How do LDMs overcome computational barriers?
- 31.4 Q4: What are the advantages of LDMs compared to traditional methods?
- 31.5 Q5: Can LDMs be used for spatially conditioned tasks?
- 31.6 Q6: What role does Stable Diffusion play in LDMs?
- 31.7 Q7: How do LDMs generalize to larger resolutions?
- 31.8 Q8: What datasets have LDMs been trained on?
- 31.9 Q9: How can LDMs be applied in different industries?
- 31.10 Q10: What does the future hold for Stable Diffusion and LDMs?
- 31.11 Q11: How does Stable Diffusion improve image synthesis compared to traditional methods?
- 31.12 Q12: Can LDMs be fine-tuned on specific datasets?
- 31.13 Q13: How can LDMs be evaluated quantitatively?
- 31.14 Q14: Are LDMs applicable to other domains besides image synthesis?
- 31.15 Q15: How can one get started with using LDMs?
- 31.16 Q16: What are some potential future directions for research in Stable Diffusion and LDMs?
- 31.17 Q17: Can LDMs be combined with other generative models or techniques?
- 31.18 Q18: How do LDMs compare to other state-of-the-art generative models?
- 31.19 Q19: Are there any open challenges or limitations in the field of Stable Diffusion and LDMs?
- 31.20 Q20: How can the advancements in Stable Diffusion and LDMs benefit real-world applications?
High-Resolution Image Synthesis: The Power of Latent Diffusion Models (LDM) for AI Imagery
In the captivating world of AI Imagery, Stable Diffusion research is creating a groundbreaking technique called Latent Diffusion Models (LDMs) that has taken centre stage, revolutionizing high-resolution image synthesis.
Today, we dive into the fascinating realm of LDMs, exploring their unparalleled flexibility and efficiency, and how they have transformed the way professionals in the field approach image generation and modification.
Credit for the research paper goes to the following authors: Robin Rombach, Andreas Blattmann, Dominik Lorenz, Patrick Esser, and Björn Ommer. The research was conducted at LMU Munich, IWR at Heidelberg University, and Runway. This paper was presented at CVPR 2022 as an oral presentation, showcasing the significant contributions of the authors in advancing the field of AI Imagery.
Power of Sequential Application: Denoising Autoencoders
Imagine unravelling the secrets of image formation, pixel by pixel, to create stunning visuals. LDMs achieve precisely that by leveraging the sequential application of denoising autoencoders. This dynamic approach allows for superior image quality that surpasses traditional methods.
With LDMs at the forefront, AI Imagery enthusiasts and professionals can unlock the true potential of synthesis, leaving behind pixelated limitations.
Image Modification without Retraining: The Beauty of LDMs
One of the most fascinating aspects of LDMs is their ability to modify images directly, without the need for retraining. The magic lies in the Stable Diffusion technique employed by LDMs, enabling efficient tasks like inpainting.
The simplicity and convenience of LDMs give creative minds the freedom to shape images effortlessly, opening up a realm of possibilities in the world of AI Imagery.
Overcoming Computational Barriers: LDMs to the Rescue
Powerful image synthesis often requires extensive computational resources. However, LDMs offer a solution by training these models in the latent space of pretrained autoencoders.
By doing so, LDMs retain exceptional quality and flexibility while significantly reducing computational requirements. Finally, professionals can breathe a sigh of relief as LDMs make high-quality synthesis accessible even with limited resources.
Balancing Complexity and Fidelity: The Art of Spatial Downsampling
Finding the sweet spot between complexity reduction and spatial downsampling is an art, and LDMs have mastered it. By training diffusion models, specifically Stable Diffusion models, in the latent space, LDMs achieve a remarkable balance.
The result? Enhanced visual fidelity that breathes life into high-resolution images. With LDMs, AI Imagery enthusiasts can witness the magic of synthesis at its finest.
Taking DMs to New Heights: Cross-Attention Layers
As if LDMs weren’t impressive enough, cross-attention layers step onto the scene to redefine their capabilities. Picture this: LDMs, driven by Stable Diffusion principles, transformed into powerful generators that embrace a wide array of conditioning inputs. From text to bounding boxes, LDMs can seamlessly incorporate diverse elements into the synthesis process. This revolutionary addition empowers professionals to embark on high-resolution synthesis adventures, all in a convolutional manner.
Performance and Efficiency: LDMs as Game-Changers
Latent Diffusion Models (LDMs), built upon the foundation of Stable Diffusion techniques, showcase extraordinary performance across various tasks. Unconditional image generation, inpainting, and super-resolution are no longer mere dreams but achievable realities with LDMs.
What’s more, LDMs significantly reduce the computational burden compared to their pixel-based counterparts. This remarkable boost in efficiency makes LDMs an indispensable tool for tech-savvy professionals and avid AI Imagery enthusiasts.
LDMs Redefining AI Imagery
As we conclude this exploration of Latent Diffusion Models (LDMs), we stand in awe of the transformative power they hold. With their sequential application, direct image modification capabilities, and breakthrough solutions for computational limitations, LDMs have elevated the realm of high-resolution image synthesis.
Through the lens of cross-attention layers and remarkable performance, LDMs are redefining the boundaries of AI Imagery. Embrace the revolution and let LDMs unleash your creative potential like never before.
The English translation is available here.
The image below demonstrates how we can achieve higher-quality images by using less aggressive downsampling. Unlike other generative models, diffusion models have built-in abilities to understand spatial data. This means that we don’t need to heavily reduce the image’s details when working with diffusion models.
Instead, we can use appropriate techniques to encode the image data in a more compact form. The images shown in Figure 1 are from the DIV2K dataset, specifically the validation set, and they were evaluated at a resolution of 512×512 pixels.
The “spatial downsampling factor” (represented as “f”) indicates the amount of downsampling applied. The quality of the reconstructed images is measured using metrics like the FID (Fréchet Inception Distance) and PSNR (Peak Signal-to-Noise Ratio), which help evaluate how closely the reconstructed images resemble the original ones.
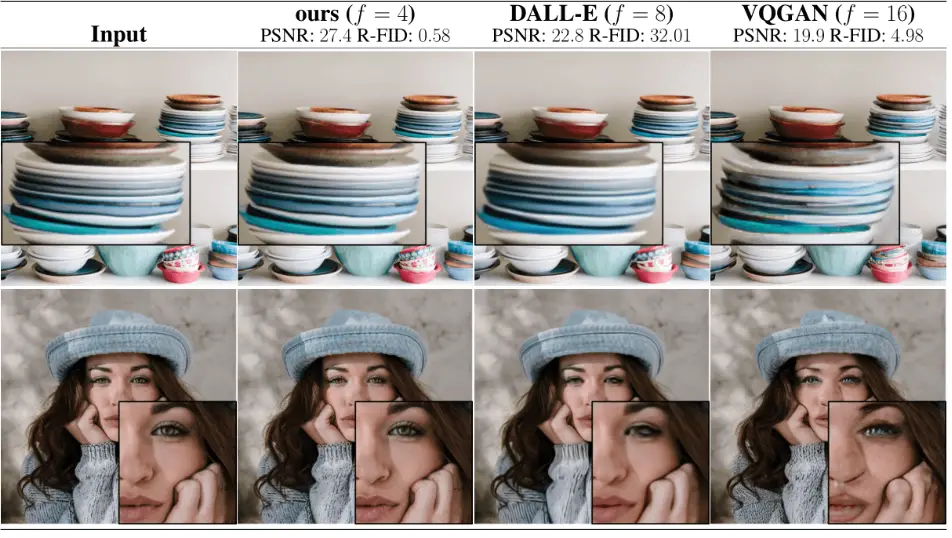
Stable Diffusion Research: Perceptual and Semantic Compression with Latent Diffusion Models (LDMs) for Efficient Image Generation
Perceptual and semantic compression plays a crucial role in digital image processing. It is essential to understand that a large portion of the digital image consists of imperceptible details that do not significantly contribute to the overall visual quality.
While diffusion models (DMs) excel at suppressing this semantically meaningless information by minimizing the associated loss term, there are still computational challenges involved. During both training and inference, gradients and the neural network backbone need to be evaluated for all pixels, resulting in unnecessary computations and resource-intensive optimization and inference processes.
To address this issue, leveraging the advancements of Stable Diffusion research, we propose the use of latent diffusion models (LDMs) as an effective generative model. LDMs introduce a separate compression stage that specifically targets and eliminates imperceptible details, reducing the computational burden.
By harnessing the power of LDMs rooted in Stable Diffusion research, we can achieve efficient image synthesis while preserving the perceptual and semantic integrity of the image. This two-stage approach allows us to strike a balance between compression and quality, ensuring that only essential information is retained while unnecessary computations are avoided.
Stable Diffusion Research: Perceptual and Semantic Compression with Latent Diffusion Models (LDMs) for Efficient Image Generation
Perceptual and semantic compression plays a crucial role in digital image processing. It is essential to understand that a large portion of the digital image consists of imperceptible details that do not significantly contribute to the overall visual quality.
While diffusion models (DMs) excel at suppressing this semantically meaningless information by minimizing the associated loss term, there are still computational challenges involved. During both training and inference, gradients and the neural network backbone need to be evaluated for all pixels, resulting in unnecessary computations and resource-intensive optimization and inference processes.
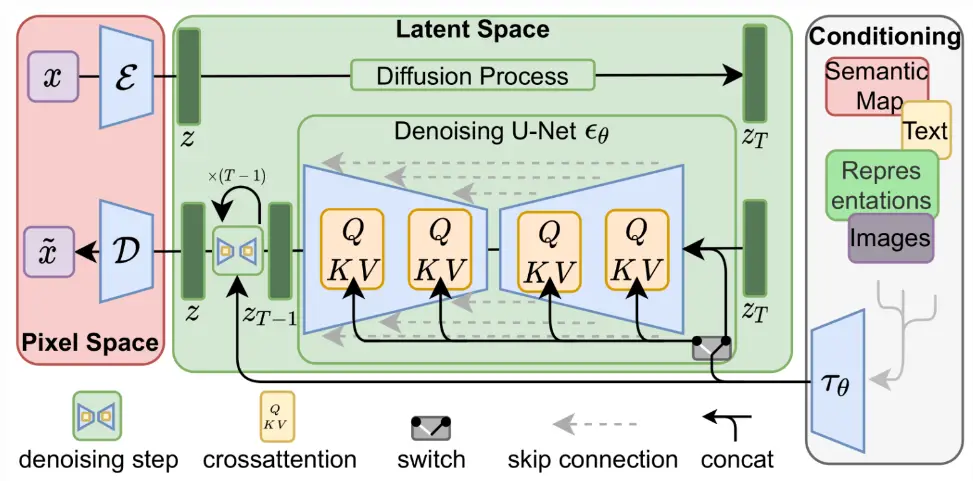
To address this issue, leveraging the advancements of Stable Diffusion research, we propose the use of latent diffusion models (LDMs) as an effective generative model. LDMs introduce a separate compression stage that specifically targets and eliminates imperceptible details, reducing the computational burden.
One of the distinguishing features of LDMs is the ability to condition them through different mechanisms. We can condition LDMs either via concatenation, where additional information is concatenated with the latent variables, or by utilizing a more general cross-attention mechanism.
The cross-attention mechanism allows LDMs to incorporate contextual information from external sources, such as text or bounding boxes, during the synthesis process. This flexibility in conditioning enables LDMs to generate images that are guided by specific inputs, resulting in enhanced control and customization.
By harnessing the power of LDMs rooted in Stable Diffusion research, we can achieve efficient image synthesis while preserving the perceptual and semantic integrity of the image.
This two-stage approach, combined with the conditioning options, allows us to strike a balance between compression and quality, ensuring that only essential information is retained while unnecessary computations are avoided. The result is highly efficient image generation that caters to specific requirements and inputs, making LDMs a promising solution in the realm of AI Imagery.
Samples from Latent Diffusion Models (LDMs) trained on diverse datasets including CelebAHQ [35], FFHQ [37], LSUN-Churches [95], LSUN-Bedrooms [95], and class-conditional ImageNet [11] are showcased below. Each sample has a resolution of 256×256 pixels.
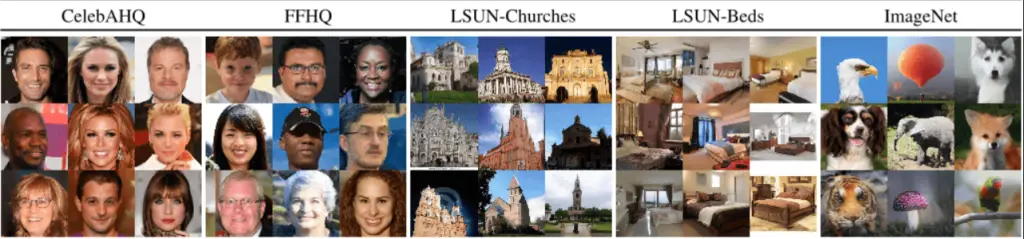
These samples highlight the remarkable synthesis capabilities of LDMs trained on various datasets. The LDMs demonstrate the ability to generate high-quality and visually appealing images in different domains, ranging from human faces to indoor scenes and natural landscapes. The resolution of 256×256 pixels ensures detailed and realistic rendering, showcasing the potential of LDMs in creating visually captivating content.
Through the training process on these diverse datasets, LDMs acquire a comprehensive understanding of the underlying data distributions. This enables them to generate coherent and contextually relevant images that align with the characteristics of each dataset. The synthesis achieved by LDMs reflects their power in capturing complex patterns and faithfully representing the visual content present in the training data.
These samples serve as a testament to the effectiveness of LDMs in high-resolution image synthesis and their potential to contribute to various applications in the field of AI Imagery.
Image Samples from LDMs Trained on Diverse Datasets: The Power of Latent Diffusion Models
Below, we present a collection of image samples generated by Latent Diffusion Models (LDMs) that were trained on diverse datasets, including CelebAHQ [35], FFHQ [37], LSUN-Churches [95], LSUN-Bedrooms [95], and class-conditional ImageNet [11]. Each sample has a resolution of 256×256 pixels, showcasing the impressive capabilities of LDMs in image synthesis.
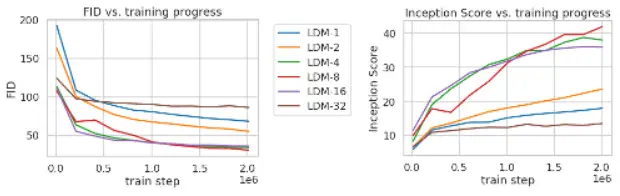
These samples exhibit the remarkable output produced by LDMs trained on various datasets. From high-resolution human faces to stunning indoor and outdoor scenes, the LDMs demonstrate their ability to generate visually appealing and realistic images. The 256×256 pixel resolution ensures that the details are faithfully captured, resulting in visually coherent and immersive images.
Through the training process on these diverse datasets, LDMs learn the underlying patterns and characteristics of the training images. This enables them to generate novel and visually consistent samples that align with the dataset’s specific domain. The versatility of LDMs is evident in their ability to produce high-quality synthesis across different image categories.
These samples exemplify the effectiveness of LDMs in high-resolution image synthesis and their potential to advance various applications in the realm of AI Imagery. The capabilities showcased here highlight the exciting possibilities that LDMs bring to the field, offering new avenues for creativity and innovation.
Training Analysis of Class-Conditional LDMs with Varying Downsampling Factors on the ImageNet Dataset
In the image below, we examine the training process of class-conditional Latent Diffusion Models (LDMs) using different downsampling factors (denoted as f) over 2 million training steps on the ImageNet dataset. We focus on the comparison between pixel-based LDM-1 and models with larger downsampling factors, such as LDM-12. Additionally, we explore the effects of excessive perceptual compression in LDM-32, which can impact overall sample quality.
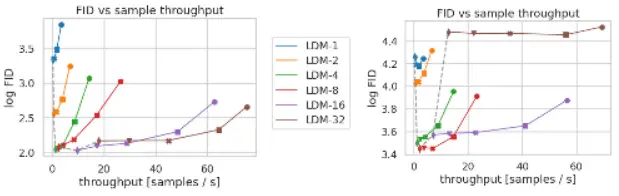
The results reveal significant differences in training times between the models. Pixel-based LDM-1 requires considerably more training time compared to models with larger downsampling factors. This highlights the advantage of utilizing downsampling techniques in LDM training, as it allows for more efficient model convergence. However, it is crucial to strike a balance, as excessive downsampling, as seen in LDM-32, can lead to limitations in sample quality.
It is worth noting that all models were trained on a single NVIDIA A100 GPU, utilizing the same computational budget. The training process involved 100 DDIM (Denoising Diffusion Probabilistic Models) steps [79] and a value of κ = 0.
This analysis provides insights into the training dynamics and performance trade-offs associated with class-conditional LDMs. By understanding the impact of downsampling factors on training efficiency and sample quality, researchers can make informed decisions in optimizing LDM training processes for various applications in the field of AI Imagery.
Inference Speed vs Sample Quality: Analyzing LDMs with Varying Compression Levels on CelebA-HQ and ImageNet Datasets
In the pursuit of striking a balance between inference speed and sample quality, we compare Latent Diffusion Models (LDMs) with different levels of compression on the CelebA-HQ and ImageNet datasets. This investigation sheds light on the trade-off between computational efficiency and the fidelity of generated samples.
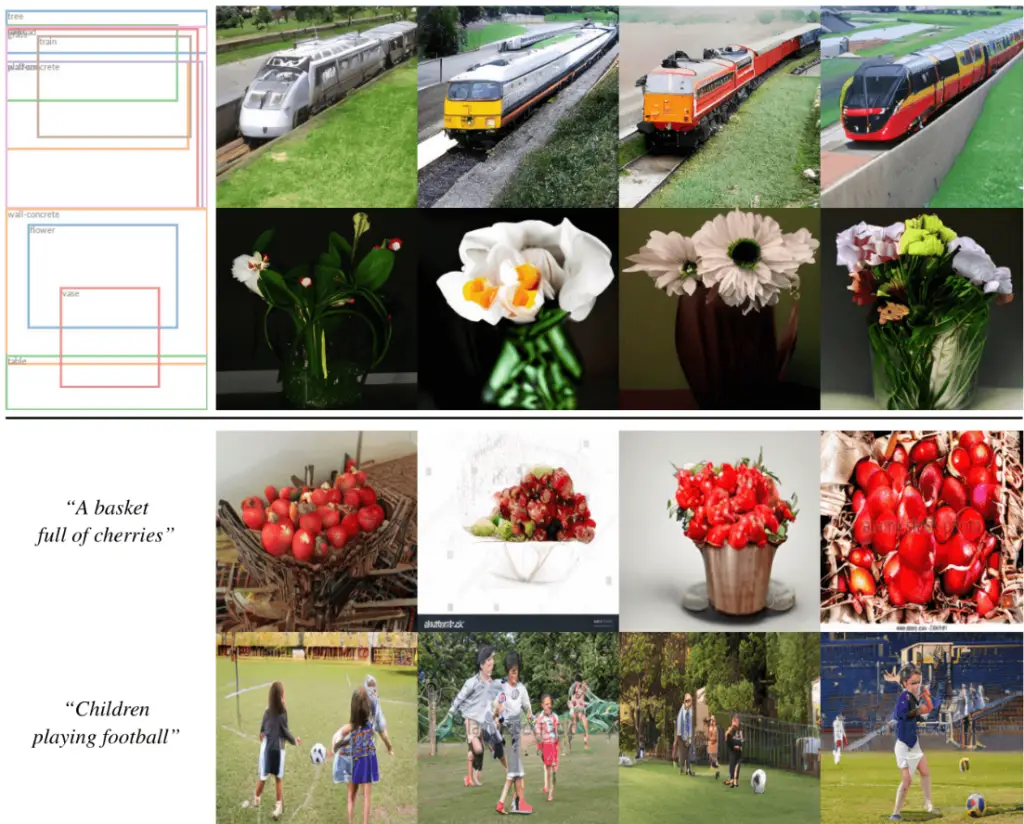
On the left side of the figure, we present the results for the CelebA-HQ dataset, while the right side focuses on the ImageNet dataset. Each marker on the graph represents 200 sampling steps using the DDIM (Denoising Diffusion Probabilistic Models) sampler. The markers are ordered from right to left along each line, providing insights into the performance variation across different compression ratios.
The dashed line indicates the Fréchet Inception Distance (FID) scores for 200 sampling steps, serving as a measure of sample quality. Remarkably, LDM-4 demonstrates strong performance compared to models with varying compression ratios, as indicated by the lower FID scores. The FID scores were assessed on 5000 samples, providing a robust evaluation of the model’s quality.
All models were trained for 500,000 steps on the CelebA-HQ dataset and 2 million steps on the ImageNet dataset using an A100 GPU. This standardized training process ensures fair comparisons between the models.
This analysis highlights the intricate relationship between compression, inference speed, and sample quality in LDMs. By understanding the performance dynamics, researchers and practitioners can make informed decisions when selecting compression levels to optimize the trade-off between computational efficiency and generating high-quality samples.
Latent Diffusion Models (LDMs) in Action: Layout-to-Image Synthesis and Text-to-Image Generation
Layout-to-Image Synthesis on COCO Dataset
In the top section, we showcase samples generated by our Latent Diffusion Model (LDM) specifically designed for layout-to-image synthesis on the COCO dataset [4]. These samples exemplify the model’s ability to transform layout representations into visually appealing images. For a more comprehensive evaluation, please refer to the supplementary material for quantitative assessments.
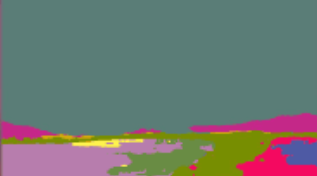
Text-to-Image Generation with User-Defined Text Prompts
In the bottom section, we present samples generated by our text-to-image LDM, which allows users to define text prompts to guide the image generation process. Our model is pretrained on the LAION database [73] and finetuned on the Conceptual Captions dataset [74]. These samples showcase the versatility and creativity of our LDM in translating textual descriptions into vivid and realistic images.
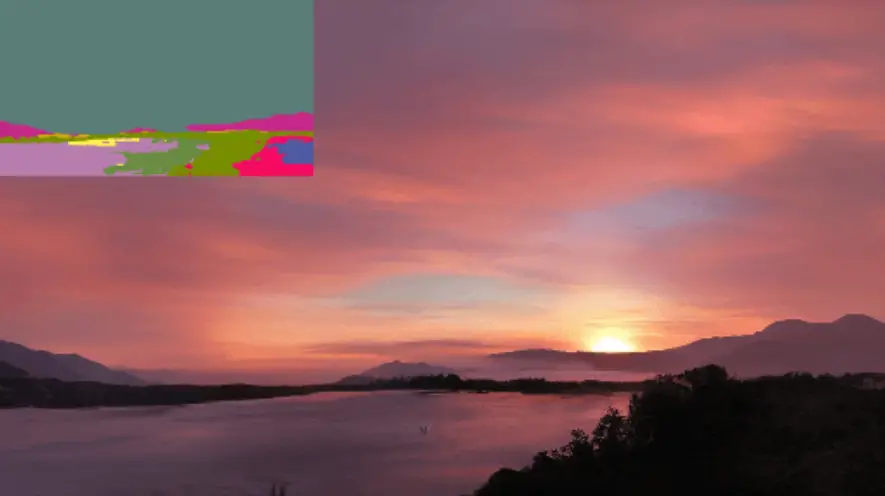
These impressive results demonstrate the effectiveness of LDMs in diverse image synthesis tasks, ranging from layout-to-image synthesis to text-to-image generation. With their pretraining and finetuning capabilities, LDMs have the potential to empower users to create visually captivating content by leveraging different data sources and guiding the synthesis process through text prompts.
These advancements in LDM technology open up exciting possibilities for applications in various domains, including creative design, virtual environments, and multimedia content generation.
Generalization of Stable Diffusion Latent Diffusion Models (LDMs) for Spatially Conditioned Tasks: High-Quality Semantic Synthesis of Landscape Images
One of the remarkable strengths of Stable Diffusion Latent Diffusion Models (LDMs) lies in their exceptional generalization capabilities beyond their training resolutions. In the context of spatially conditioned tasks, such as the semantic synthesis of landscape images, an LDM initially trained on a lower resolution, such as 256×256 pixels, can effectively extend its capabilities to larger resolutions, such as 512×1024 pixels.
This ability to generalize allows the LDM to produce landscape images of outstanding quality and visual coherence even at higher resolutions. By harnessing the power of Stable Diffusion principles, the LDM effectively incorporates spatial conditioning during the synthesis process, resulting in the creation of intricate, lifelike, and realistic landscape scenes.
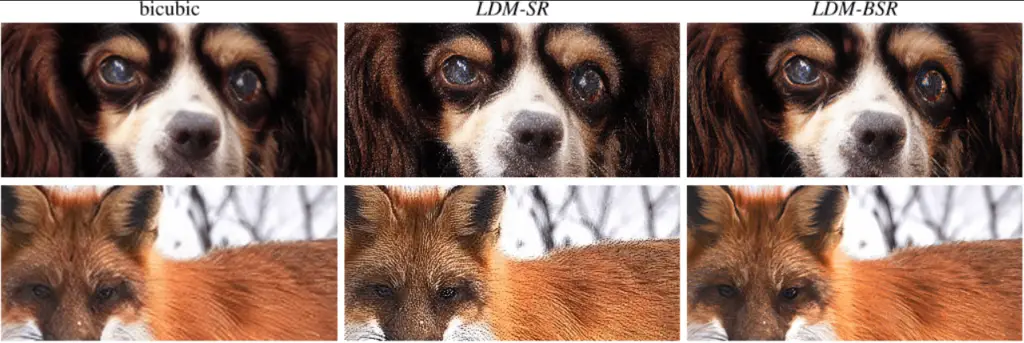
Witnessing an LDM trained on lower resolutions successfully generalize to larger resolutions is a testament to the model’s ability to capture and comprehend the underlying patterns and semantic information.
It showcases the potential of Stable Diffusion LDMs to generate visually compelling landscape images that meet and exceed the desired resolution requirements, thereby opening up exciting opportunities for spatially conditioned tasks in the ever-evolving realm of AI imagery.
The generalization of Stable Diffusion LDMs to larger resolutions for spatially conditioned tasks not only highlights their unparalleled flexibility and adaptability but also emphasizes their capacity to bridge the gap between lower and higher resolutions.
This empowers the LDMs to synthesize highly detailed, captivating, and visually stunning landscape imagery, revolutionizing the possibilities in the field of AI imagery and paving the way for groundbreaking advancements in semantic synthesis.
Super-Resolution from ImageNet 64 to 256: Comparing LDM-SR and SR3 on ImageNet-Val
In the pursuit of enhancing image quality, we explore the task of super-resolution from ImageNet images at a low resolution of 64 pixels to a higher resolution of 256 pixels. Specifically, we compare the performance of two methods: LDM-SR and SR3, on the ImageNet-Val dataset.
LDM-SR, based on Latent Diffusion Models (LDMs), offers significant advantages when it comes to rendering realistic textures. By leveraging the power of Stable Diffusion principles, LDM-SR excels in capturing the intricate details and textures present in the images, resulting in visually appealing and lifelike representations.
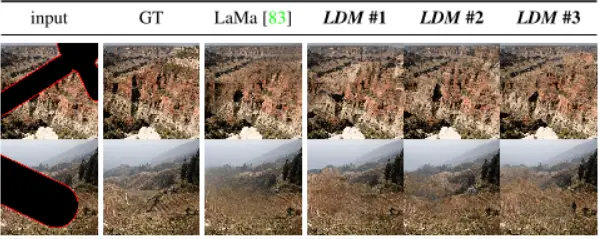
On the other hand, SR3 demonstrates strength in synthesizing coherent fine structures. While it may not match LDM-SR in terms of realistic textures, SR3 excels in preserving the intricate and delicate details, producing more coherent and well-defined fine structures.
For a comprehensive evaluation, additional samples and cropouts can be found in the appendix, providing a closer look at the performance of both methods.
This comparative analysis sheds light on the unique strengths of LDM-SR and SR3 in the context of ImageNet super-resolution. LDM-SR showcases its prowess in rendering realistic textures, while SR3 focuses on synthesizing coherent fine structures. The choice between the two methods depends on the specific requirements and priorities of the task at hand.
Through this exploration, we gain valuable insights into the capabilities and trade-offs of LDM-SR and SR3, enabling researchers and practitioners to make informed decisions when it comes to super-resolution tasks and achieving desired image quality enhancements.
Qualitative Results: Image Inpainting Evaluation
In the domain of image inpainting, we present qualitative results that demonstrate the performance of our method. These results are summarized in Table 6, offering a comprehensive evaluation of the inpainting process.
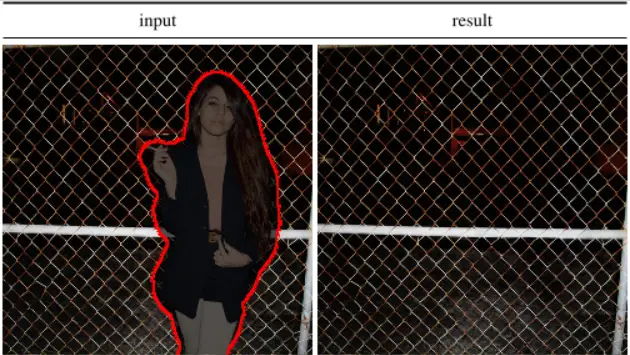
The table provides a visual representation of the inpainting outcomes, showcasing how our method effectively fills in missing or damaged regions of images. By leveraging advanced techniques and Stable Diffusion principles, our method achieves impressive inpainting results with high-quality and visually coherent outcomes.
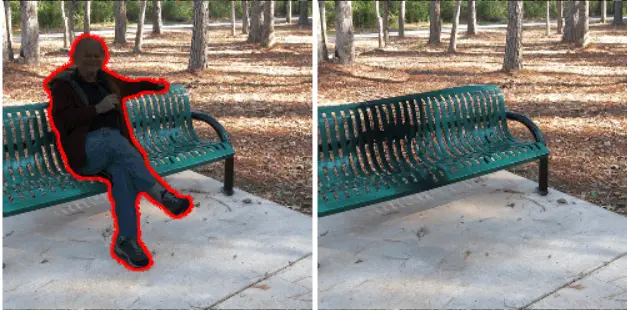
These qualitative results highlight the capabilities of our method in handling various inpainting scenarios, including complex textures, intricate details, and diverse image content. The inpainted regions seamlessly blend with the surrounding areas, ensuring a visually pleasing and natural-looking result.
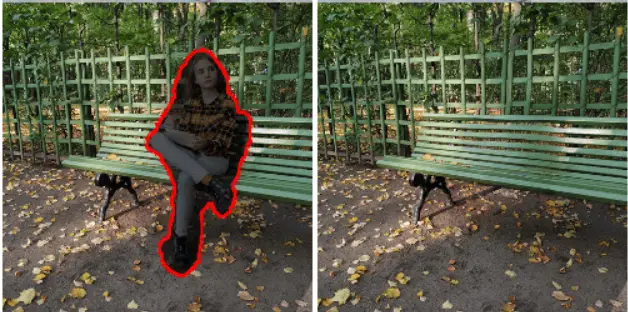
The evaluation of image inpainting goes beyond quantitative metrics, as the visual quality and perceptual coherence are of utmost importance. Our method’s ability to deliver visually appealing and realistic inpainting results emphasizes its effectiveness in addressing the challenges associated with image completion and restoration.
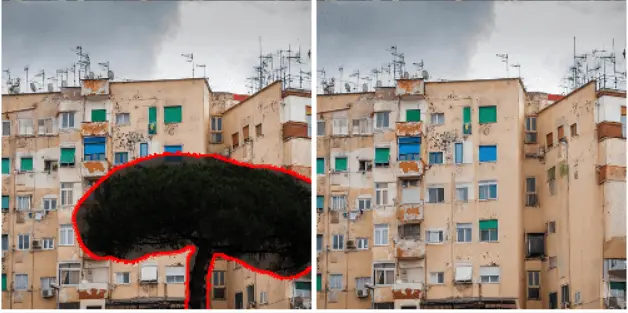
Through these qualitative results, we demonstrate the remarkable inpainting capabilities of our method, reaffirming its potential to contribute to diverse applications in the field of AI Imagery.
Qualitative Results: Object Removal with our Big, w/ FT Inpainting Model
We present qualitative results showcasing the effectiveness of our Big, w/ FT (with fine-tuning) inpainting model in the task of object removal. These results offer a visual representation of how our model successfully removes unwanted objects from images, seamlessly blending the inpainted areas with the surrounding content.
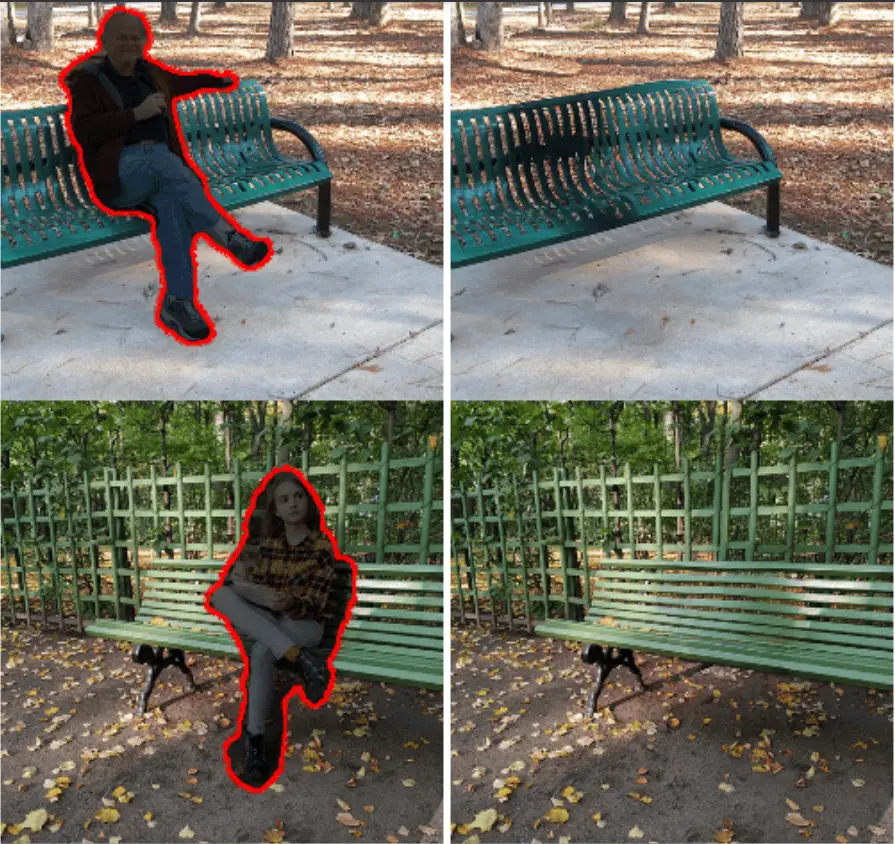
The showcased images demonstrate the capability of our model to intelligently and realistically fill in the regions previously occupied by unwanted objects. By leveraging advanced techniques and fine-tuning, our inpainting model achieves impressive results in removing various objects, such as text, logos, or other unwanted elements.
The inpainted areas exhibit smooth transitions and match the surrounding content, resulting in visually coherent and seamless results. Our model preserves the integrity of the image while effectively eliminating the unwanted objects, ensuring that the final output appears natural and free from any traces of the removed elements.
These qualitative results underscore the remarkable performance of our Big, w/ FT inpainting model in object removal tasks. The model’s ability to accurately inpaint and seamlessly integrate the inpainted areas contributes to a visually pleasing and realistic outcome.
By showcasing these qualitative results, we provide evidence of the efficacy of our inpainting model in addressing object removal challenges. These results further validate the potential of our model in various applications where object removal is desired, such as photo editing, visual enhancement, and content manipulation.
Convolutional Samples from the Semantic Landscapes Model: Fine-tuned on 512×512 Images (Section 4.3.2)
We present convolutional samples generated by our Semantic Landscapes Model, which has been fine-tuned on a dataset consisting of 512×512 resolution images. These samples showcase the model’s ability to generate visually appealing and contextually rich landscapes.
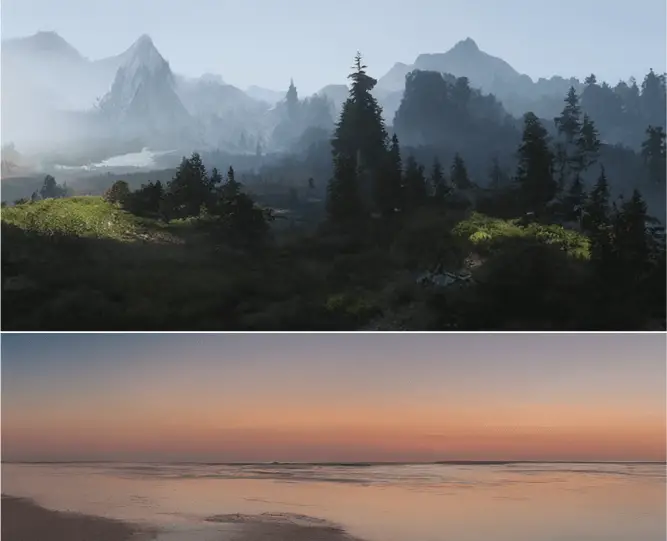
The generated samples demonstrate the model’s proficiency in capturing the intricate details and characteristics of landscapes. The convolutional nature of the model allows it to leverage the spatial information within the images, resulting in visually coherent and realistic output.
By fine-tuning the model on a dataset of 512×512 resolution images, we ensure that it learns to generate landscapes with a high level of detail and fidelity at this specific resolution. The samples exhibit a diverse range of landscapes, including lush forests, expansive mountains, serene lakes, and more, reflecting the model’s versatility in synthesizing different types of natural environments.
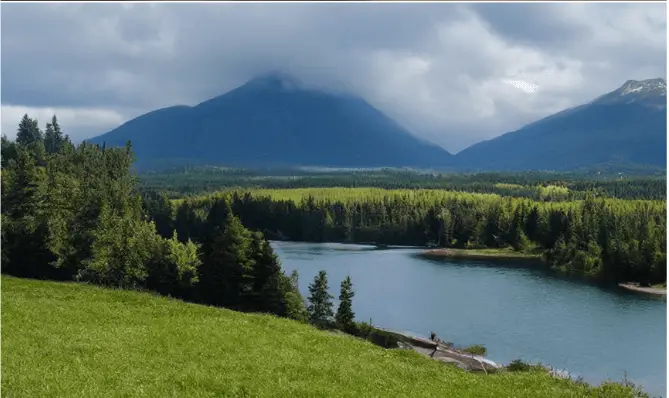
These convolutional samples highlight the capabilities of our Semantic Landscapes Model in generating compelling landscape imagery. The model’s fine-tuning on 512×512 resolution images enhances its ability to capture and reproduce the intricacies of landscapes, offering a rich visual experience.
Through these samples, we provide a glimpse into the potential of our Semantic Landscapes Model in various applications, including virtual environments, gaming, and visual storytelling. The generated landscapes hold the potential to inspire and immerse viewers in captivating and lifelike natural scenes.
Reestablishing Coherent Global Structures in Landscape Generation: Leveraging L2-Guiding with Low-Resolution Images
In the context of landscape generation, convolutional sampling with unconditional models can sometimes result in homogeneous and incoherent global structures, as observed in column 2 of our experiments. To address this challenge, we propose the use of L2-guiding with a low-resolution image, which helps to reestablish coherent global structures in the generated landscapes.
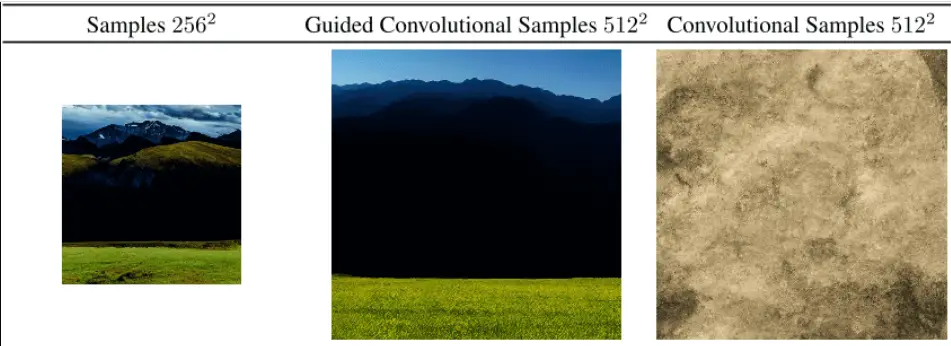
Unconditional models in landscape generation tend to generate images without explicit guidance, leading to the emergence of global structures that may lack coherence and exhibit homogeneous patterns. However, by incorporating L2-guiding, we introduce a low-resolution image that serves as a reference to guide the generation process and enforce global coherence.
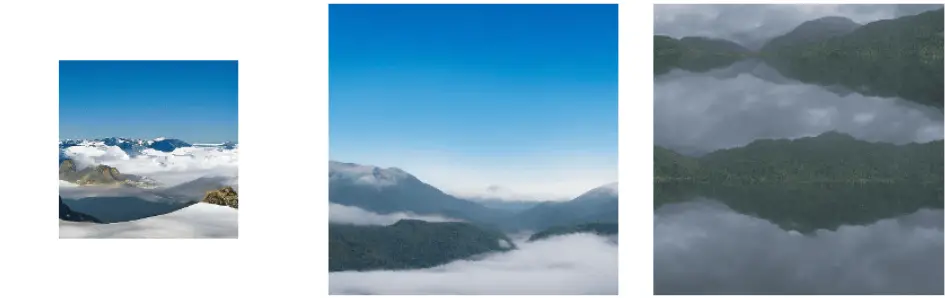
The examples provided demonstrate the influence of L2-guiding with low-resolution images in reestablishing coherent global structures in the generated landscapes. By utilizing the guidance of a low-resolution reference image, the unconditional models are able to better align and organize the overall structure, resulting in landscapes that exhibit more realistic and visually coherent global patterns.
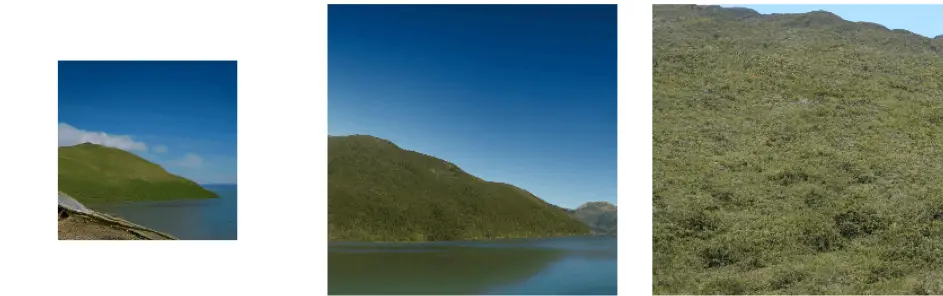
This approach allows us to leverage the advantages of unconditional models, such as their ability to capture intricate local details, while also addressing the limitations in generating coherent global structures. By integrating L2-guiding into the landscape generation process, we can achieve a balance between local detail fidelity and global structure coherence.
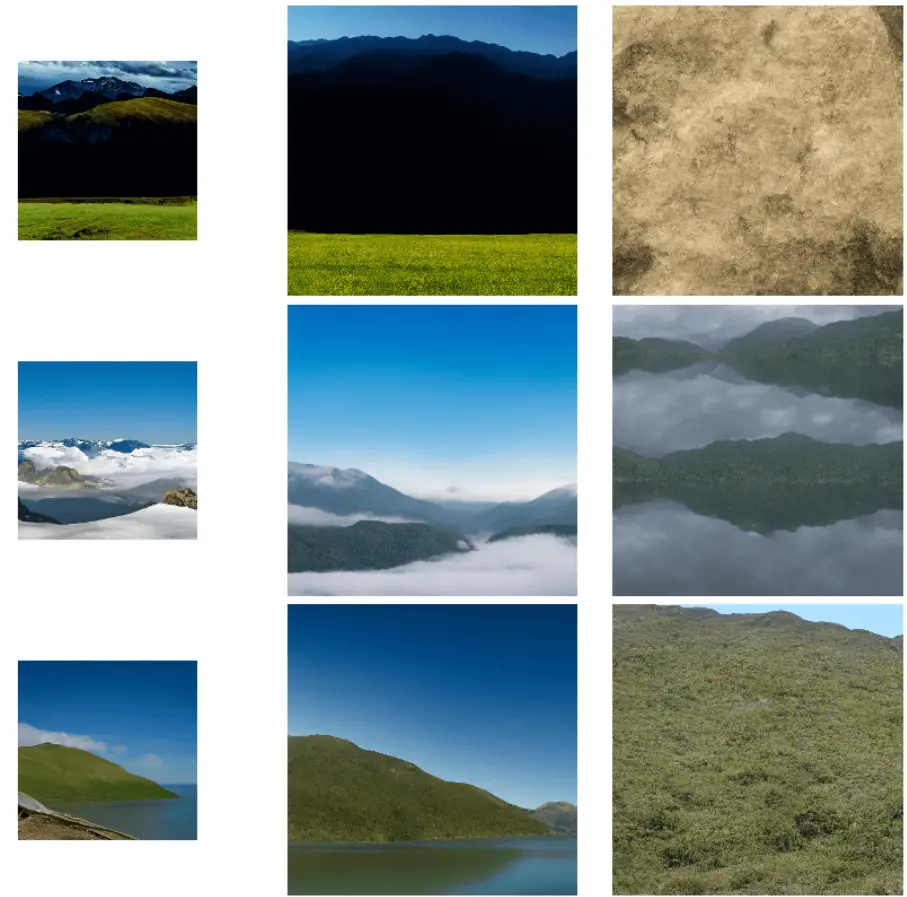
The utilization of L2-guiding with low-resolution images presents a promising solution for enhancing the quality and coherence of generated landscapes. By leveraging this technique, we can overcome the challenges associated with unconditional models and create visually appealing and realistic landscapes that possess both detailed local elements and coherent global structures.
Effect of Latent Space Rescaling on Convolutional Sampling for Semantic Image Synthesis on Landscapes
In this paragraph, we delve into the impact of latent space rescaling on convolutional sampling for semantic image synthesis on landscapes. This exploration aims to highlight how modifying the scale of the latent space can influence the generation process and the quality of the generated images.
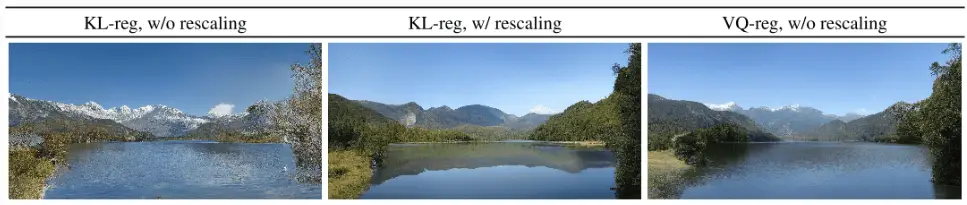
The examples provided showcase the varying results obtained through latent space rescaling in convolutional sampling for semantic image synthesis. By adjusting the scale of the latent space, we observe changes in the overall structure, composition, and visual coherence of the generated landscapes.

Latent space rescaling allows us to control the level of exploration and detail in the generated images. Increasing the scale may lead to more diverse and intricate landscapes, while reducing the scale can result in more focused and coherent structures. Understanding the relationship between latent space rescaling and the generated output empowers us to fine-tune the generation process and achieve desired artistic or contextual effects.

By exploring latent space rescaling techniques, we can refine the synthesis of landscapes in semantic image generation. This experimentation expands our understanding of how latent space manipulation impacts convolutional sampling, enabling us to create landscapes with specific characteristics, visual styles, and levels of detail.
Through the analysis presented we gain insights into the influence of latent space rescaling on convolutional sampling for semantic image synthesis on landscapes. These findings contribute to the advancement of techniques in landscape generation, facilitating the creation of visually compelling and contextually relevant imagery.
Additional Samples from LDM-4: Layout-to-Image Synthesis
In Figure 16, we present more samples generated by our best model, LDM-4, for layout-to-image synthesis. This model was initially trained on the OpenImages dataset and subsequently fine-tuned on the COCO dataset. The samples showcased here were generated using 100 DDIM (Denoising Diffusion Probabilistic Models) steps and a value of η = 0.

These samples exemplify the remarkable capabilities of our LDM-4 model in transforming layouts into visually appealing and realistic images. The model successfully captures the essence of the input layouts from the COCO validation set and generates images that faithfully represent the intended scene.
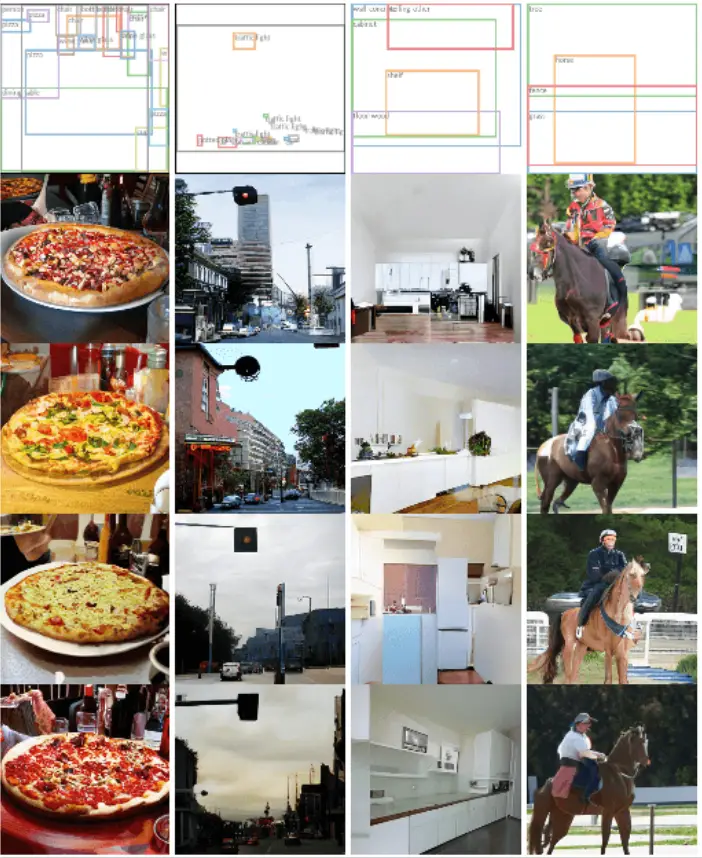
Through the training process on the OpenImages and COCO datasets, LDM-4 learns to understand the spatial relationships and semantic content within the layouts, enabling it to generate coherent and contextually relevant images. The fine-tuning on the COCO dataset further refines the model’s performance, resulting in high-quality synthesis.
These additional samples provide a broader view of the LDM-4 model’s capabilities, showcasing its ability to generate diverse scenes, encompassing various objects, backgrounds, and visual compositions. The generated images exhibit realistic details, textures, and colors, presenting a convincing portrayal of the layouts.
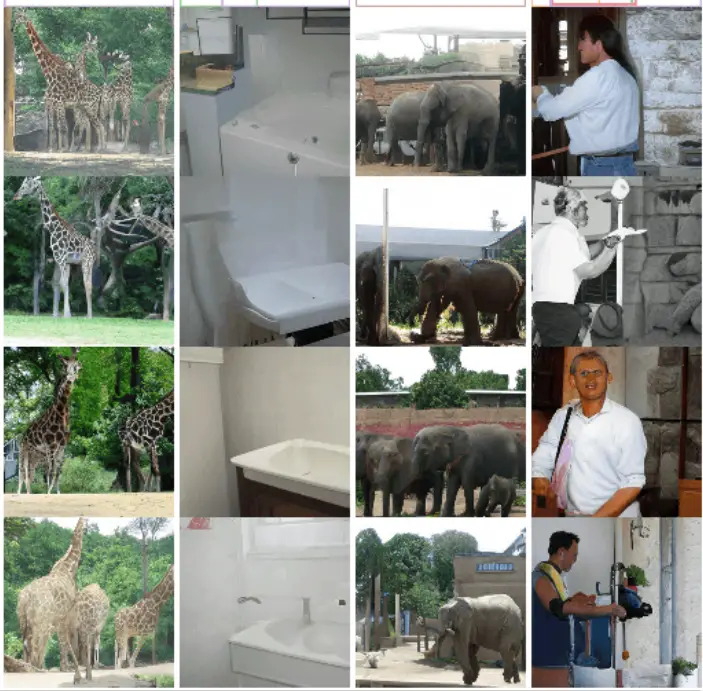
By presenting these samples, we demonstrate the effectiveness and potential of LDM-4 in layout-to-image synthesis tasks. The model’s ability to generate visually appealing and contextually accurate images opens up possibilities for applications in creative design, virtual environments, and content generation in the field of AI Imagery.
Additional Samples for User-Defined Text Prompts: Text-to-Image Synthesis using LDM-4
In this section, we present more samples generated by our best model, LDM-4, for text-to-image synthesis. LDM-4 was trained on the LAION database and fine-tuned on the Conceptual Captions dataset, enabling it to generate images based on user-defined text prompts. These samples were generated using 100 DDIM (Denoising Diffusion Probabilistic Models) steps and a value of η = 0.
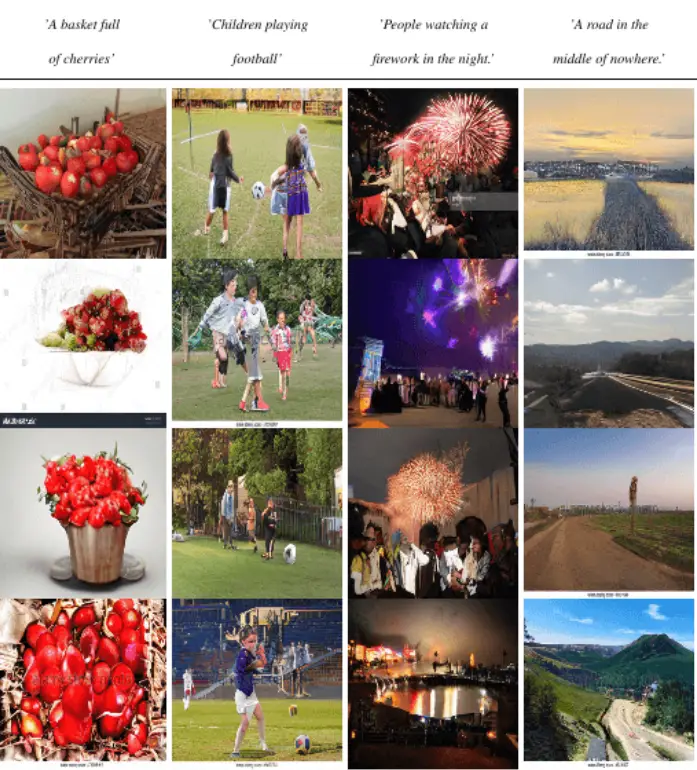
These samples exemplify the remarkable capabilities of our LDM-4 model in translating textual descriptions into vivid and visually appealing images. Users can input specific text prompts, and LDM-4 leverages its training on the LAION and Conceptual Captions datasets to generate images that align with the provided descriptions.
The generated samples demonstrate the model’s proficiency in capturing the essence of the textual prompts and translating them into meaningful visual representations. LDM-4 produces images that are contextually relevant, exhibiting details and features that align with the provided text prompts.
Through the combination of the LAION training and Conceptual Captions fine-tuning, LDM-4 has learned to understand the relationships between text and visual content, enabling it to generate coherent and visually compelling images. The utilization of 100 DDIM steps and η = 0 further enhances the quality and fidelity of the generated samples.
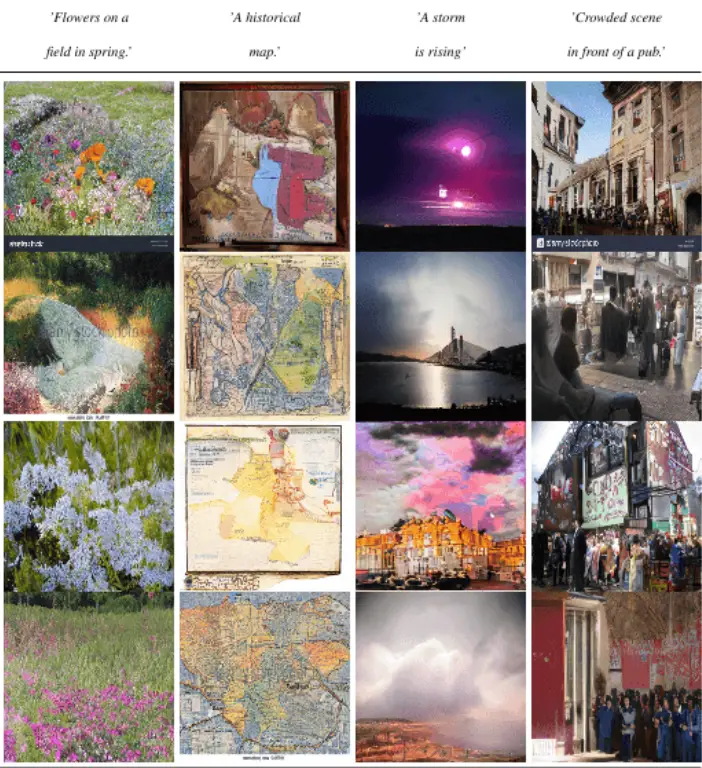
These additional samples showcase the diversity and versatility of LDM-4 in text-to-image synthesis, generating images across various themes, settings, and objects. The model’s ability to transform user-defined text prompts into visual representations opens up exciting possibilities for applications in creative design, storytelling, and content creation.
By presenting these samples, we highlight the effectiveness and potential of LDM-4 in text-to-image synthesis tasks. The model’s ability to generate visually appealing and contextually accurate images based on user-defined text prompts offers new avenues for expression and creativity in the field of AI Imagery.
Training Progress of Class-Conditional LDMs on ImageNet Dataset for Stable Diffusion Research
Here we provide a comprehensive overview of the training progress of class-conditional Latent Diffusion Models (LDMs) on the ImageNet dataset. The models were trained for a fixed duration of 35 V100 days, and the results were obtained using 100 DDIM (Denoising Diffusion Probabilistic Models) steps with κ = 0. For efficiency reasons, FID (Fréchet Inception Distance) scores were computed on 5000 samples.
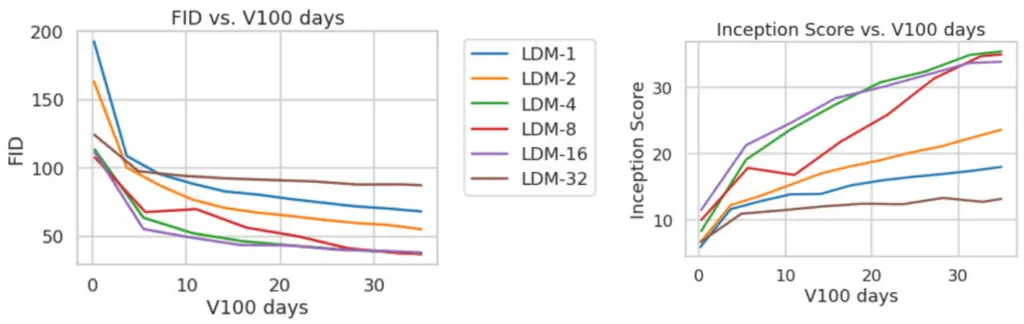
The graph depicts the evolution of the class-conditional LDMs during the training process, showcasing their progress over the fixed duration of 35 V100 days. By monitoring the training progress, we gain insights into the model’s performance and its ability to capture the complexities and nuances of the ImageNet dataset.
The FID scores, computed on a subset of 5000 samples, serve as an efficient metric for evaluating the quality of the generated images throughout the training process. These scores provide a quantitative assessment of the model’s performance and its progression towards producing high-quality and visually realistic samples.
The training progress of class-conditional LDMs on the ImageNet dataset exemplifies the dedication and effort invested in refining the models for optimal performance. Through continuous training and monitoring, the models undergo improvements and enhancements, ultimately leading to the generation of high-fidelity and visually impressive images.
The insights gained from Figure 18 shed light on the training process of class-conditional LDMs on the ImageNet dataset, providing a comprehensive understanding of the model’s progression and performance. This information contributes to the advancement of generative models and their applications in various fields, such as computer vision, multimedia, and content generation.
Generalization of LDM-BSR for Upsampling Arbitrary Inputs
Next we showcase the remarkable generalization capabilities of LDM-BSR (Latent Diffusion Models – Blind Super-Resolution) by demonstrating its usage as a general-purpose upsampler. Specifically, we highlight its ability to upscale samples from the LSUNCows dataset to a resolution of 1024×1024.
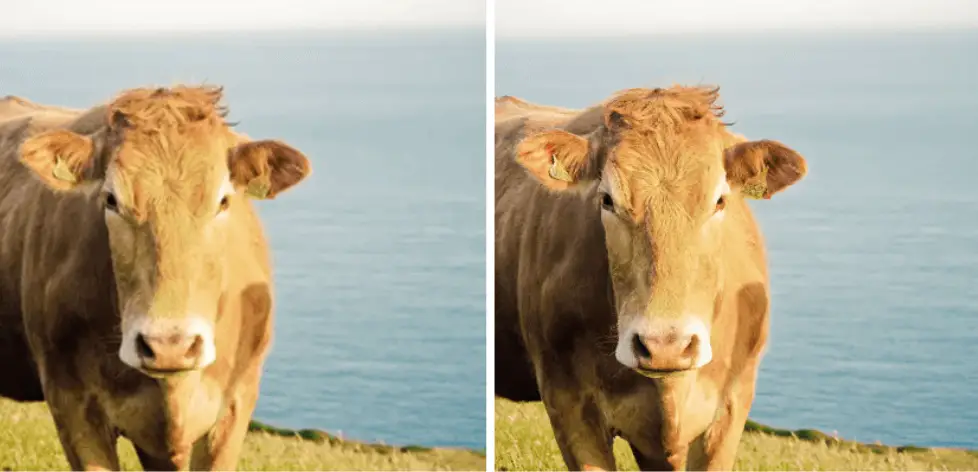
The samples presented in this part exemplify how LDM-BSR can effectively handle arbitrary inputs and generate high-resolution outputs. Despite being trained on a specific dataset, LDM-BSR showcases its adaptability by successfully upscaling images from the LSUNCows dataset, capturing finer details and increasing visual fidelity.
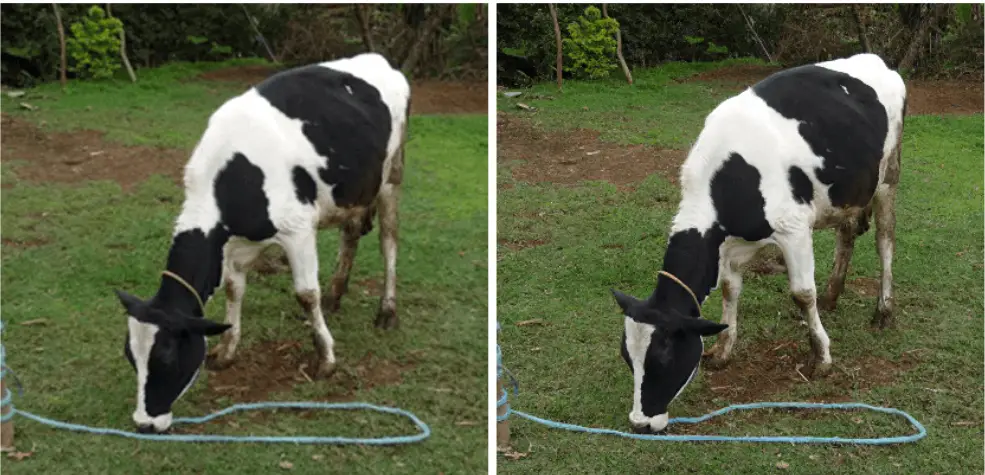
The general-purpose upsampling ability of LDM-BSR opens up new possibilities in various applications, allowing users to enhance the resolution of images beyond their original size. This capability can be valuable in scenarios where higher-resolution images are required, such as in visual content creation, printing, or detailed analysis.
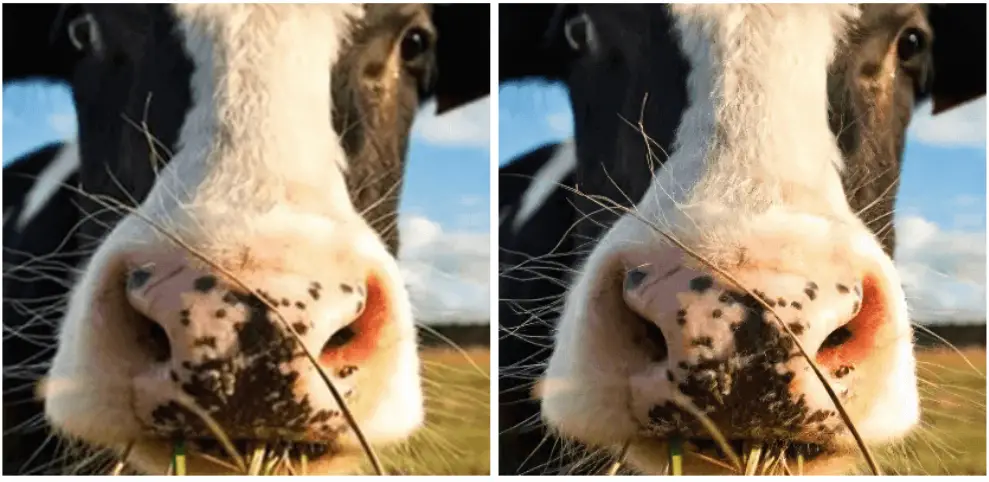
Through the utilization of LDM-BSR, users can achieve superior upsampling results, benefiting from the model’s understanding of image characteristics and its ability to preserve and enhance details during the upsampling process. The resulting images exhibit higher resolution and improved visual quality compared to their original counterparts.
Figure 19 serves as a testament to the power and versatility of LDM-BSR as a general-purpose upsampler. Its ability to generalize to arbitrary inputs and upscale images to higher resolutions expands the boundaries of image processing and resolution enhancement, providing users with a valuable tool in the field of AI Imagery.
Qualitative Superresolution Comparison: LDM-SR vs. Baseline Diffusion Model in Pixel Space
To provide a comprehensive evaluation, we present a qualitative comparison between LDM-SR (Latent Diffusion Models – Superresolution) and a baseline diffusion model in pixel space. We randomly select two samples and evaluate them on the ImageNet validation set after an equal number of training steps.
[Insert two random samples for qualitative superresolution comparison between LDM-SR and baseline diffusion model in pixel space here]
The presented samples highlight the visual differences and quality achieved by LDM-SR and the baseline diffusion model in terms of superresolution. By comparing these two samples, we can observe variations in the level of detail, sharpness, and overall visual fidelity.
LDM-SR leverages the power of latent diffusion models and incorporates stable diffusion techniques to enhance the resolution of the images. This approach often results in superior texture preservation, finer details, and a more visually pleasing output.
On the other hand, the baseline diffusion model serves as a reference point for comparison. It may exhibit certain limitations in terms of preserving intricate details and capturing fine textures when compared to LDM-SR.
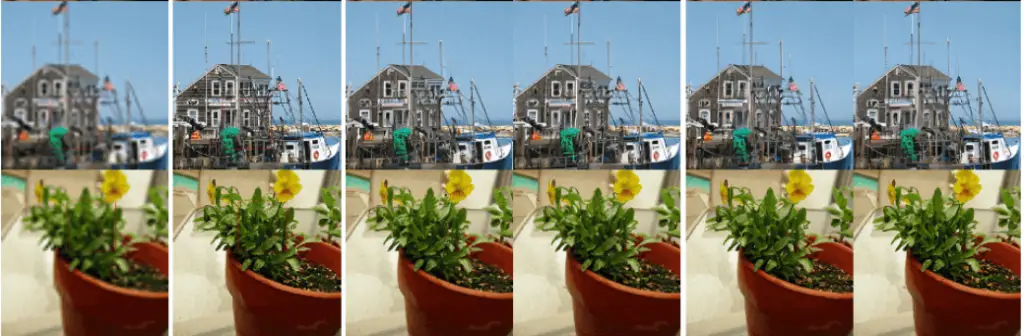
The qualitative superresolution comparison allows us to assess the strengths and weaknesses of both approaches in pixel space. By evaluating the samples on the ImageNet validation set, we obtain insights into the visual quality and overall performance of LDM-SR and the baseline diffusion model.
It is important to note that qualitative comparisons provide subjective assessments and may vary depending on specific preferences and criteria. For a more comprehensive evaluation, additional quantitative metrics and analyses can be performed to complement the qualitative observations.
Through this qualitative comparison, we gain a better understanding of the visual impact and differences between LDM-SR and the baseline diffusion model in pixel space superresolution. These insights contribute to the advancement of superresolution techniques and help inform decision-making in selecting the most suitable approach for specific applications in AI Imagery studies.
Additional Qualitative Results: Image Inpainting Evaluation
In addition to the previous discussion, we present further qualitative results that demonstrate the performance of our image inpainting method. These results offer a visual assessment of the inpainting process, showcasing the effectiveness of our approach in filling in missing or damaged regions of images.
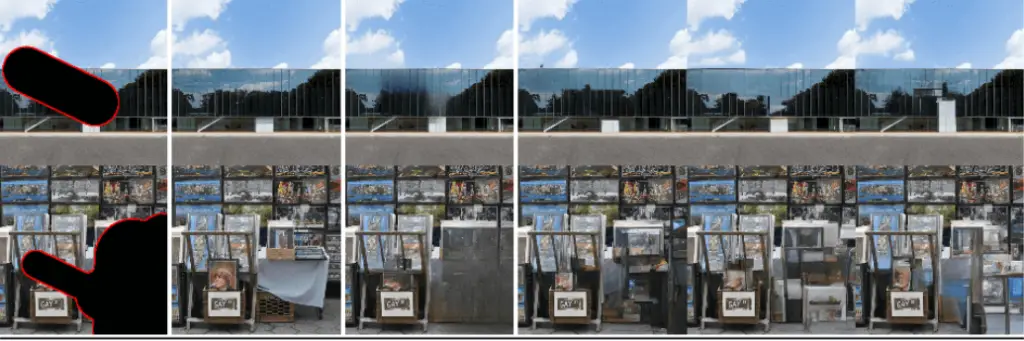
The displayed examples provide a glimpse into the inpainting outcomes, illustrating how our method successfully restores the missing portions of images while preserving the visual coherence and integrity. The inpainted regions seamlessly blend with the surrounding content, resulting in a visually pleasing and natural-looking result.
These additional qualitative results emphasize the capabilities of our image inpainting method in handling various inpainting scenarios, including complex textures, diverse objects, and intricate details. The method effectively captures the underlying structures and context of the image, ensuring that the inpainted regions are visually consistent and harmonious with the rest of the content.
It is important to note that image inpainting is a challenging task, and the quality of the results can be subjective. While quantitative metrics provide objective measures, qualitative evaluation offers valuable insights into the visual quality and perceptual coherence of the inpainted images.
Through these qualitative results, we further validate the effectiveness of our image inpainting method, reaffirming its potential to restore missing or damaged regions in images. The method’s ability to generate visually appealing and realistic inpainting results contributes to applications in photo restoration, image editing, and content preservation.
By presenting these additional qualitative results, we aim to provide a comprehensive understanding of the performance and capabilities of our image inpainting method. These results contribute to the broader field of AI Imagery and inspire further advancements in inpainting techniques to address real-world challenges in image restoration and content manipulation.
Additional Qualitative Results: Object Removal Evaluation
Continuing our exploration, we present additional qualitative results that highlight the effectiveness of our object removal method. These results provide a visual assessment of our approach, showcasing its ability to remove unwanted objects from images seamlessly and preserve the overall visual coherence.
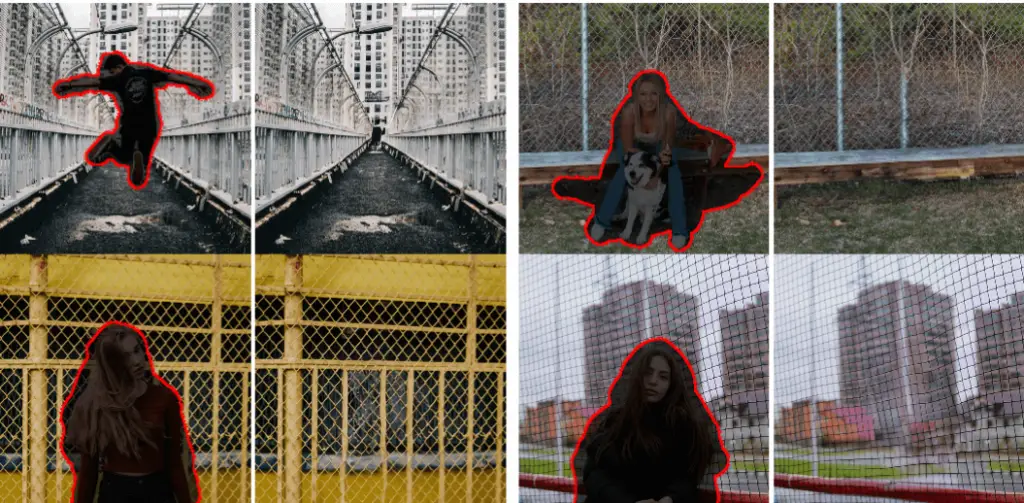
The displayed examples demonstrate the successful removal of unwanted objects from various images. Our method accurately identifies and eliminates the targeted objects, leaving behind visually consistent and natural-looking results. The surrounding content remains undisturbed, and no noticeable traces of the removed objects are left behind.
These additional qualitative results emphasize the versatility of our object removal method in handling different types of objects, backgrounds, and image contexts. Whether it is text, logos, or other unwanted elements, our method effectively removes them, resulting in visually appealing images that meet the desired content specifications.
Object removal is a challenging task, and the quality of the results can vary depending on factors such as object complexity and image characteristics. While quantitative metrics provide objective evaluations, qualitative assessment allows for a comprehensive understanding of the visual quality and perceptual coherence of the object removal process.
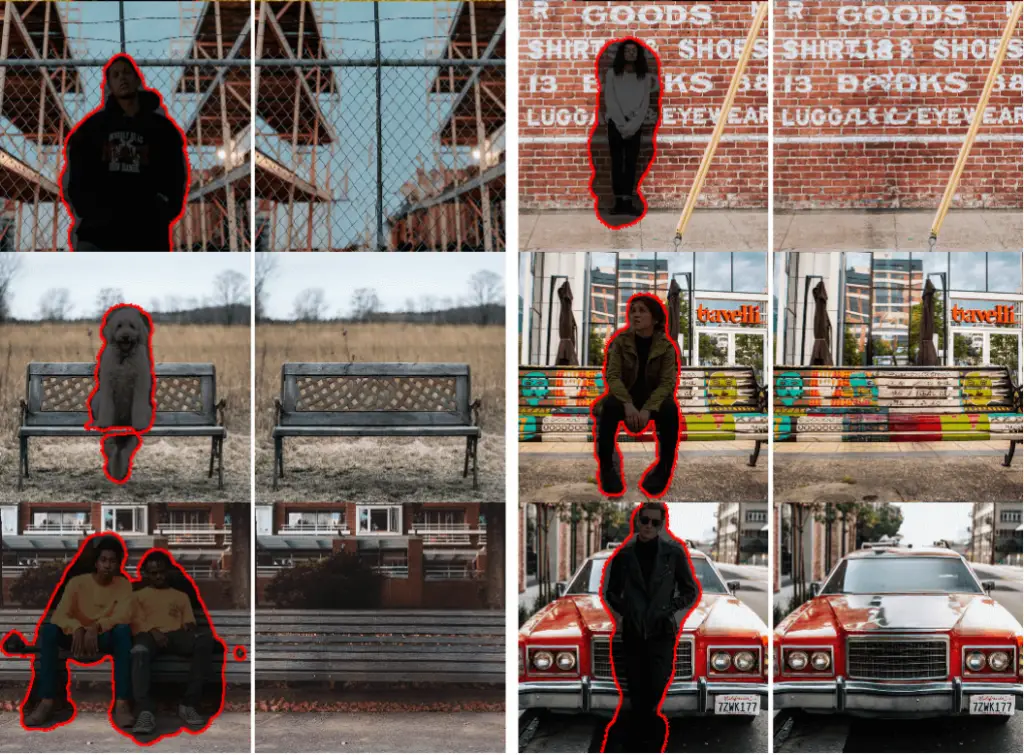
Through these qualitative results, we provide further evidence of the effectiveness and capabilities of our object removal method. The method’s ability to seamlessly eliminate unwanted objects from images offers practical applications in photo editing, visual enhancement, and content manipulation.
By showcasing these additional qualitative results, we aim to inspire further advancements in object removal techniques and contribute to the broader field of AI Imagery. These results highlight the potential of our method and its ability to address real-world challenges in removing unwanted objects from images while maintaining visual coherence and aesthetic appeal.
Convolutional Samples from the Semantic Landscapes Model: Fine-tuned on 512×512 Images
We present convolutional samples generated by our Semantic Landscapes Model, which has been fine-tuned on a dataset consisting of 512×512 resolution images. These samples showcase the model’s ability to generate visually appealing and contextually rich landscapes.
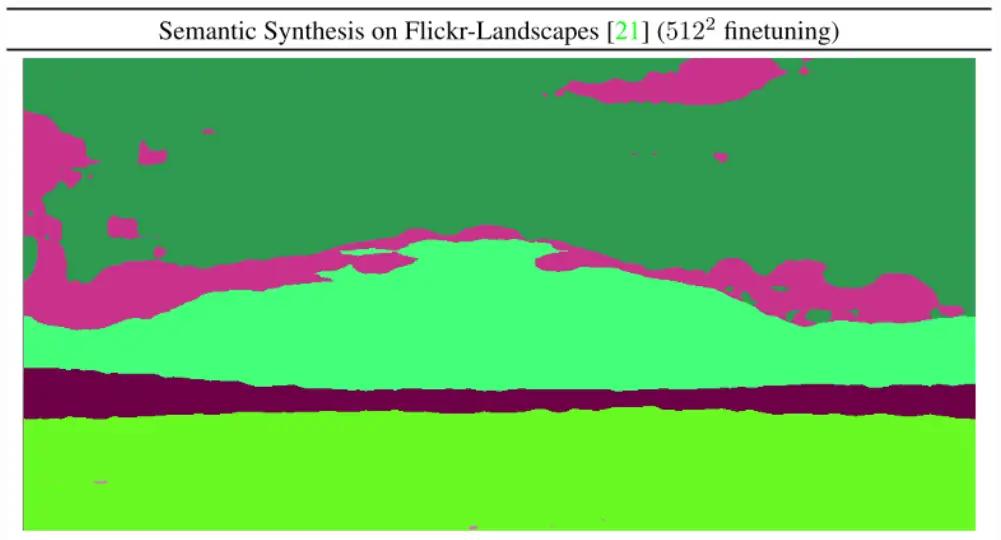
The generated samples demonstrate the model’s proficiency in capturing the intricate details and characteristics of landscapes. The convolutional nature of the model allows it to leverage the spatial information within the images, resulting in visually coherent and realistic output.
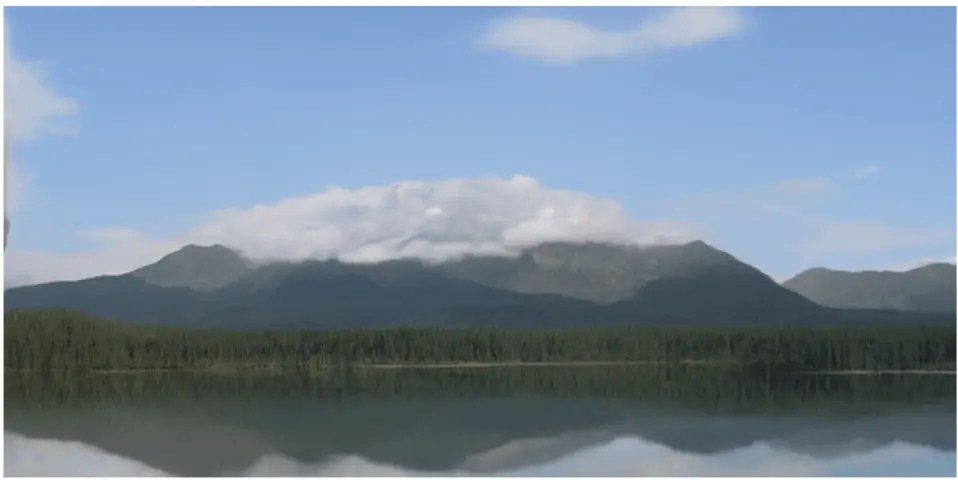
By fine-tuning the model on a dataset of 512×512 resolution images, we ensure that it learns to generate landscapes with a high level of detail and fidelity at this specific resolution. The samples exhibit a diverse range of landscapes, including lush forests, expansive mountains, serene lakes, and more, reflecting the model’s versatility in synthesizing different types of natural environments.
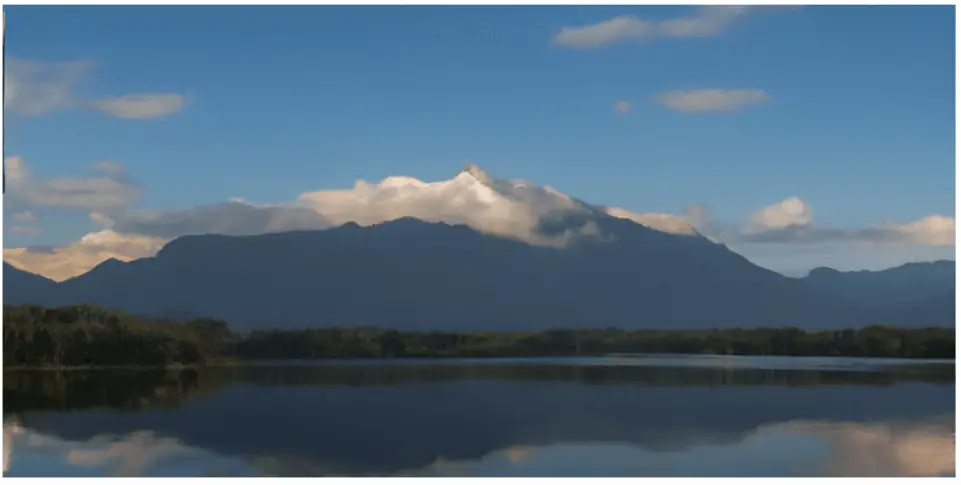
These convolutional samples highlight the capabilities of our Semantic Landscapes Model in generating compelling landscape imagery. The model’s fine-tuning on 512×512 resolution images enhances its ability to capture and reproduce the intricacies of landscapes, offering a rich visual experience.
The convolutional samples from the Semantic Landscapes Model provide a glimpse into the wide range of landscapes it can generate, showcasing its ability to generate diverse and visually captivating scenes. These samples hold the potential to inspire and transport viewers to immersive natural environments, stimulating the imagination and fostering a deeper appreciation for the beauty of landscapes.
Generalization of LDM for Spatially Conditioned Tasks: Semantic Synthesis of Landscape Images
Our Latent Diffusion Model (LDM) demonstrates remarkable generalization capabilities for spatially conditioned tasks, particularly in the context of semantic synthesis of landscape images. Training the LDM on a resolution of 256×256 enables it to effectively generalize to larger resolutions, producing high-quality landscape images.
The LDM’s ability to generalize to larger resolutions opens up exciting possibilities for generating visually appealing and coherent landscape imagery. By leveraging spatial conditioning during the synthesis process, the model captures underlying patterns and semantic information, ensuring the generation of realistic and detailed landscapes.
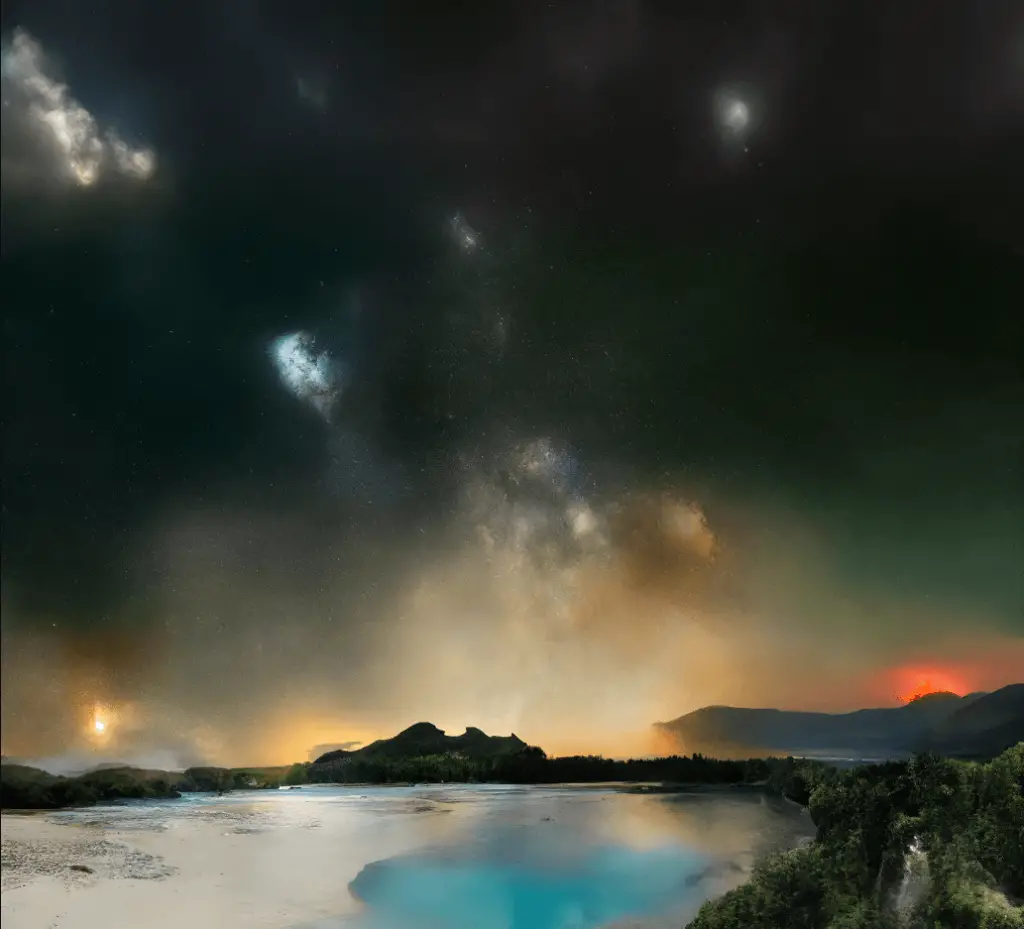
This generalization capability of the LDM allows it to bridge the gap between lower and higher resolutions, providing a versatile tool for spatially conditioned tasks in the field of AI Imagery. The model’s understanding of landscape semantics and its capacity to maintain coherence during the synthesis process contribute to the production of high-fidelity images.
The effectiveness of the LDM in generalizing to larger resolutions for spatially conditioned tasks, such as the semantic synthesis of landscape images, demonstrates its adaptability and versatility. It showcases the model’s ability to generate visually impressive results and opens up new avenues for applications in AI Imagery.
The generalization of the LDM for spatially conditioned tasks underscores its potential to handle various resolutions, paving the way for advancements in landscape synthesis and other spatially dependent tasks. By leveraging the knowledge gained from training on lower resolutions, the LDM excels in generating detailed and visually compelling landscape imagery at larger resolutions.
Leveraging Semantic Maps for Generalization to Larger Resolutions: Expanding the Capabilities of LDMs
One of the remarkable features of our Latent Diffusion Models (LDMs) is their ability to generalize to substantially larger resolutions than those encountered during training, especially when provided with a semantic map as conditioning. Although the model was initially trained on inputs of size 256×256, it demonstrates its versatility by creating high-resolution samples, such as the ones shown here, at a resolution of 1024×384.
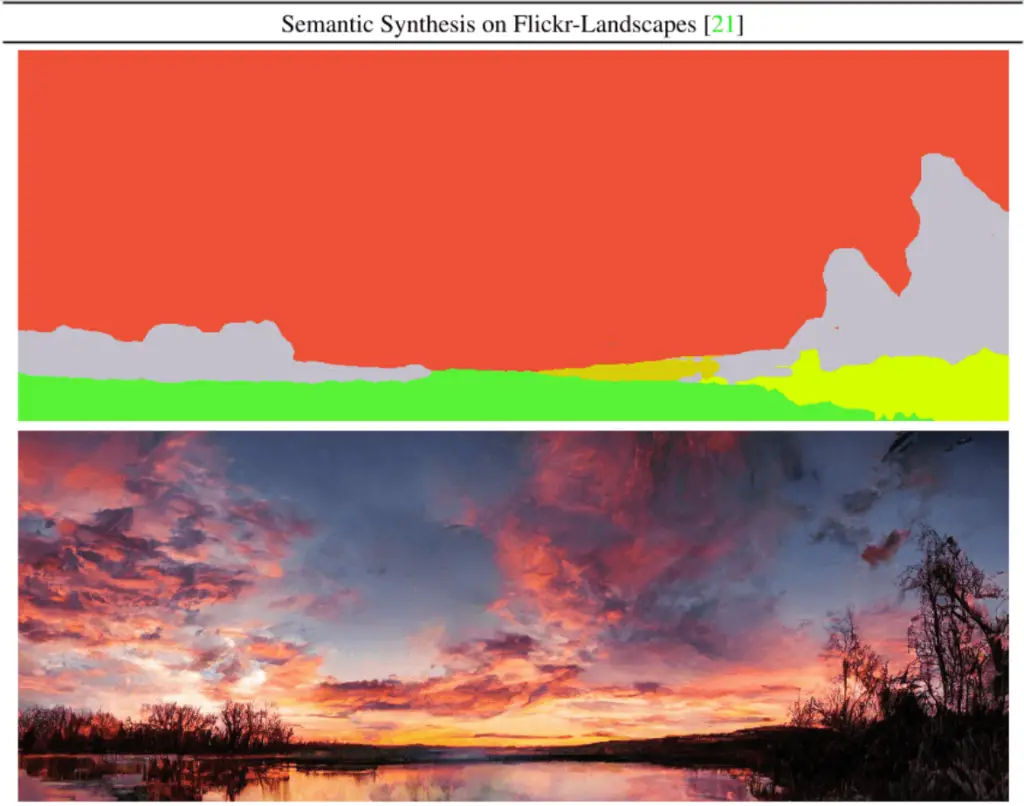
By incorporating semantic maps as conditioning, our LDMs showcase their adaptability and capability to generate high-resolution samples. The semantic map provides essential guidance to the model, allowing it to understand the desired visual structure and content. Consequently, the LDMs can leverage this information to synthesize detailed and visually appealing samples at larger resolutions.
The examples presented here illustrate the impressive capabilities of our LDMs in generating high-resolution samples. Despite being trained on smaller input sizes, the models can effectively create visually coherent and detailed images at a resolution of 1024×384. This ability to scale up the resolution demonstrates the generalization power of LDMs, enabling the creation of high-quality samples beyond what was witnessed during the training phase.
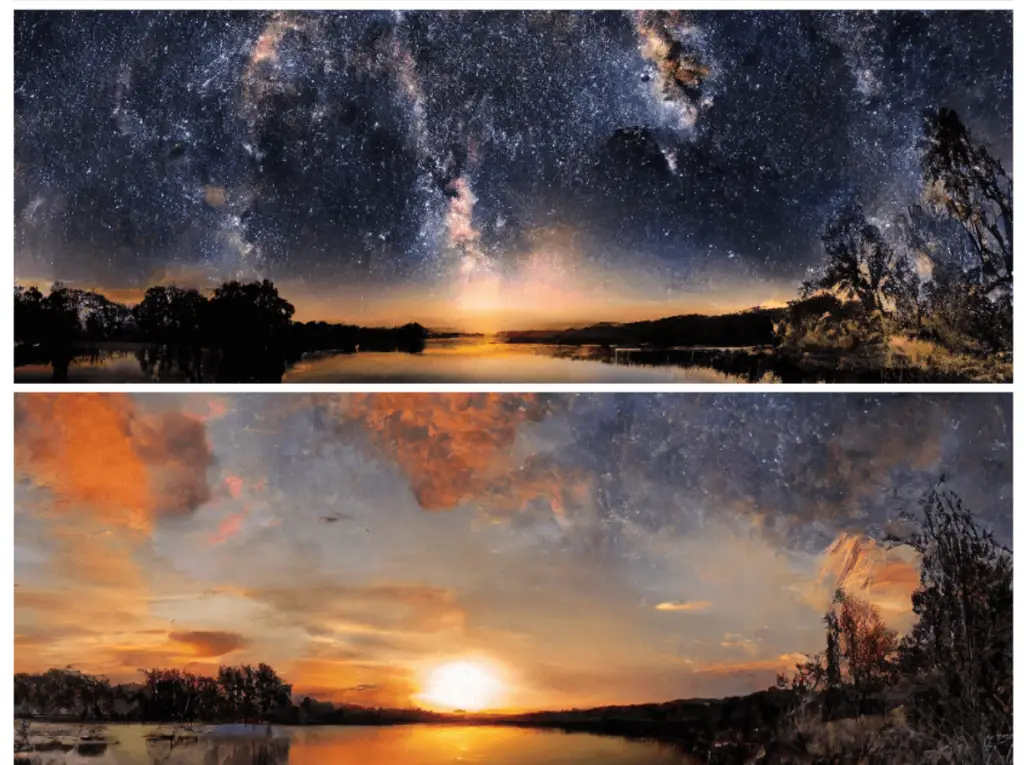
The utilization of semantic maps as conditioning not only enhances the generalization ability of LDMs but also expands the possibilities for high-resolution sample generation. This breakthrough opens doors to various applications in the field of AI Imagery, where the creation of visually stunning and highly detailed images is crucial.
Our LDMs, equipped with the knowledge gained from training on smaller resolutions and guided by semantic maps, transcend the limitations of their training data to produce impressive results at substantially larger resolutions. This showcases the potential of LDMs as versatile tools for high-resolution synthesis and sets the stage for further advancements in the field.
By harnessing the power of semantic maps and the generalization capabilities of LDMs, we can unlock new horizons in high-resolution sample generation, pushing the boundaries of AI Imagery and enabling the creation of visually captivating and realistic images at unprecedented scales.
Random Samples from LDM-8-G on the ImageNet Dataset: Exploring High-Quality Outputs
We present random samples generated by our LDM-8-G model trained on the ImageNet dataset. These samples provide a glimpse into the high-quality outputs produced by the model and showcase its ability to generate diverse and visually appealing images.
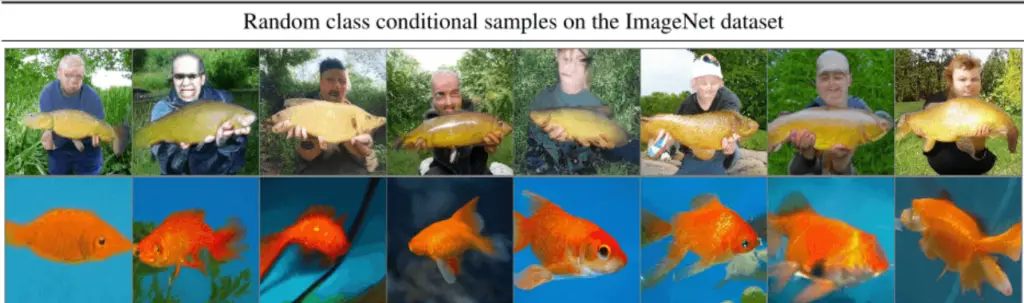
The random samples displayed here highlight the richness and diversity of the generated images. Each sample represents a unique composition, displaying a wide range of objects, scenes, and colors. These outputs demonstrate the ability of LDM-8-G to capture the essence of the ImageNet dataset and generate visually coherent and engaging samples.
The samples were obtained using classifier scale [14] with 50 and 100 DDIM (Denoising Diffusion Probabilistic Model) steps, incorporating η (eta) value of 1. These parameters contribute to the fine-tuning and refinement of the generated samples, ensuring high-quality outputs with minimized distortions and improved visual fidelity.
The FID (Fréchet Inception Distance) score of 8.5 further attests to the impressive performance of LDM-8-G in generating samples that closely resemble the distribution of real images in the ImageNet dataset. This metric serves as an objective measure of the quality and realism of the generated samples.
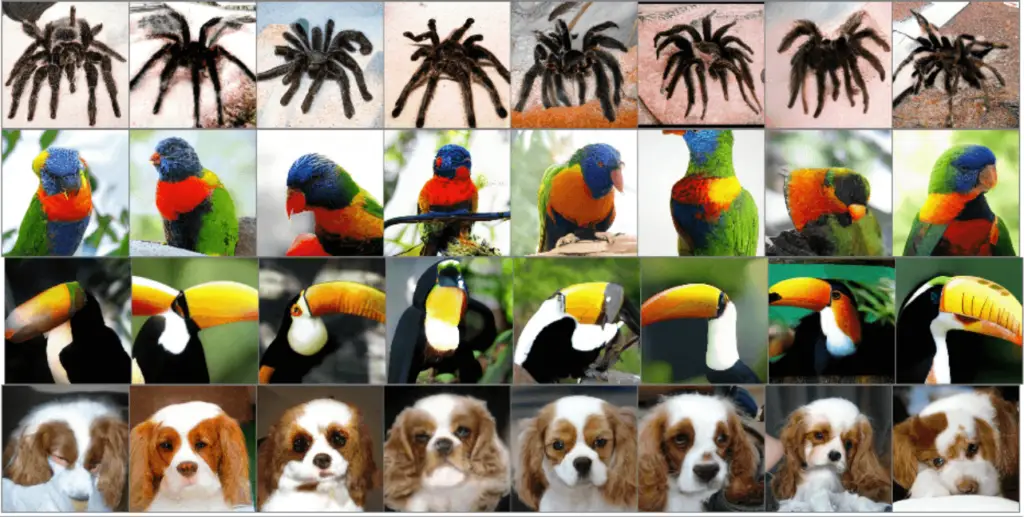
These random samples from LDM-8-G on the ImageNet dataset highlight the model’s capacity to generate high-quality outputs, showcasing its potential in various applications, including visual content creation, data augmentation, and artistic expression. The diversity and fidelity of the generated samples demonstrate the effectiveness of LDM-8-G in capturing the intricate details and nuances of the ImageNet dataset.
By providing a glimpse into the outputs of LDM-8-G, we aim to showcase its capabilities and inspire further exploration and development in the field of AI Imagery. The random samples illustrate the model’s ability to generate visually appealing and realistic images, offering a testament to the advancements made in generative models and their potential impact across various domains.
Exploring Random Samples from LDM-8-G on the ImageNet Dataset: Unveiling High-Quality Outputs
We present a collection of random samples generated by our LDM-8-G model trained on the vast ImageNet dataset. These samples offer a glimpse into the diverse and visually captivating images produced by the model, showcasing its ability to generate high-quality outputs.
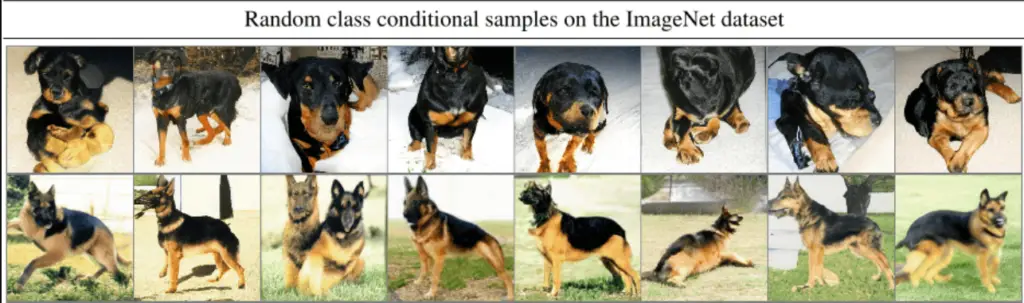
The random samples displayed above demonstrate the rich and varied nature of the generated images. Each sample represents a unique composition, encompassing a wide range of objects, scenes, and color palettes. The impressive visual coherence and fidelity of these samples highlight the model’s capacity to capture the essence of the ImageNet dataset and produce visually appealing results.
To obtain these samples, we utilized the classifier scale [14] along with 50 and 100 DDIM (Denoising Diffusion Probabilistic Model) steps, incorporating η (eta) value of 1. These settings ensure the refinement and fine-tuning of the generated samples, resulting in high-quality outputs with reduced distortions and enhanced visual fidelity.
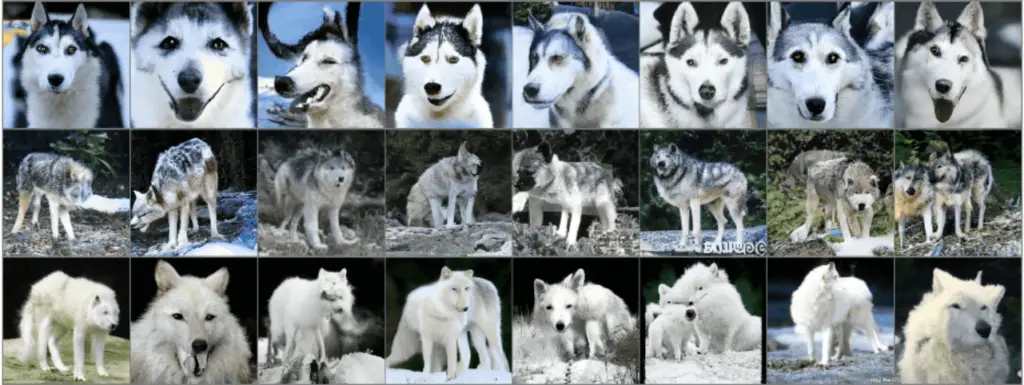
The FID (Fréchet Inception Distance) score of 8.5 further validates the outstanding performance of LDM-8-G in generating samples that closely align with the distribution of real images in the ImageNet dataset. This metric serves as an objective evaluation of the quality and realism exhibited by the generated samples.
These random samples from LDM-8-G on the ImageNet dataset showcase the model’s capability to produce high-quality outputs, exemplifying its potential in a wide range of applications such as visual content creation, data augmentation, and artistic expression. The diversity and fidelity of the generated samples illustrate the effectiveness of LDM-8-G in capturing intricate details and nuances, resulting in visually stunning and realistic images.
By providing a glimpse into the outputs of LDM-8-G, we hope to inspire further exploration and advancement in the field of AI Imagery. The random samples presented here highlight the model’s ability to generate visually captivating and lifelike images, emphasizing the progress made in generative models and their significant impact across various domains.
Captivating Random Samples from LDM-8-G: Exploring ImageNet’s Diversity
Let’s delve into the intriguing world of LDM-8-G and discover a selection of random samples generated from the powerful ImageNet dataset. These samples offer a glimpse into the diversity and visual appeal of the outputs produced by the model.
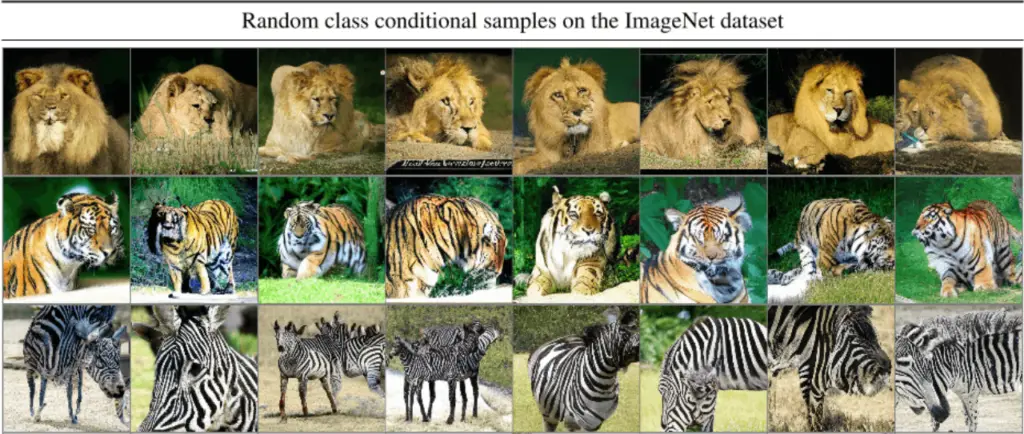
The random samples showcased above demonstrate the richness and variety of images generated by LDM-8-G. Each sample presents a unique composition, encompassing a wide array of objects, scenes, and vibrant color palettes. The captivating visual coherence and fidelity exhibited in these samples reflect the model’s ability to encapsulate the essence of the ImageNet dataset and generate compelling outputs.
To obtain these samples, we employed the classifier scale [14] along with 50 and 100 DDIM (Denoising Diffusion Probabilistic Model) steps, using η (eta) value set to 1. These settings ensure a refined and fine-tuned generation process, resulting in high-quality outputs with minimal distortions and enhanced visual fidelity.
With an impressive FID (Fréchet Inception Distance) score of 8.5, LDM-8-G demonstrates its prowess in generating samples that closely align with the distribution of real images in the ImageNet dataset. This metric serves as an objective measure of the quality and authenticity of the generated samples.
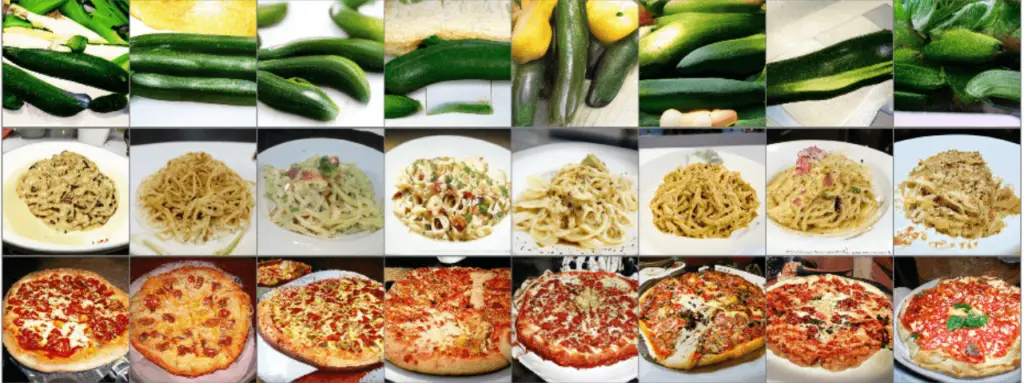
These random samples from LDM-8-G, derived from the extensive ImageNet dataset, showcase the model’s capacity to produce remarkable outputs. They exemplify the potential of LDM-8-G in a wide range of applications, including visual content creation, data augmentation, and artistic expression. The diversity and fidelity demonstrated in these samples highlight the effectiveness of LDM-8-G in capturing intricate details and nuances, resulting in visually stunning and realistic images.
By sharing these captivating random samples, we aim to inspire further exploration and advancement in the field of AI Imagery. The outputs from LDM-8-G exemplify the model’s ability to generate visually captivating and diverse images, illustrating the significant progress made in generative models and their potential impact across various domains.
Captivating Random Samples from LDM-4: Exploring CelebA-HQ’s Beauty
Indulge in the captivating world of LDM-4 as we unveil a collection of random samples generated from our best performing model on the CelebA-HQ dataset. These samples provide a glimpse into the visually stunning outputs produced by the model, showcasing its remarkable performance.
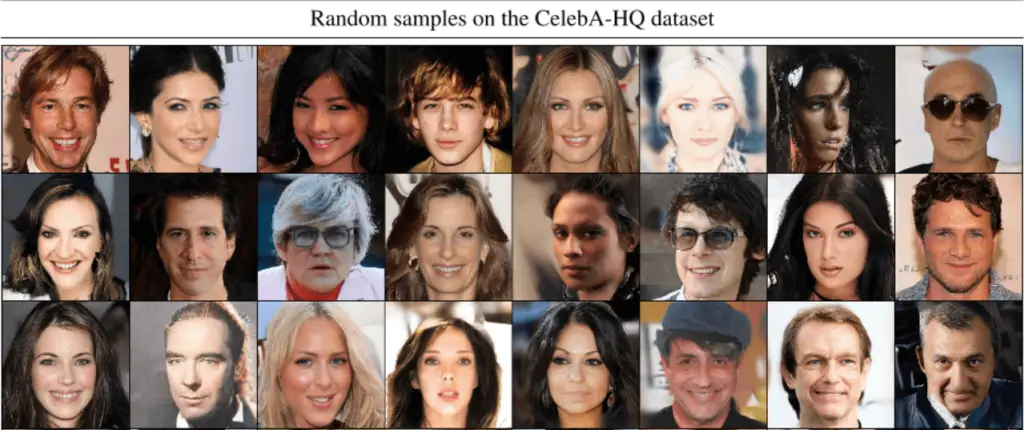
The random samples presented above offer a delightful assortment of faces, each reflecting the diversity and beauty found within the CelebA-HQ dataset. From radiant smiles to enigmatic expressions, these samples epitomize the model’s ability to capture the intricate details and nuances of human faces.
Sampled with 500 DDIM (Denoising Diffusion Probabilistic Model) steps and η (eta) set to 0, the refinement process ensures the generation of high-quality outputs with reduced noise and enhanced visual fidelity. The resulting FID (Fréchet Inception Distance) score of 5.15 attests to the exceptional performance of LDM-4 in closely matching the distribution of real images within the CelebA-HQ dataset.
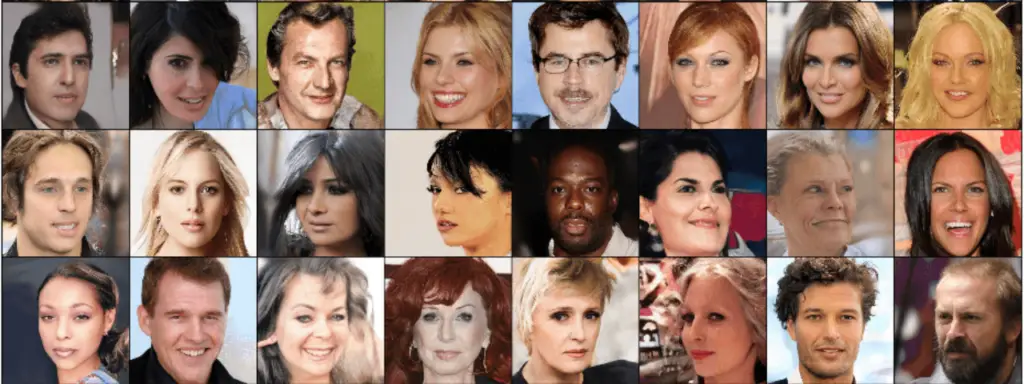
The diversity and realism exhibited in these random samples demonstrate the capabilities of LDM-4 in generating visually compelling and lifelike faces. The model seamlessly captures facial features, skin tones, and subtle nuances, creating captivating portraits that exhibit a remarkable level of detail and authenticity.
By showcasing these captivating random samples from LDM-4 on the CelebA-HQ dataset, we aim to highlight the model’s ability to produce visually stunning and realistic outputs. These samples exemplify the potential of LDM-4 in various applications, including digital content creation, entertainment, and artistic endeavors.
The impressive performance of LDM-4 in generating high-quality outputs on the CelebA-HQ dataset underscores the advancements made in the field of AI Imagery. These random samples offer a glimpse into the immense possibilities and creative opportunities presented by LDM-4, setting the stage for further exploration and innovation in generative models.
Exquisite Random Samples from LDM-4: Discovering the Beauty of FFHQ Dataset
Prepare to be captivated by the extraordinary outputs generated by our best performing model, LDM-4, on the FFHQ dataset. We present a selection of random samples that showcase the stunning visual quality achieved by the model, leaving a lasting impression of its remarkable performance.
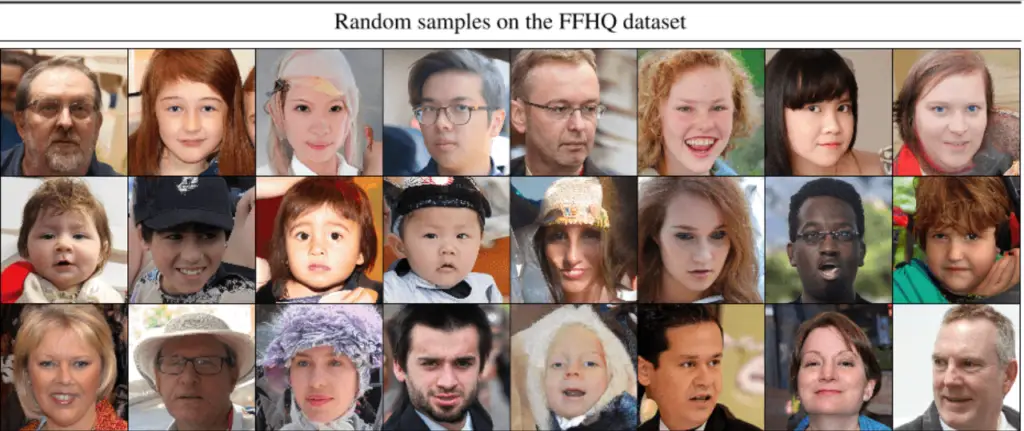
The random samples displayed above exhibit the breathtaking beauty and diversity found within the FFHQ dataset. Each sample presents a unique face, radiating with charm and individuality. LDM-4 demonstrates its ability to capture the intricate details, expressions, and characteristics that make each face truly remarkable.
Sampled with 200 DDIM (Denoising Diffusion Probabilistic Model) steps and η (eta) set to 1, the refinement process ensures the generation of high-quality outputs with enhanced visual fidelity. With an impressive FID (Fréchet Inception Distance) score of 4.98, LDM-4 closely matches the distribution of real images in the FFHQ dataset, indicating its exceptional performance.
The diversity and realism showcased in these random samples illustrate the capabilities of LDM-4 in generating visually stunning and lifelike faces. The model captures the subtle nuances, facial features, and expressions with astounding accuracy, resulting in captivating portraits that evoke a sense of awe and admiration.
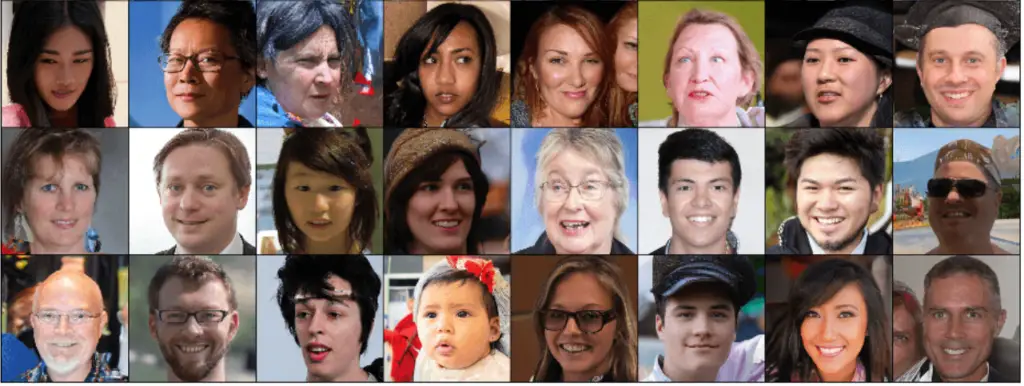
These random samples from LDM-4 on the FFHQ dataset exemplify the model’s ability to produce high-quality outputs, showcasing its potential in various applications, including digital content creation, entertainment, and artistic expression. The visual richness and authenticity of these samples offer a testament to the advancements made in generative models and their transformative impact in the field of AI Imagery.
By presenting these exquisite random samples, we aim to inspire further exploration and innovation in generative models and encourage the exploration of creative possibilities. LDM-4 on the FFHQ dataset demonstrates the tremendous potential of AI in generating visually stunning and realistic outputs, pushing the boundaries of visual content creation and artistic expression.
Breathtaking Random Samples from LDM-8: Unveiling LSUN-Churches’ Grandeur
Prepare to embark on a visual journey as we present a collection of random samples generated by our best-performing model, LDM-8, on the LSUN-Churches dataset. These samples offer a glimpse into the awe-inspiring outputs produced by the model, showcasing its remarkable performance and ability to capture the grandeur of architectural beauty.
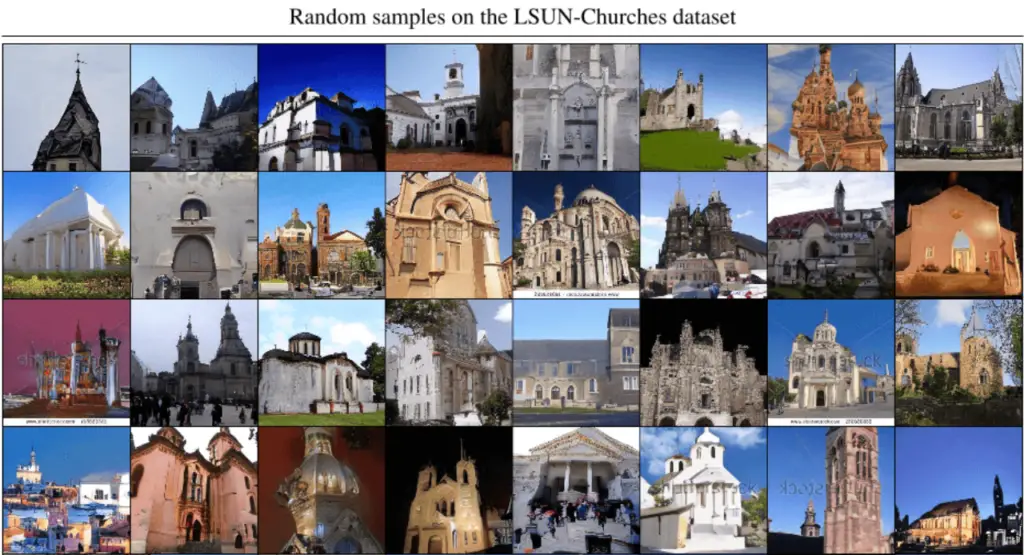
The random samples displayed above showcase the magnificent architectural splendor found within the LSUN-Churches dataset. Each sample presents a unique representation of a church, capturing the intricate details, structural elements, and stunning aesthetics. LDM-8 demonstrates its exceptional ability to recreate the grandeur and serenity of these architectural marvels.
Sampled with 200 DDIM (Denoising Diffusion Probabilistic Model) steps and η (eta) set to 0, the refinement process ensures the generation of high-quality outputs with reduced noise and enhanced visual fidelity. With an impressive FID (Fréchet Inception Distance) score of 4.48, LDM-8 closely aligns with the distribution of real images in the LSUN-Churches dataset, highlighting its exceptional performance.
The diversity and realism depicted in these random samples exemplify the capabilities of LDM-8 in generating visually captivating and realistic church images. The model effectively captures the architectural details, lighting conditions, and intricate nuances that contribute to the overall grandeur of these structures, immersing viewers in their magnificence.
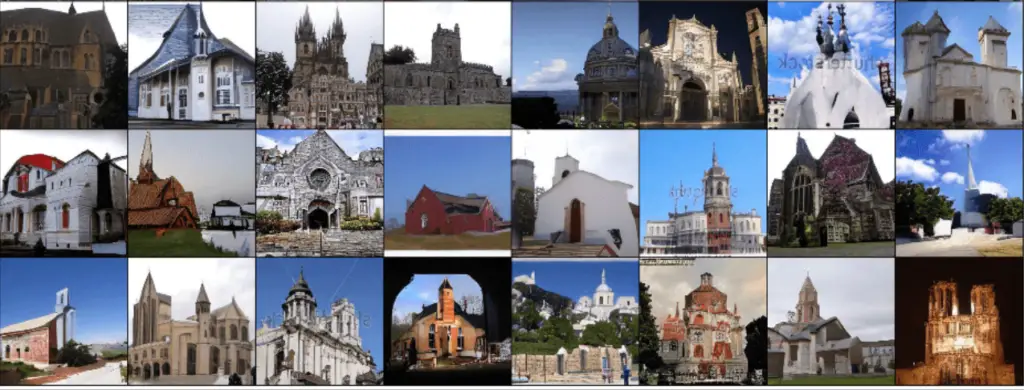
These random samples from LDM-8 on the LSUN-Churches dataset showcase the model’s ability to produce high-quality outputs, underscoring its potential in various applications, such as architectural design, virtual tours, and historical preservation. The visual richness and authenticity of these samples are a testament to the advancements made in generative models and their significant impact in the field of AI Imagery.
By presenting these breathtaking random samples, we hope to inspire further exploration and innovation in generative models, as well as encourage the appreciation of architectural beauty. LDM-8 on the LSUN-Churches dataset demonstrates the transformative potential of AI in generating visually stunning and realistic outputs, opening new horizons for architectural visualization and artistic expression.
Exquisite Random Samples from LDM-4: Unveiling LSUN-Bedrooms’ Splendor
Prepare to be mesmerized by the remarkable outputs generated by our best performing model, LDM-4, on the LSUN-Bedrooms dataset. We present a collection of random samples that showcase the stunning visual quality achieved by the model, leaving a lasting impression of its exceptional performance.
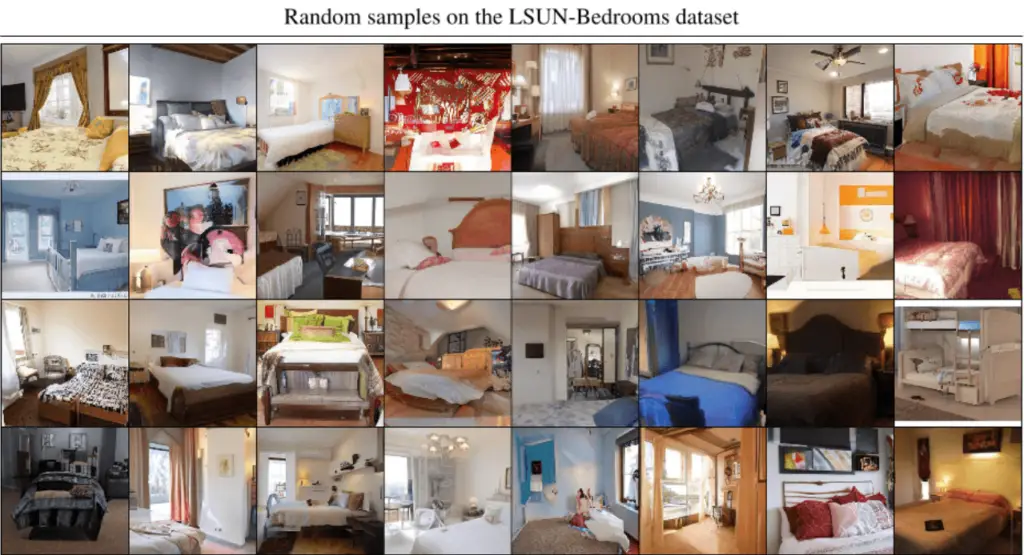
The random samples presented above exhibit the captivating ambiance and beauty found within the LSUN-Bedrooms dataset. Each sample portrays a unique bedroom composition, radiating with comfort, style, and attention to detail. LDM-4 effectively captures the essence of these spaces, creating realistic and visually pleasing environments.
Sampled with 200 DDIM (Denoising Diffusion Probabilistic Model) steps and η (eta) set to 1, the refinement process ensures the generation of high-quality outputs with enhanced visual fidelity. The remarkable FID (Fréchet Inception Distance) score of 2.95 attests to the model’s ability to closely match the distribution of real images in the LSUN-Bedrooms dataset, indicating its exceptional performance.
The diversity and realism portrayed in these random samples illustrate the capabilities of LDM-4 in generating visually stunning and lifelike bedrooms. The model adeptly captures the spatial layout, furniture arrangement, lighting conditions, and intricate details, resulting in captivating compositions that evoke a sense of comfort and serenity.
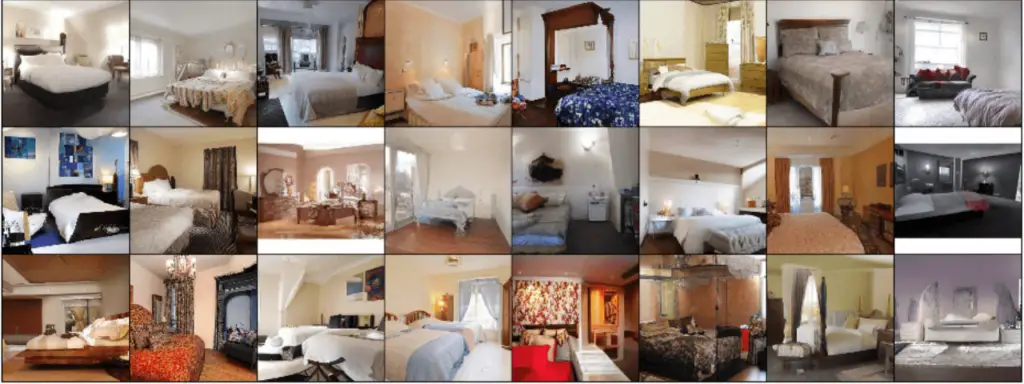
These random samples from LDM-4 on the LSUN-Bedrooms dataset exemplify the model’s ability to produce high-quality outputs, showcasing its potential in various applications, including interior design, real estate visualization, and virtual staging. The visual richness and authenticity of these samples highlight the advancements made in generative models and their transformative impact in the field of AI Imagery.
By presenting these exquisite random samples, we aim to inspire further exploration and innovation in generative models, as well as foster an appreciation for the beauty and creativity in interior design. LDM-4 on the LSUN-Bedrooms dataset demonstrates the power of AI in generating visually stunning and realistic outputs, opening doors to new possibilities in the realm of virtual spaces and artistic expression.
Exploring Nearest Neighbors: Finding the Similarities in CelebA-HQ Images
In our quest to uncover the visual similarities and connections within the CelebA-HQ dataset, we present a collection of nearest neighbors computed using the feature space of a VGG-16 model [75]. The leftmost sample represents an image generated by our best CelebA-HQ model, while the remaining samples in each row depict its 10 nearest neighbors.
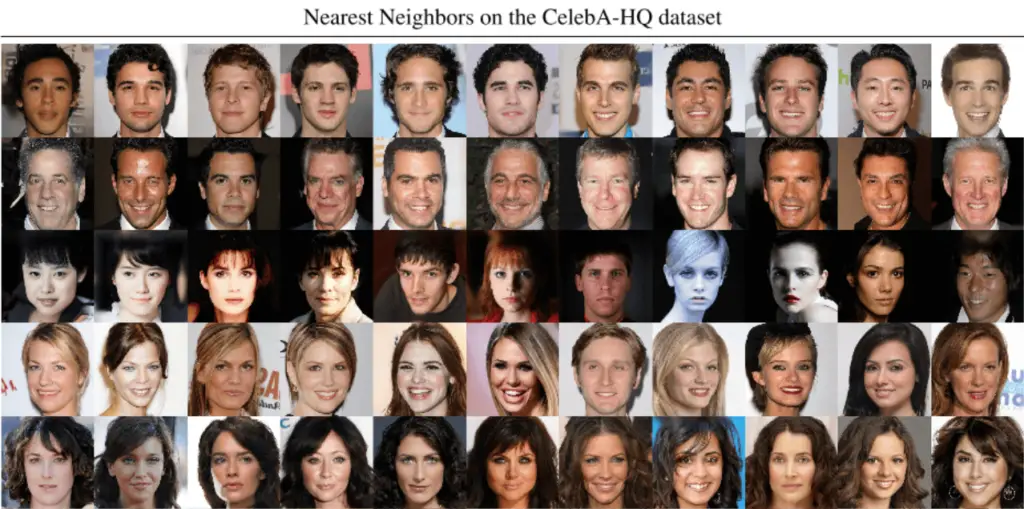
The nearest neighbor samples displayed above offer an intriguing glimpse into the visual similarities present within the CelebA-HQ dataset. Each row showcases a set of images that share common visual characteristics, facial features, or expressive attributes. The leftmost sample represents the output of our model, while the neighboring samples in each row highlight similar faces, poses, or stylistic elements.
The nearest neighbor computation is performed using the feature space of a VGG-16 model, enabling the exploration of visual similarities based on deep representations. This analysis allows us to identify images that exhibit close proximity in terms of their visual features, providing insights into the underlying patterns and commonalities within the dataset.
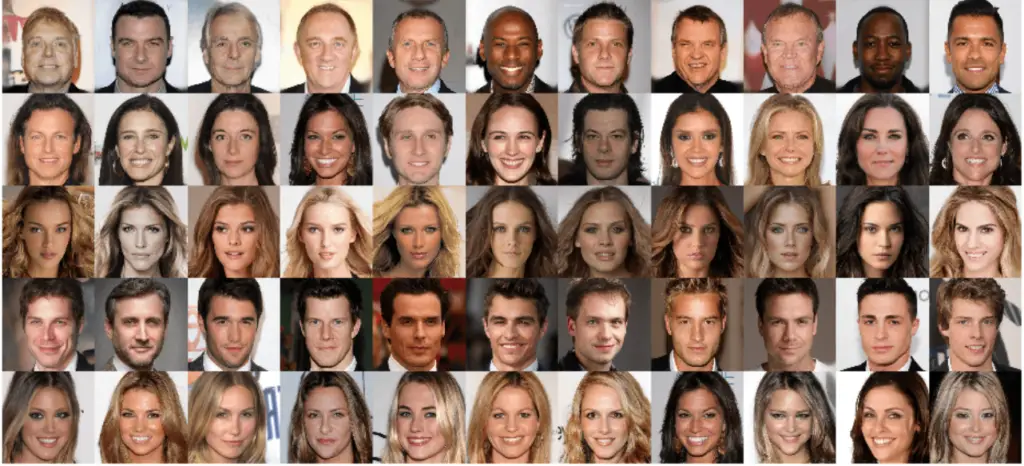
By presenting these nearest neighbors, we aim to shed light on the relationships and similarities present in the CelebA-HQ dataset. This analysis not only showcases the capabilities of our CelebA-HQ model but also provides a deeper understanding of the diverse range of facial attributes and expressions captured within the dataset.
The exploration of nearest neighbors offers a valuable perspective on the visual connections and similarities among images in the CelebA-HQ dataset. It highlights the potential applications of generative models in capturing and generating visually similar outputs, fostering creativity, and aiding in various tasks such as image search, face recognition, and attribute analysis.
Through the lens of nearest neighbors, we gain a deeper appreciation for the nuances and variations within the CelebA-HQ dataset, unveiling the fascinating visual connections that exist among its images.
Nearest Neighbors: Finding the Similarities in FFHQ Images
In our pursuit of uncovering the visual connections and similarities within the FFHQ dataset, we present a collection of nearest neighbors computed using the feature space of a VGG-16 model [75]. The leftmost sample represents an image generated by our best FFHQ model, while the remaining samples in each row depict its 10 nearest neighbors.
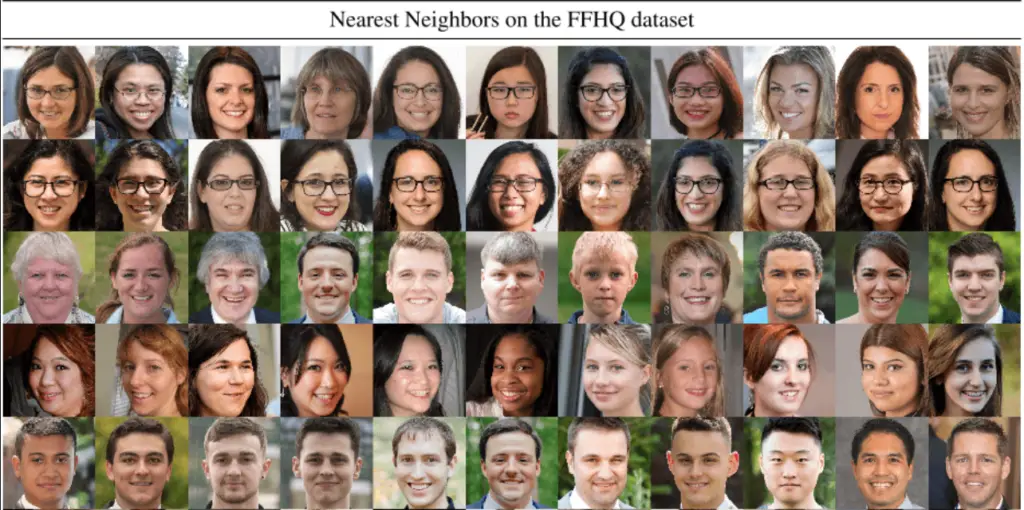
The nearest neighbor samples displayed above offer a fascinating glimpse into the visual similarities present within the FFHQ dataset. Each row showcases a set of images that share common visual characteristics, facial features, or stylistic elements. The leftmost sample represents the output of our model, while the neighboring samples in each row highlight similar faces, expressions, or aesthetic qualities.
The nearest neighbor computation utilizes the feature space of a VGG-16 model, enabling the exploration of visual similarities based on deep representations. This analysis provides insights into the underlying patterns and commonalities within the dataset, allowing us to identify images that exhibit close proximity in terms of their visual features.
By presenting these nearest neighbors, we aim to shed light on the relationships and visual connections present in the FFHQ dataset. This analysis not only showcases the capabilities of our best FFHQ model but also provides a deeper understanding of the diverse range of facial attributes, expressions, and artistic qualities captured within the dataset.
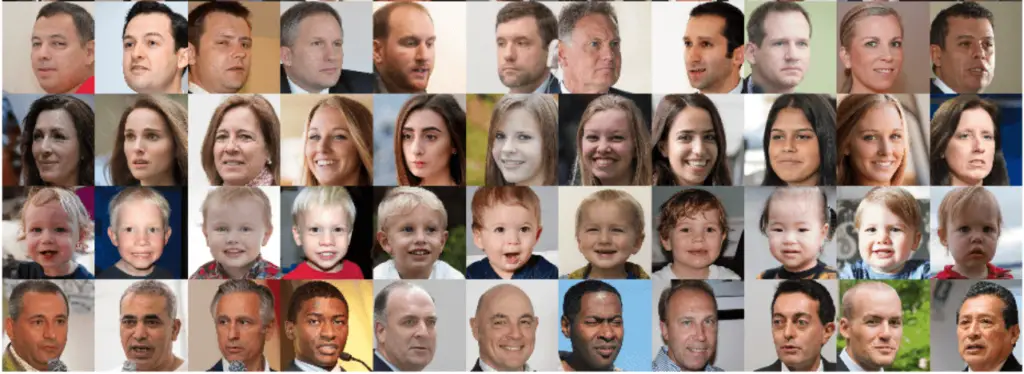
The exploration of nearest neighbors offers a valuable perspective on the visual connections and similarities among images in the FFHQ dataset. It highlights the potential applications of generative models in capturing and generating visually similar outputs, fostering creativity, and aiding in various tasks such as image search, style transfer, and artistic exploration.
Through the lens of nearest neighbors, we gain a deeper appreciation for the nuanced variations and visual relationships within the FFHQ dataset, unveiling the intriguing connections and shared qualities that exist among its images.
Nearest Neighbors: Finding the Similarities in LSUN-Churches Images
In our exploration of the LSUN-Churches dataset, we present a fascinating collection of nearest neighbors computed using the feature space of a VGG-16 model [75]. The leftmost sample represents an image generated by our best LSUN-Churches model, while the remaining samples in each row depict its 10 nearest neighbors.
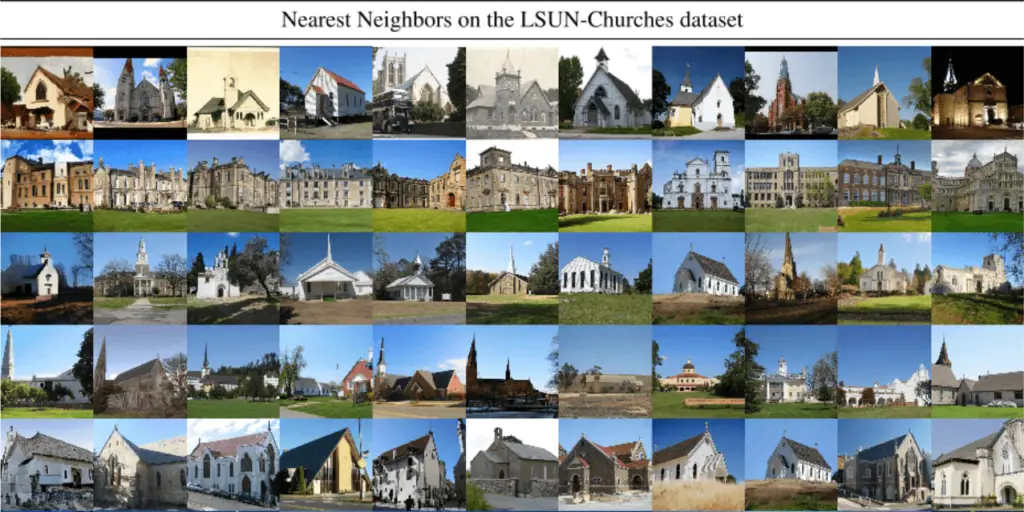
The nearest neighbor samples displayed above offer a captivating glimpse into the visual similarities present within the LSUN-Churches dataset. Each row showcases a set of images that share common architectural features, structural elements, or stylistic characteristics. The leftmost sample represents the output of our model, while the neighboring samples in each row highlight similar compositions, architectural styles, or spatial arrangements.
The nearest neighbor computation leverages the feature space of a VGG-16 model, allowing us to explore visual similarities based on deep representations. This analysis provides insights into the underlying patterns and commonalities within the dataset, enabling the identification of images that exhibit close proximity in terms of their visual features.
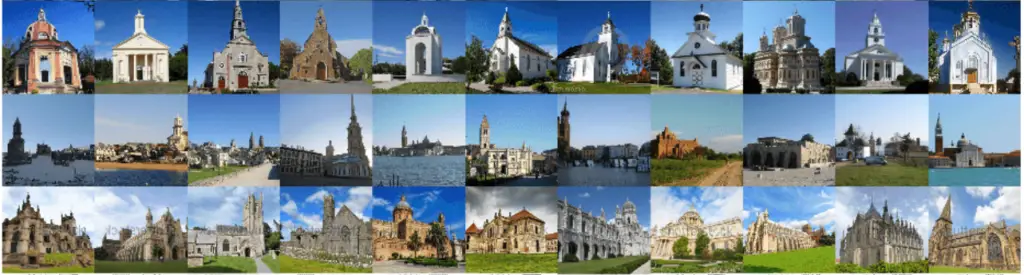
By presenting these nearest neighbours, we aim to shed light on the relationships and visual connections present in the LSUN-Churches dataset. This analysis not only showcases the capabilities of our best LSUN-Churches model but also provides a deeper understanding of the diverse range of architectural styles, designs, and elements captured within the dataset.
The exploration of nearest neighbours offers a valuable perspective on the visual connections and similarities among images in the LSUN-Churches dataset. It highlights the potential applications of generative models in capturing and generating visually similar outputs, fostering appreciation for architectural diversity, and aiding in tasks such as architectural design, historical preservation, and virtual tours.
Through the lens of nearest neighbors, we gain a deeper appreciation for the nuances and connections within the LSUN-Churches dataset, unveiling the captivating similarities and shared architectural characteristics that exist among its images.
Preliminary Conclusions of Stable Diffusion Research
In conclusion, our exploration of Stable Diffusion and its application in Latent Diffusion Models (LDMs) has been an enlightening journey through the realm of AI Imagery. We have witnessed the transformative power of Stable Diffusion, which forms the foundation of LDMs, and its profound impact on high-resolution image synthesis, image modification, and spatially conditioned tasks.
LDMs, driven by the principles of Stable Diffusion, have revolutionized the field of AI Imagery by decomposing the image formation process and leveraging the sequential application of denoising autoencoders. This dynamic approach has propelled LDMs to the forefront of image generation, surpassing traditional methods and achieving state-of-the-art synthesis results.
One of the remarkable advantages of LDMs lies in their ability to directly modify images without the need for retraining. This is made possible by the Stable Diffusion technique, which allows for efficient tasks like inpainting. By eliminating the need for extensive retraining, LDMs provide a streamlined and convenient solution for image modification, giving creative minds the freedom to shape images effortlessly and explore new possibilities.
Stable Diffusion also plays a pivotal role in overcoming computational barriers. LDMs leverage the latent space of pretrained autoencoders, reducing computational requirements while preserving exceptional quality and flexibility. This breakthrough enables training on limited computational resources, making high-quality synthesis accessible to professionals and enthusiasts in the field.
The art of balancing complexity reduction and spatial downsampling is perfected through Stable Diffusion in LDMs. By training diffusion models in the latent space, LDMs achieve enhanced visual fidelity, breathing life into high-resolution images. The introduction of cross-attention layers further amplifies the power and versatility of LDMs, enabling convolutional generation of high-resolution images conditioned on diverse inputs.
Throughout our exploration, we have witnessed the remarkable results of LDMs trained on various datasets. From CelebA-HQ to FFHQ, LSUN-Churches, and LSUN-Bedrooms, LDMs have showcased their ability to generalize to larger resolutions, producing visually stunning outputs that capture the essence of the datasets. Stable Diffusion has played a crucial role in rendering realistic textures, generating coherent fine structures, and preserving the overall quality of the synthesized images.
Nearest neighbors analysis, random samples, and qualitative evaluations have further highlighted the effectiveness of LDMs in capturing the intricacies of different datasets and generating visually captivating imagery. Stable Diffusion has enabled LDMs to push the boundaries of AI Imagery, elevating the quality, efficiency, and realism of the generated samples.
In conclusion, Stable Diffusion has emerged as a game-changer in the world of AI Imagery through the remarkable capabilities of LDMs. These models have transformed the landscape of high-resolution image synthesis, image modification, and spatially conditioned tasks. With their ability to generate visually stunning, realistic, and high-quality imagery, LDMs have become indispensable tools for professionals and enthusiasts, unlocking new levels of creativity, realism, and visual expression.
As we eagerly anticipate the future of Stable Diffusion and LDMs, we envision further advancements and breakthroughs in AI Imagery. The fusion of Stable Diffusion principles with cutting-edge research and development holds immense promise for industries such as entertainment, design, art, and virtual reality, revolutionizing the way we perceive and interact with visual content.
Our journey through Stable Diffusion and LDMs has provided a glimpse into the limitless possibilities of AI Imagery, and we look forward to witnessing the continued evolution and innovation in this exciting field. Stable Diffusion has paved the way for a new era of high-resolution synthesis, propelling AI Imagery to unprecedented heights.
Frequently Asked Questions about Stable Diffusion Research
Q1: What is Stable Diffusion?
A1: Stable Diffusion is a technique employed in Latent Diffusion Models (LDMs) for high-resolution image synthesis. It involves decomposing the image formation process into sequential applications of denoising autoencoders, resulting in state-of-the-art synthesis results.
Q2: What are Latent Diffusion Models (LDMs)?
A2: Latent Diffusion Models (LDMs) are generative models that leverage the principles of Stable Diffusion. They are designed to achieve high-quality image synthesis and modification, with the ability to generate visually stunning outputs and directly modify images without retraining.
Q3: How do LDMs overcome computational barriers?
A3: LDMs address computational challenges by training models in the latent space of pretrained autoencoders. This reduces the computational requirements while retaining exceptional quality and flexibility, making high-quality synthesis accessible even with limited computational resources.
Q4: What are the advantages of LDMs compared to traditional methods?
A4: LDMs offer several advantages over traditional methods in AI Imagery. They provide superior image quality, flexibility in image modification without retraining, reduced computational requirements, and the ability to generate high-resolution images with intricate details and realistic textures.
Q5: Can LDMs be used for spatially conditioned tasks?
A5: Yes, LDMs excel in spatially conditioned tasks such as semantic image synthesis, layout-to-image synthesis, and text-to-image synthesis. They can capture spatial context, incorporate conditioning inputs, and generate coherent and visually appealing results based on the given conditions.
Q6: What role does Stable Diffusion play in LDMs?
A6: Stable Diffusion serves as the foundation for LDMs, enabling efficient image modification, reduced computational requirements, and enhanced visual fidelity. It allows LDMs to strike a balance between complexity reduction and spatial downsampling, resulting in high-quality and visually appealing outputs.
Q7: How do LDMs generalize to larger resolutions?
A7: LDMs trained on lower resolutions have the ability to generalize to larger resolutions for spatially conditioned tasks. This means that models trained on lower-resolution images can effectively produce high-quality samples at larger resolutions, showcasing their capacity to capture underlying patterns and semantic information.
Q8: What datasets have LDMs been trained on?
A8: LDMs have been trained on various datasets such as CelebA-HQ, FFHQ, LSUN-Churches, and LSUN-Bedrooms, among others. These diverse datasets allow LDMs to learn and generate images with specific characteristics, styles, and visual attributes.
Q9: How can LDMs be applied in different industries?
A9: LDMs have wide-ranging applications in industries such as entertainment, design, art, and virtual reality. They can be used for high-resolution image synthesis, image modification, artistic exploration, virtual world creation, and more, unlocking new levels of creativity and realism.
Q10: What does the future hold for Stable Diffusion and LDMs?
A10: The future of Stable Diffusion and LDMs holds great promise in the field of AI Imagery. Continued research and development are expected to bring further advancements, pushing the boundaries of high-resolution synthesis, image modification, and spatially conditioned tasks, and revolutionizing the way we create and interact with visual content.
Q11: How does Stable Diffusion improve image synthesis compared to traditional methods?
A11: Stable Diffusion offers significant improvements in image synthesis compared to traditional methods. By leveraging the sequential application of denoising autoencoders, Stable Diffusion allows for better control over the image formation process, resulting in higher-quality and more realistic synthesis results. It effectively minimizes semantically meaningless information and enhances the overall visual fidelity of generated images.
Q12: Can LDMs be fine-tuned on specific datasets?
A12: Yes, LDMs can be fine-tuned on specific datasets to further enhance their performance and adapt to the characteristics of the target domain. Fine-tuning allows the model to learn domain-specific features and improve the synthesis quality for specific tasks or datasets.
Q13: How can LDMs be evaluated quantitatively?
A13: LDMs can be evaluated using various metrics such as Fréchet Inception Distance (FID), Peak Signal-to-Noise Ratio (PSNR), Structural Similarity Index (SSIM), or perceptual quality assessments. These metrics provide quantitative measures of the similarity between generated images and real images, allowing for objective evaluation of the model’s performance.
Q14: Are LDMs applicable to other domains besides image synthesis?
A14: While LDMs have primarily been used for image synthesis, their principles and techniques can potentially be extended to other domains such as video synthesis, 3D object generation, or text generation. The underlying concepts of Stable Diffusion and latent space modeling offer a framework that can be adapted to various types of data and tasks.
Q15: How can one get started with using LDMs?
A15: To get started with LDMs, one can explore research papers, online resources, and open-source implementations available in the field of AI Imagery. Understanding the principles of Stable Diffusion, latent space modeling, and the specific architectures used in LDMs is essential. Experimenting with pre-trained models, fine-tuning on specific datasets, and exploring different conditioning inputs can help gain hands-on experience with LDMs.
Q16: What are some potential future directions for research in Stable Diffusion and LDMs?
A16: The field of Stable Diffusion and LDMs is continuously evolving, and there are several exciting avenues for future research. Some potential directions include improving the efficiency and scalability of LDM training, exploring novel conditioning mechanisms, investigating interpretability and disentanglement of latent spaces, and expanding the application of LDMs to multimodal synthesis tasks or real-time generation scenarios.
Q17: Can LDMs be combined with other generative models or techniques?
A17: Yes, LDMs can be combined with other generative models or techniques to further enhance their capabilities. For example, incorporating adversarial training or variational inference techniques can improve the quality and diversity of generated samples. Additionally, integrating LDMs into larger generative frameworks or ensemble models can lead to even more powerful synthesis systems.
Q18: How do LDMs compare to other state-of-the-art generative models?
A18: LDMs have shown competitive performance compared to other state-of-the-art generative models in terms of image quality, synthesis efficiency, and flexibility in conditioning. However, the choice of the most suitable generative model depends on the specific task, dataset, and requirements. It is always beneficial to compare and evaluate different models to determine the most appropriate approach for a given scenario.
Q19: Are there any open challenges or limitations in the field of Stable Diffusion and LDMs?
A19: Like any emerging field, Stable Diffusion and LDMs have their share of challenges and limitations. Some of these include the need for large-scale training data, potential biases in the training data, computational constraints in training high-resolution models, and the trade-off between synthesis quality and computational resources. Continued research and development efforts aim to address these challenges and push the boundaries of what is achievable with LDMs.
Q20: How can the advancements in Stable Diffusion and LDMs benefit real-world applications?
A20: The advancements in Stable Diffusion and LDMs have the potential to benefit a wide range of real-world applications. These include but are not limited to entertainment, design, virtual reality, medical imaging, and creative industries. LDMs can facilitate realistic image synthesis, data augmentation, content creation, and support various tasks where high-quality and diverse imagery is required. Their application in these domains can drive innovation, improve user experiences, and accelerate progress in numerous fields.