In the realm of image processing and analysis, advancements in diffuse methodologies have given rise to a fascinating cross-pollination of mathematical principles and visual transformations. Specifically, stable diffusion processes have proven to be of considerable worth in the context of image-to-image transformations.
This cutting-edge technology enables finer and more precise modifications of images, paving the way for nuanced visual narratives that are dynamic and engrossing. At its core, the science of stable diffusion img2img revolves around meticulous extraction of image properties, skillful manipulation of these properties via model training and predictive approaches, as well as continual refinement of these models for the sake of stability and consistency. This essay delves into the foundations, implementations, challenges, and future prospects of stable diffusion in image processing.
Contents
Foundations and Principles of Stable Diffusion
Unraveling the Core Principles and Foundations of Stable Diffusion: An In-Depth Examination
In the intricate world of molecular movement, the concept of diffusion reigns supreme, holding a significance that resonates from the corridors of our everyday life straight to high-level scientific research and technological advancements. As resolute pursuers of scientific enlightenment, it becomes vital to dissect and interpret the fundamental principles and foundational aspects of stable diffusion, an oft-underrated, but critical aspect of this dynamic phenomenon.
Possessing the mind of an explorer, let us delve into understanding stable diffusion. At the most elementary level, stable diffusion refers to the tendency of particles to distribute themselves evenly within a given system. This involves a naturally occurring migration from regions of high concentration to those of lower concentration, creating a statistical equilibrium. In essence, it is the choreographer of a delicate molecular dance where randomness reigns, yet stability ensues.
The principals underlying stable diffusion are rooted in the laws of statistical mechanics. Foremost, Fick’s First Law of Diffusion is a driving force. It outlines a linear relationship between the instantaneous rate of diffusion (flux) and the concentration gradient, ruled by the intrinsic property of the material, the diffusion coefficient. This law captures the core of diffusion, effectively outlining how particles map out their paths through space and time.
On a foundation of thermodynamics, diffusion is seen as the net result of a series of random movements. Certain particles may travel primarily in one direction, while others may move in an entirely different route. However, despite the seemingly chaotic orientation, these irregular paths lend themselves to the regularity of diffusion, leading to a stable condition.
This stably diffusive state underscores another imperative principle: dynamic equilibrium. When interpreting diffusion, some might mistakenly perceive it as an end goal rather than an ongoing process. In stable diffusion, although the concentration of particles may have equalized over time, the movement of said molecules does not halt. Rather, it continues invisibly and ceaselessly, maintaining the lines of the equilibrium and fostering stability.
Inherent randomness or Brownian motion further solidifies the foundation of stable diffusion. In this context, it is important to appreciate the stochastic nature of particle movement. Each particle navigates according to its own random walk, independent of its neighbors, but collectively their paths map the bigger picture of the diffusion process.
The principles and antagonistic nature of randomness and stability in diffusion often make a fascinating read. They unveil the harmony that defies chaos in the microscopic realms, shedding light on the paradoxical beauty of nature’s order. While seemingly abstract, these principles have far-reaching implications in various fields, from polymer science and materials engineering to biological processes and technological advancements.
So, an understanding of stable diffusion is indispensable, even if it initially appears elusive due to its sophisticated interplays. It ultimately points towards the relentless truth of nature – an unyielding quest in the journey towards the universal goal – achieving equilibrium.
The exploration of stable diffusion hence promises not just an upgrade in scientific comprehension, but also a glimpse into the inherent magic that guides universe’s numerous macro and microscopic phenomena. A moment of paused reflection on this fundamental yet perplexing principle of diffusion indeed drives home the unified narrative of harmony within the chaos, and stability within the randomness.
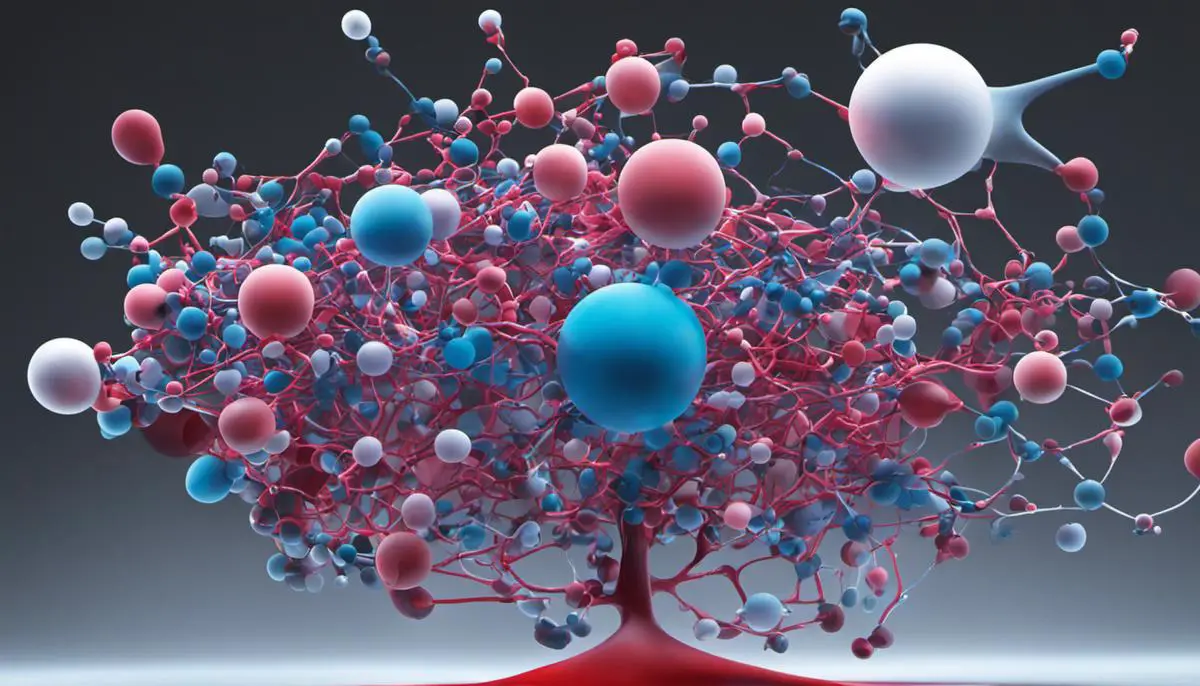
Stable Diffusion Implementation with Image Transformation
As we continue to delve further into the intricacies of stable diffusion, it is crucial to consider its implementation in the nascent yet rapidly burgeoning field of image to image translation.
Here, stable diffusion offers us fresh insights, allowing us to comprehend and interpret the transformations of pixel intensities, colors, and other vital image properties that create narrative and artistic expressions.
Beginning with neural networks, we must understand how these powerful tools can be employed to model the stable diffusion process.
The framework of a neural network, especially the convolutional variant, permits the delineation and examination of the path traversed by random particles within an image, akin to probing the nuances of a real-world diffusion process.
With stochasticity as an inherent element in the design, the implementation of stable diffusion via neural networks becomes a feasible proposition and a potentially transformative tool in image translation tasks.
The volatility and speed of image translation processes often mirror the inherent randomness of stable diffusion.
Thus, a well-calibrated neural network becomes an effective medium to replicate the randomness and stability observed in diffusion processes, through its series of transformational layers.
This structure enables the processing and tracking of changes occurring within the diffusive system, transposing these transformative elements onto the canvas of image to image translation and providing an optimal result.
Crucially, the parameterization of the diffusive process is central to the regulated progression from the input image to the output image.
In this context, diffusion-based neural networks can control the optimization process, ensuring equilibrium in image translation—akin to stable diffusion aiming for dynamic equilibrium—by minimizing the overall energy of the system.
This can be likened to a model’s decision boundaries lowering their potential energy, much like particles moving towards a minimum in reaction-diffusion systems.
Finally, to fully appreciate the mechanism of stable diffusion within image to image translation, mention must be made of the Markov Random Fields (MRF).
The stochastic processes that these fields capture reflect the intrinsically unpredictable aspects of diffusion.
Through MRFs, the spatial dependency and randomness characteristic of diffusion come alive in image translation, bringing an additional layer of sophistication and practicality to the ever-evolving field of computer vision.
Inarguably, the marriage of stable diffusion techniques and image translation promises to be a symbiotic partnership that enriches both fields.
As scientists, while we gauge the stability amidst the randomness, and the equilibrium within the chaos, the objective remains the same: to harness the lessons that stable diffusion offers in presenting a more informed, nuanced, and ultimately transformative approach to image translation—pushing the boundaries of understanding in the burgeoning domain of computer vision.
Undoubtedly, this rendezvous with stable diffusion has only scratched the surface soon, and soon enough, we will steer deeper into the ocean of possibilities that this robust concept presents.
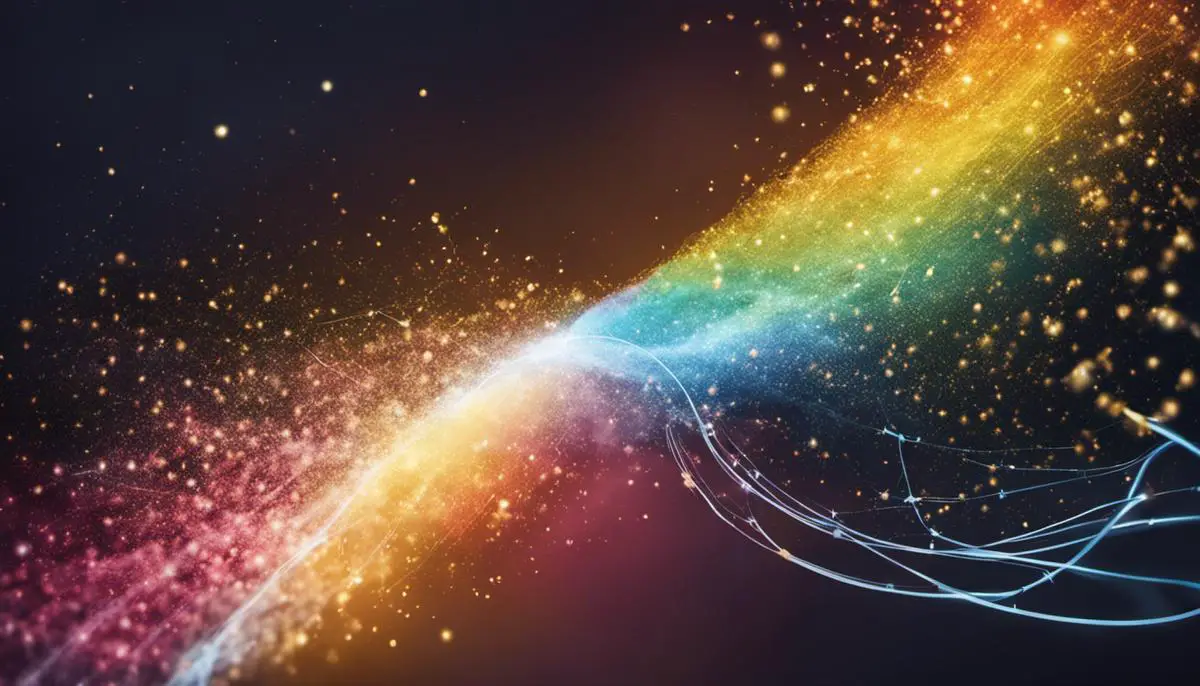
Challenges and Future Prospects in Stable Diffusion img2img
A fitting point of departure to expound on the challenges of stable diffusion within image translation lies in the prominence of image-to-image translation in contemporary research. It has fostered a surge of interest in wielding its potential to glean insights from a myriad of contexts, from healthcare diagnostics to guiding autonomous vehicles. Stable diffusion is a pivotal dynamic that aids in effectively accomplishing this feat. However, the intricacies of implementing stable diffusion in such applications constitute a significant challenge.
Image-to-image transformation requires the incorporation of stable diffusion in a way that effectively maintains the fidelity of original image details while granting the flexibility for image manipulation. The precarious balance between stability and randomness in diffusion complicates this task. An excessive tilt towards either extreme leads to inaccuracies in image transformations. The fine-tuning of this balance presents a challenging conundrum for researchers striving for optimal outcomes.
Further, simulating the inherently random Brownian motion in stable diffusion using artificial neural networks poses another formidable obstacle. Convolutional neural networks, used in large-scale image-to-image translation tasks, habitually encounter hurdles in mirroring the random nature of diffusive processes. Despite their extraordinary neural plasticity, the intricacy of imitating unpredictable, random movements in a controlled environment remains a tough nut to crack.
Transforming models that put the principles of Fick’s First Law into utility is another challenge to be reckoned with. The elusive nature of achieving dynamic equilibrium often curbs the successful execution of image transformations, demanding heavy computational resources and sophisticated algorithms to precisely calibrate the diffusion processes. The task requires complex calibration techniques that undeniably multiply the sophistication of the image translation process.
Notwithstanding the current complexities, the frontier of stable diffusion in image-to-image transformation is poised for groundbreaking advancement. Exploration in Markov Random Fields for unraveling the interplay of stability and randomness in diffusion presents a promising avenue for future research.
Additionally, fine-tuning the parameterization of the diffusive process could yield considerable improvements in the accuracy and efficiency of image translation, pushing the boundaries of current capabilities. Innovations in deep learning offer the prospect of refining the harnessing of stable diffusion, lighting the way for more detailed and precise image transformations.
Further research into neural network models offers vast potential for understanding and successfully mirroring randomness and stability in diffusive processes. This exploration harbors the possibility of outmaneuvering the challenges of calibration and improving the accuracy of image translations.
Ultimately, the marriage of stable diffusion and image-to-image translation points towards a horizon of scientific advancement that will undoubtedly continue to stretch and refine the landscape of modern science. Embracing the challenge and opportunity stable diffusion presents is pivotal to driving future breakthroughs in the field of image translation, and indeed, paints a vibrant picture of scientific accomplishment.
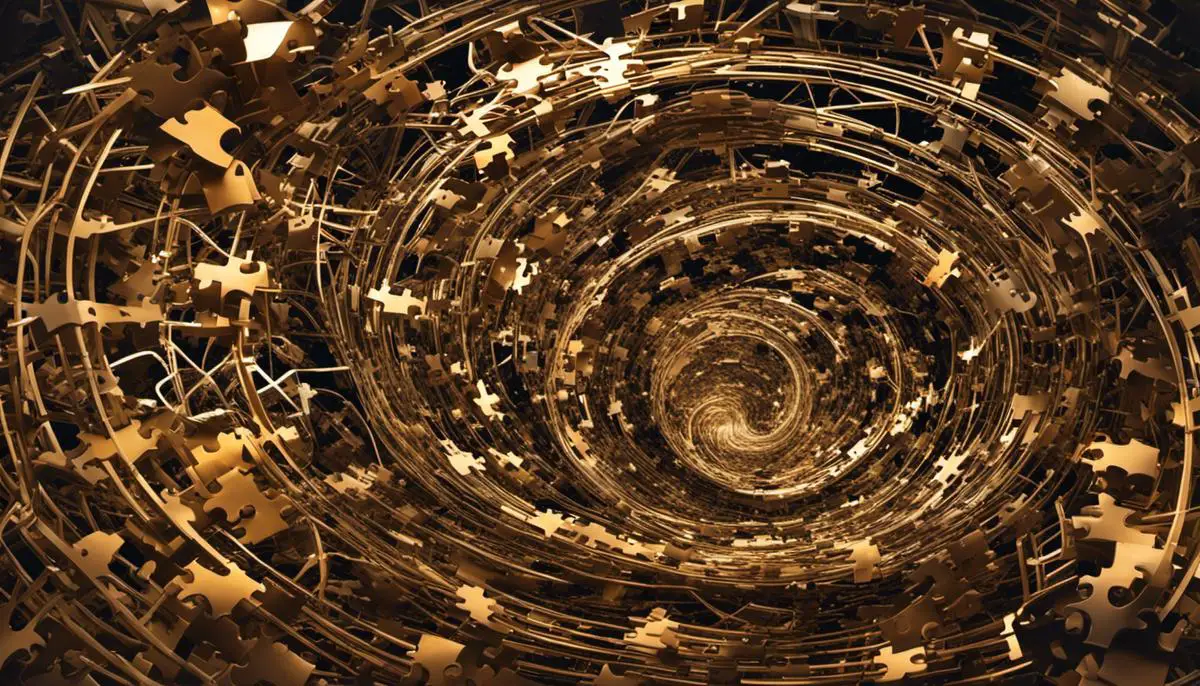
Understanding stable diffusion img2img is like piercing the veil of what is visually possible, allowing us to glimpse the architecture behind intricate transformations of images. Emphasizing on its mathematical underpinnings, this discourse sheds light on the implementation of the technology, the challenges that arise, and how they can be mitigated.
Looking forward, the constant evolution in computational capacities and machine-learning methodologies points to a future where stable diffusion processes could further revolutionize the way we perceive and interact with digital images. As we continue to refine and develop these models, their integration becomes more seamless and their potential applications become more diverse and far-reaching, hinting at an advanced era of visual communication.
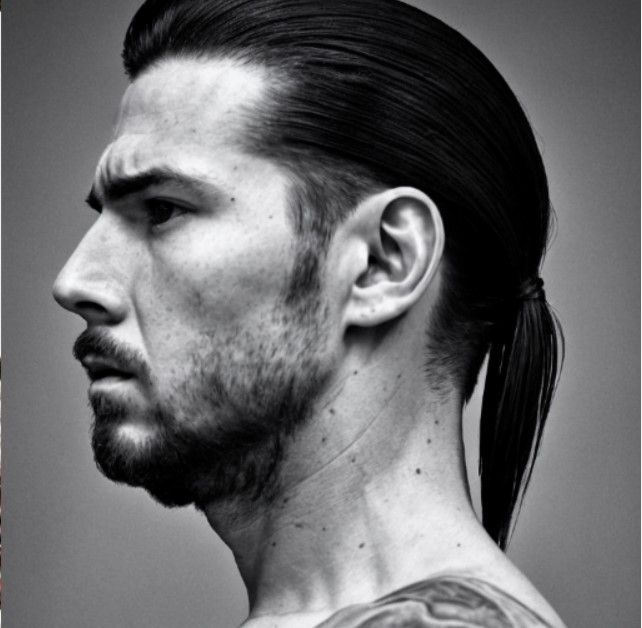
Emad Morpheus is a tech enthusiast with a unique flair for AI and art. Backed by a Computer Science background, he dove into the captivating world of AI-driven image generation five years ago. Since then, he has been honing his skills and sharing his insights on AI art creation through his blog posts. Outside his tech-art sphere, Emad enjoys photography, hiking, and piano.