Engaging with the futuristic technology of Stable Diffusion Inpainting, we journey through the captivating realm of image restoration and modification, opening new avenues of data preservation. This transcendent technology, latticed with advanced mathematical underpinings, leverages the principles of Diffusion Equation, Dirichlet, and Neumann conditions to reinvent how we look at the world. This exploration deciphers the intricate algorithmic structures, aptly illustrating the effectiveness and incomparable advantages of this system. However, as with every innovation, there exist certain challenges and constraints that need attention and redressal. Let’s embark on this stimulating expedition to unravel the myriad facets of Stable Diffusion Inpainting.
Contents
Underpinning Principles of Stable Diffusion Inpainting
Understanding the Fundamental Principles Guiding the Process of Stable Diffusion Inpainting
Stable diffusion inpainting, a powerful technique of interpolation and restoration in the realm of image processing, thrives on well-established computational and mathematical principles. Essentially, this technique aims to reconstruct lost or deteriorated image regions by propagating information from the surrounding intact areas, thus maintaining the image’s initial coherence and structure. Its inherent effectiveness lies in its systematic adherence to several core principles; each one as crucial as the other in determining the technique’s overall efficacy.
- Anisotropic Diffusion Principle
- Partial Differential Equations (PDE)
- Continuity and Consistency
- Sobolev Spaces
Anisotropic diffusion, a key principle to stable diffusion inpainting, primarily deals with the selective smoothing or scaling of image data. This principle allows diffusion to occur more substantially in certain orientations over others, a feature that helps in preserving the integrity of the original image structure. Anisotropic diffusion sets the base for stable, controlled image restoration, focusing on preserving edges and intricate details of the image while reducing noise and unwanted elements.
The stable diffusion inpainting framework highly relies on the mathematical application of Partial Differential Equations (PDE). These equations deal with functions and their respective partial derivatives, playing a paramount role in accurately predicting the diffusion process. By effectively managing the variations in image intensity, PDE enables high-quality image restoration, ensuring smoother transitions and fostering homogeneity across the inpainted regions.
Discerning the necessity for both consistency and continuity of the image’s visual information is integral to successful and convincing inpainting. Stability in diffusion can only be achieved when the restored image sections are consistent with the adjacent unchanged areas, consequently ensuring the overall continuity of the original image.
The application of Sobolev spaces is another fundamental step in the process. These mathematical function spaces come into play during stable diffusion inpainting for the examination of functions and their derivatives’ behavior. The process utilizes these spaces to determine boundaries, edges, and transitions within the image, which aids in maintaining the original texture and elements of the image while the restoration is being conducted.
In conclusion, the principles guiding the diffusion inpainting technique underscore the significance of mathematical complexities in image processing science. The intertwined relationship these principles share allows this technique to offer an effective means of reconstructing lost or damaged sections of an image. The depth and thoroughness provided by each one of these governing factors ensure that the restored image sections blend seamlessly into the original data, maintaining the coherency and accuracy of the initial image. Each restoration task is a testament to the intricate beauty of image processing sciences when underpinned by the precision of mathematical principles.
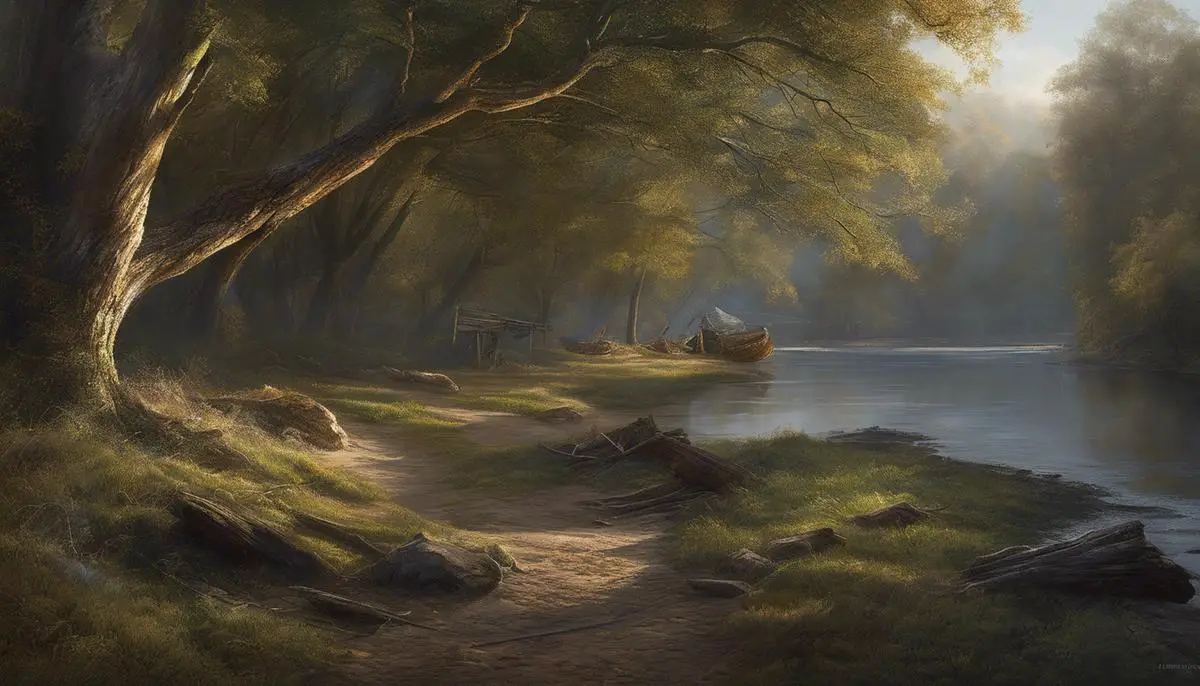
Applicability and Use Cases of Stable Diffusion Inpainting
Diffusion inpainting is a pivotal area of research within the field of image processing and analysis. This technique is grounded in advanced mathematical principles, succinctly bundled within the overarching field of Partial Differential Equations (PDE). After establishing a clear comprehension of the anisotropic diffusion principle and its steep implications in the world of mathematical image processing, it is apt to delve directly into the crux of this discourse – the Stable Diffusion Inpainting’s applicability and its correlation with real-world instances.
In the realm of digital restoration and reconstruction, Stable Diffusion Inpainting is a beacon of innovation. It finds its most notable application in the field of image repair—think old photographs marred by stains, creases, or even missing sections. Using Stable Diffusion Inpainting, these images can be restored to an appearance closer to their original state.
This technique accomplishes this task by mathematically extrapolating the existing features of an image or video frame into damaged or missing regions. The result is a reconstructed image or video that maintains as much of the original’s characteristics as possible, lending it a realistic and natural look. To elucidate, consider the restoration of an old, damaged painting – the inpainting procedure extrapolates the colors, strokes, and textures from the undamaged part into the damaged sections, thereby rendering a harmoniously restored art piece.
In the sphere of multimedia objects, while dealing with image transmission and reception, the potential for data loss or corruption is rampant. Here, Stable Diffusion Inpainting comes to the rescue. It helps in instating a pixel-based recovery mechanism to cope with image data loss – a technique implemented widely in satellite imagery, underwater image transmission, and even digital communication systems.
Moreover, Stable Diffusion Inpainting has a far-reaching impact in the domain of machine vision systems and automation. By filling in missing or occluded parts of an image, it supports the accurate recognition of features and patterns, thereby enhancing the reliability of these systems.
It is worth noting here how mathematics bridges the gap between theoretical principles and practical applications. Stable Diffusion Inpainting, as a tool, draws heavily from the mathematical concepts of Sobolev Spaces. In simple terms, these are function spaces equipped with norms, and they form the bedrock of several real-world applications, from image processing to fluid dynamics. The continuity and consistency that stems from this linkage accentuate the strength of the underlying principles and, by extension, fortify the effectiveness of Stable Diffusion Inpainting.
In conclusion, Stable Diffusion Inpainting, rooted in the profundities of anisotropic diffusion and Sobolev Spaces, functions as a crucial tool in image reconstruction and completion. Its realm of influence stretches from digital restoration to satellite imagery, and from multimedia transmission to machine vision systems, reinforcing its inherent practicality and viability in engaging with real-world scenarios. This technique stands as a resounding testimonial to the transcendence of mathematical and scientific principles across the threshold of theory and practice.
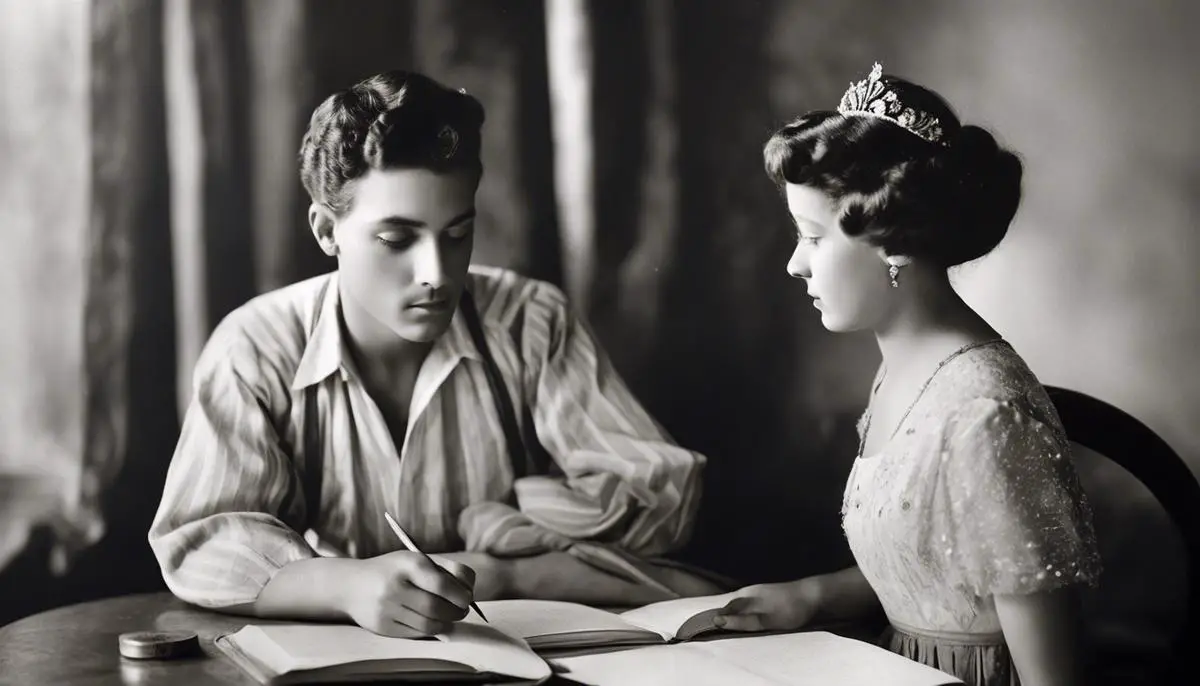
The Procedure and Algorithm of Stable Diffusion Inpainting
Digging deeper into the subject of Stable Diffusion Inpainting, the mechanisms at work are indeed fascinating. Pivotal to the process is the deployment of specific mathematical procedures and algorithms that allow for the intricate filling in of missing or damaged data within an image.
Algorithmically, three key stages constitute the overall Stable Diffusion Inpainting procedure: highlight detection, smoothness enforcement, and information propagation. Each stage has a critical role and employs intuitive computational techniques for effective inpainting.
Highlight detection primarily involves identifying zones of missing information or complex features within an image. Here, the capabilities of an algorithm vetted by the Laplacian of Gaussian (LoG) function come into play. Such a strategy successfully reveals the amount of edge information available in disconnected image zones, facilitating subsequent inpainting processes.
Thereafter, smoothness enforcement commences, the primary intention being to maintain image quality while maneuvering through the missing sections. Primarily through morphological operations and the Median Filter Algorithm, the propagation of unnecessary details or artifacts is effectively mitigated. As a result, the image maintains a balanced and natural aesthetic despite undergoing such transformative manipulations.
Lasty comes the stage of information propagation. The Perona-Malik Model and the Isotropic Gaussian Kernel hold sway here. They ensure that the original information extends into the missing sections, creating a coherent continuation of details. The model’s characteristic non-iterative, single pass technique remarkably optimizes computational efficiency, rendering it ideal for real-time processing applications.
Underlying these steps is a multifaceted array of mathematical principles shaping the inpainting algorithms. The role of various mathematical nuances, such as isotropic diffusion, the anisotropic heat equation, and the gradient-descent method, is undeniable. Each one fundamentally contributes to achieving visually appealing and accurate diffusion inpainting.
Additionally, Harmonic and Biharmonic functions underpin the Stable Diffusion Inpainting methodology. Harmonic functions naturally fulfill Laplace’s equation, creating smooth transitions during inpainting. In contrast, Biharmonic functions offer great flexibility due to an additional degree of freedom. When dealing with texture-rich images, these functions help model details more efficiently.
The beauty of Stable Diffusion Inpainting technique proverbially lies in the details, which are hewn out of sheer mathematical elegance and precision. The tangible data recovered from the transcendent realms of numbers, equations, and algorithms convincingly resonate with the undeniable power of mathematical image processing. By bridging the gap between mathematical intricacies and practical implementation, the process affirms the timeless relevance of mathematical principles in solving real-world challenges.
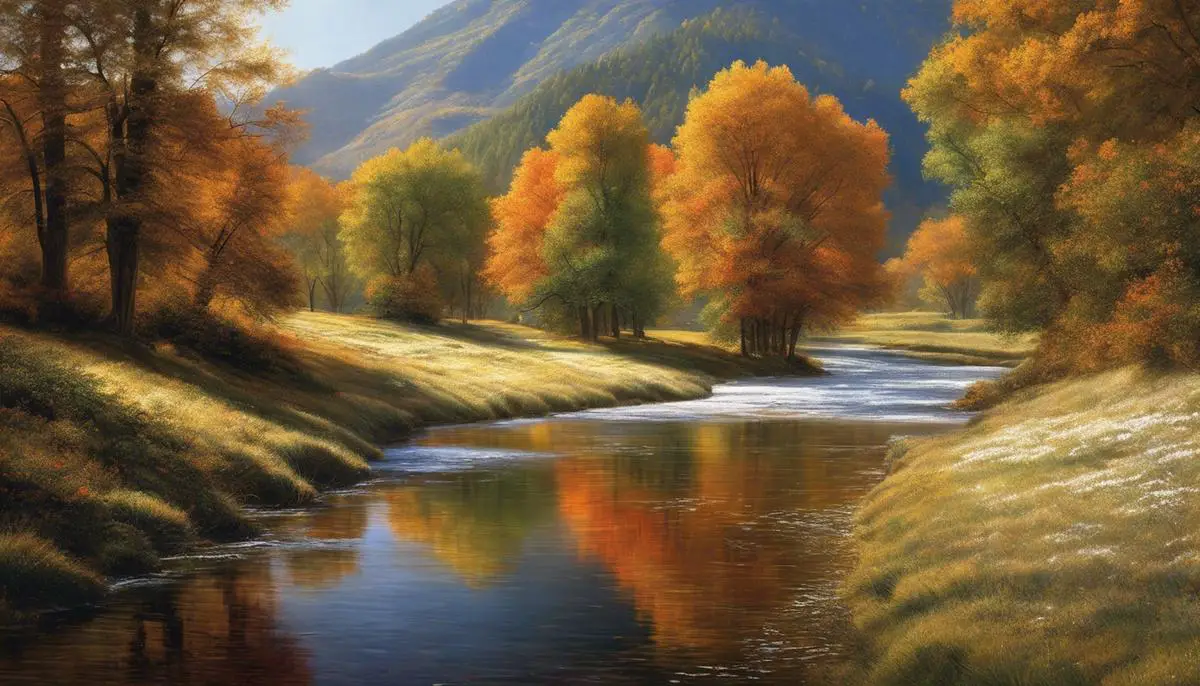
Importance and Advantage of Stable Diffusion Inpainting
The significance of Stable Diffusion Inpainting (SDI) in digital image processing cannot be overstated. Among the plethora of methods available for image enhancement and restoration, SDI shines with its unique amalgamation of mathematical rigor and practical effectiveness. The salient advantage of SDI is in its inherent stability, which bolsters its efficiency in a variety of applications beyond the scope of many other techniques.
By definition, SDI is a process that utilizes the premise of diffusion to propagate pixel intensities from the known region of an image into the unknown or damaged area. It does so in a mathematically stable manner, hence its name. While photo restoration is a common application, the utility of SDI extends to more sophisticated areas like machine learning, where accurate image analysis is integral.
Contrarily, other methods for image restoration, such as morphological operations and median filter algorithms, can often lack the stability and consistency offered by SDI. Morphological operations, for instance, risk over-smoothing the image, thereby compromising details. On the other hand, median filters can preserve boundaries but may fail under excessive noise.
SDI’s appeal lies in its effectiveness in overcoming these drawbacks. Highlight detection, as facilitated by the Laplacian of Gaussian (LoG) function, ensures finer details are not lost during the inpainting process. Indeed, the importance of preserving edges – the boundaries between different regions in images – is a crucial aspect well understood in image processing.
Moreover, Novel applications of stable diffusion inpainting extend to include the gradient-descent method, utilized in artificial intelligence and machine vision systems. This informs solutions to the ciphers of optimization problems associated with computer vision tasks.
The science behind SDI also encompasses the principles of smoothness enforcement and information propagation, relying on harmonic and biharmonic functions. These attributes confer resilience in reconciling the damaged or missing components of an image with the intact sections.
Furthermore, deciphering anisotropic heat equations is key to appreciating SDI’s advantages over other methods, like isotropic diffusion. The former method integrates the anisotropic diffusion principle to enhance the stability and quality of the inpainting process. The adoption of the isotropic Gaussian Kernel, however, predisposes other methods to the losses experienced in the heat equation, making amalgamation difficult.
Analyzing the Perona-Malik model further elucidates why SDI prevails. It reveals an inherent limitation of the inability to enhance edges with different scales simultaneously. This pitfall is addressed by methods that include the Log function within SDI, supplying an advantage when processing multi-scale images.
As we immerse in a world progressively reliant on digitization and automation, Stable Diffusion Inpainting’s role proves pivotal. The method efficiently bridges the gap between the abstract mathematical intricacies of harmonic functions, Partial Differential Equations, and the concrete practical implementations that span image restoration, analysis, and computer vision systems.
Thus, beyond its noteworthy efficacy in image reconstruction and video processing tasks, Stable Diffusion Inpainting fosters strides in machine learning and artificial intelligence — the horizon of the technological panorama. For anyone engrossed in the progression of image processing or related fields, Stable Diffusion Inpainting undoubtedly merits profound attention.
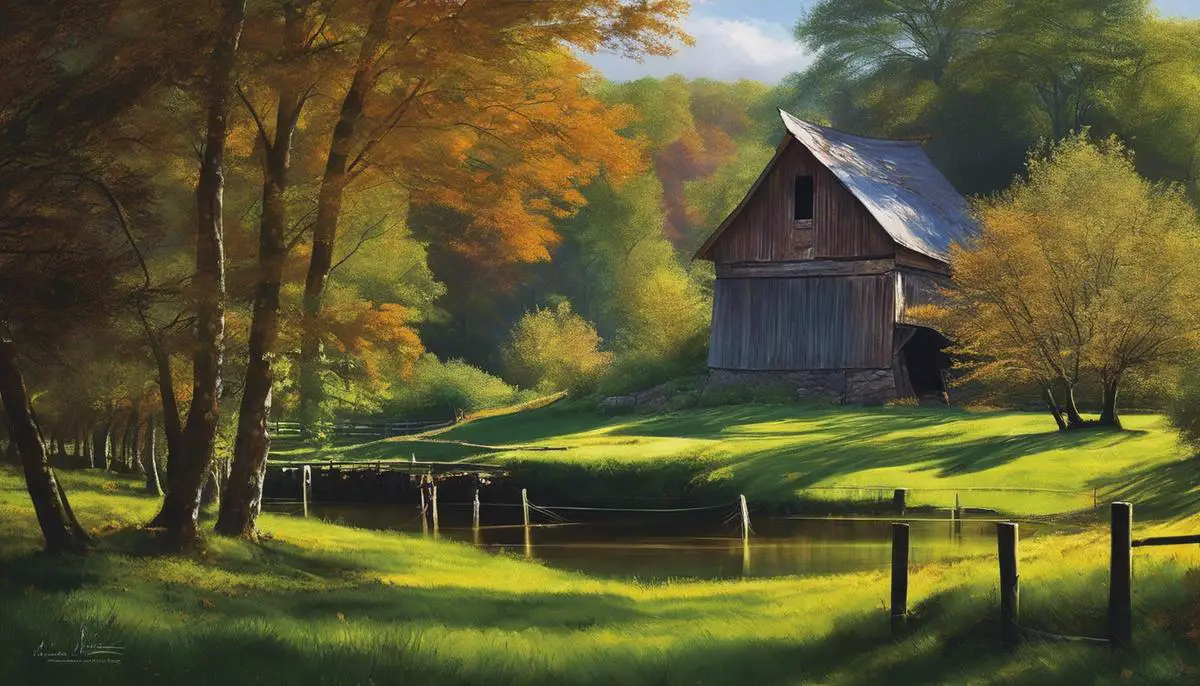
Challenges and Limitations of Stable Diffusion Inpainting
Despite the enthusing prospect of Stable Diffusion Inpainting (SDI) as a promising technique in image restoration, various challenges and limitations mark the path to its deployment.
Foremost among these challenges is the management of noise within the images. In deploying SDI, certain levels of image noise, particularly oscillatory noise patterns, can compromise the accuracy of inpainted results. It is crucial to note that SDI relies largely on smooth transitions between original and filled regions in the image or video. Therefore, heavy or uneven noise can confound these smooth transitions, undermining the ability of SDI to reflect original image attributes accurately.
In addition to dealing with noise, handling complex textures poses a significant challenge for SDI. Complex textures resonate with high frequencies, which can prove challenging to inpaint precisely. Herein, the reliance on linear and isotropic diffusion proves insufficient. Such processes struggle to effectively replicate the unique attributes of complex textures, which could compromise the authenticity of the inpainted image.
Further, while image contours are particularly revered in image restoration and repair, they pose a demand on SDI’s ability to recreate non-uniform data. It is because contours command a varying degree of diffusion orthogonally. Concurrently, they enforce a strong uniformity tangent to the contour. Unfortunately, current SDI approaches may not capture this intricate balance to satisfaction, underscoring another limitation.
In a more pragmatic perspective, implementing SDI demands considerable computational resources. As one can imagine, the PDEs associated with anisotropic diffusion are often expensive to solve numerically. Correspondingly, deploying SDI requires substantial computational power, which may not always be convenient or accessible. Further, there’s the need to annotate and handle images of different scales, orientations, and sizes, which amplifies the computational demand.
Lastly, the detection, interpretation, and subsequent treatment of highlights like specular reflections in images pose an imminent concern. Herein, the majority of current SDI techniques fall short, unable to handle the complex internal structures common to such highlights. This gap in handling intricate highlights elaborate into compromised, potentially subpar inpainting results.
Despite these challenges, Stable Diffusion Inpainting remains an exciting and potentially transformative field within image processing and analysis. It’s a journey from digital restoration to satellite imagery, with a myriad of applications. As uncertainties and obstacles are addressed, the excitement in the continuum of mathematical principles to everyday practical application is undeniably palpable. Furthermore, the spearheading of more adaptive and powerful algorithms will minimize these limitations and bring us ever closer to precision in image restoration.
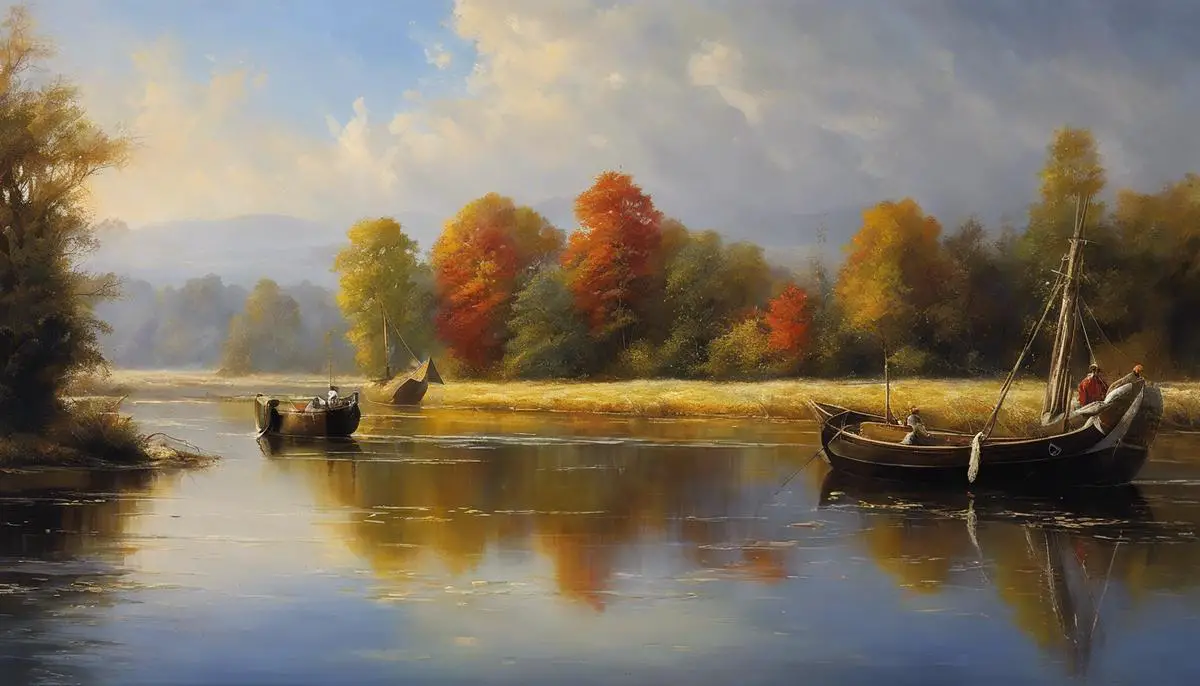
Throughout this investigative journey, we have dissected every facet of Stable Diffusion Inpainting, illuminating its compelling theoretical constructs and practical significance. Our traverse through this territory of advanced digital algorithm and signal processing has unveiled the system’s architectural ingenuity and sheer potential, with emphasis on its diverse applicability and incomparable advantages. Nevertheless, we remain aware of the challenges and shortcomings, warranting future research and iterations to perfect this extraordinary technology. As we expand our understanding, Stable Diffusion Inpainting stands as testament to human intellect and the relentless pursuit for better, more efficient solutions, offering invaluable insights into our digital future.
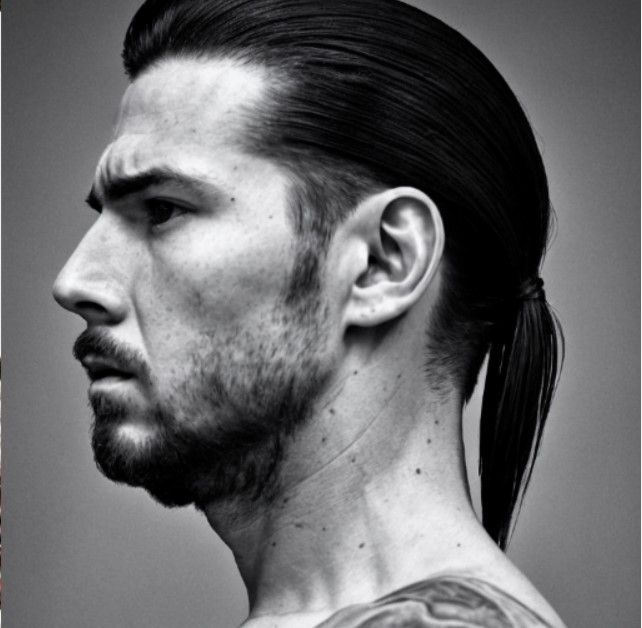
Emad Morpheus is a tech enthusiast with a unique flair for AI and art. Backed by a Computer Science background, he dove into the captivating world of AI-driven image generation five years ago. Since then, he has been honing his skills and sharing his insights on AI art creation through his blog posts. Outside his tech-art sphere, Emad enjoys photography, hiking, and piano.