As we accelerate towards a future heavily influenced by artificial intelligence, comprehending the novel concept of stable diffusion becomes absolutely pivotal, especially for college students poised at the frontier of such technological advancements.
This knowledge not only enhances our understanding of AI, but also acts as a catalyst for creative imagination, pioneering the generation of high-quality AI images. Through the journey of deep exploration into the AI realm, we will delve into the essentials of stable diffusion prompts right from its conceptualization, further enlightening ourselves on the intriguing process of AI images creation.
The excursion doesn’t stop here, as we go forth and polish this newfound knowledge while diving deep into intricate aspects related to further education in this sphere.
Contents
Understanding AI and Stable Diffusion
Understanding Artificial Intelligence
AI, or artificial intelligence, is the field of computer science dedicated to creating machines capable of intelligent behavior. These systems are designed to mimic human intelligence, learning to perform tasks and make decisions based on data inputs. It is an interdisciplinary field that incorporates aspects of computer science, mathematics, cognitive psychology, and engineering. Key areas of AI include machine learning, natural language processing, robotics, and computer vision.
Machine Learning: The Heart of AI
Machine learning is a key aspect of AI, allowing systems to learn and improve from their experiences. It uses statistical techniques to give computers the ability to learn without being explicitly programmed. The process involves feeding data into algorithms that then develop models that can predict outcomes or patterns based on that data. Stable diffusion is a recent advancement in this area.
Exploring Stable Diffusion
Stable diffusion is a perspective in machine learning related to generative modeling. Generative models are a specific type of machine learning that allows for the generation of new, unseen data, resembling the data it was trained on. They are widely used in image, video, and voice generation.
The basic idea behind stable diffusion is to produce noise-added intermediate images that gradually transition from a known original to a new generated image. The transition occurs in a series of steps, where each step includes adding a bit of random noise. This process diffuses the dependencies of the original image across each step, leading to high-quality generated images.
Crafting the Perfect Prompt for AI Images
When creating AI images, your prompt is the description you provide the AI system for what you want it to generate. For instance, for a stable diffusion AI image generator, you might input a prompt like “a bright pink flower with yellow leaves.”
The key to crafting a perfect prompt is specificity. Be very clear and detailed in your instructions to the AI. Yet, at the same time, leaving some room for creativity can lead to more interesting results.
Example Prompts
- “A vintage town square under the warm glow of a setting sun, with a brick pavement and quaint little shops on either side.”
- “An astronaut floating in space with planet earth in the background.”
- “A majestic unicorn standing at the edge of a sparkling turquoise lake, with a rainbow in the sky.”
- “A dense forest of ancient redwood trees, bathed in the soft, cool light of early morning.”
Remember, trial and error is part of the process. Refining your prompts and learning how the AI responds is a fundamental part of harnessing the power and potential of AI images. Don’t be afraid to experiment and iterate as you explore and learn.
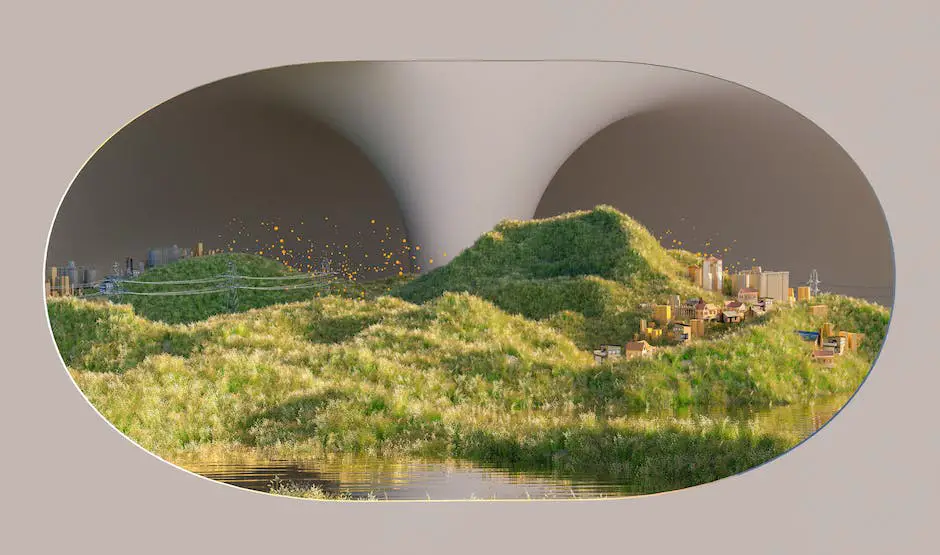
Designing Stable Diffusion Prompts
Understanding Diffusion Prompts
Diffusion prompts operate as critical guides for artificial intelligent models deployed for art generation tasks. They are textual cues that instruct the model in producing specific output, with factors such as complexity, clarity, and semantics significantly affecting the outputs yielded. For instance, a prompt like “illustrate a calm beach during sunset” can generate an image of a serene beach scene with a setting sun.
Effective Design of Stable Diffusion Prompts
Creating a stable diffusion prompt involves balancing between specificity and ambiguity. A prompt should give the AI model enough direction to elicit the desired output, but it also needs to have a degree of ambiguity to leave room for the AI’s creativity. For example, a prompt like “draw an eagle in its natural habitat” puts forth a definitive requirement while leaving ample liberty for the AI to fill in with details.
Incorporating Contextual Information
Providing context within your diffusion prompts adds another layer of direction for your AI model. Contextual information can take different forms like historical eras, geographical areas, or even emotional states. For example, “display a bustling cityscape during the industrial revolution” or “depict a vast dessert under a melancholic sunset”. This way, the AI has additional guiding points directing not only the main elements but also the overall mood or aesthetic tone of the outcome.
Ensuring Correct Semantics in Prompts
In addition to specificity and context, another crucial aspect of designing diffusion prompts is ensuring proper semantics. Often AI models struggle to comprehend abstract concepts that humans easily understand. Consequently, it’s essential to use clear, straightforward language that the AI model can easily interpret. A prompt like “draw a person feeling melancholic” could be confusing to the AI, as it’s hard for it to visualize emotions. Instead, opting for “draw a person with a sad expression sitting alone” gives the AI a clear, concrete instruction to follow.
Applying Styles and Formats in Your Prompts
Incorporating specific styles and formats into your prompt can help instruct the AI about various aspects such as structure, perspective, coloring, shading, and more. For example, “Draw an apple using cubism art style” or “Produce a black and white portrait of a woman staring directly at the viewer”.
Testing and Refining Your Prompts
Designing stable diffusion prompts is an iterative process. After formulating a prompt, you should consider testing it and evaluating the output produced by your AI model. If the results aren’t in alignment with your expectations, try tweaking and refining the prompt and its components. This process of trial and error aids in the development of increasingly effective prompts over time.
Remember, the success of a diffusion prompt significantly depends on the ability to create a balance between specificity and openness, providing context, ensuring correct semantics, and expressing style preferences clearly. With conscious practice and creative exploration, you will enhance your abilities in designing powerful diffusion prompts for AI image generation tasks.
Creating AI Images using Prompts
Getting Started with AI Image Generation
AI image generation is an exciting aspect of artificial intelligence’s capabilities. By using textual prompts, AI can generate images that align with the written description. A stable diffusion prompt for AI images functions as the ‘guide’ for the AI, telling it what to craft. You might be asking the AI to create something as simple as a “blue bird standing on a tree branch” or as complex as a “augmented cyborg in a post-apocalyptic world.”
Choosing the Right Tools for AI Generation
Selecting the right software tools to create AI images is crucial. Machine learning models such as DeepArt, ArtBreeder, Deep Dream Generator, and Runway ML are widely popular and known for their consistent results. These AI models interpret the prompts and produce images based on their learning and understanding.
Working with Prompts
Written prompts should be descriptive and clear to guide the AI towards generating the envisioned images. For example, instead of “a running dog”, use “a Border Collie sprinting on a green meadow” to allow AI to understand the breed and surrounding. Remember, your AI tool is like an artist, and the prompts you give are its inspiration.
Using the Tools
Upon selecting the desired software tool, the process of creating an AI image generally follows the same basic steps. Input the written prompt into the software, choose the level of creativity you want the AI to have — if your software allows this, and initiate the process for the AI model to create the image. The level of ‘creative freedom’ you give the AI can influence the final image. Remember that while AI can generate excellent and unexpected creations, they are not perfect. Some generated images may require tweaking and multiple attempts.
Achieving the Best Results
To get the most from AI image generation, you may need to experiment and iterate. Adjust your prompts, try different models, or try the same prompt with varying creative freedom levels. By analyzing the success and shortcomings of each attempt, you’ll gain a better understanding of the type of prompts that produce the best result for your chosen AI tool. In time, you’ll develop an intuitive knack for writing practical and successful prompts.
Exploring Different Models
Different AI models may prioritize varying aspects of your prompt or interpret it uniquely. Using the “Border Collie sprinting on a green meadow” example, one tool might showcase the speedy motion of the dog, while another focuses on detailing the dog’s breed characteristics. This is why it’s important to familiarize yourself with the common AI models used for image generation. As you try different models, you’ll inevitably find that some work better with certain types of prompts than others.
In conclusion, mastering the art of AI image creation comes with time, practice, and a willingness to explore the vast possibilities that AI offers. Its potential is immense – let your creativity steer its potential.
Refinement and Further Study
Expanding your Knowledge Base
Before embarking on any journey, it’s imperative that you are well-equipped with the right knowledge and skills. As a college student interested in exploring diffusion prompts to create AI images, your first step should be to understand the foundational theories behind it. Set aside a daily or weekly schedule dedicated to reviewing materials from AI-related subjects and deep diving into specific areas of interest. Use a variety of sources such as textbooks, online platforms and research papers to enrich your understanding and exposure to various perspectives.
Hands-on Learning
Once you’ve grasped the basic concepts, the real learning begins. Implement what you’ve learned by coding the algorithms yourself. The advantage of hands-on practice is that you can gain first-hand experience of various problems, thereby learning how to navigate around them. Coding platforms like Jupyter, Google Colab and Kaggle are a few of the places you can start with for hands-on learning.
Advance Learning
As you evolve in your learning, challenge yourself to understand complex concepts and applications. Don’t shy away from daunting topics such as Bayesian Networks, Reinforcement Learning and advanced GANs. Occasionally, take up comprehensive projects that allow you to connect and apply what you’ve learned on a bigger scale.
Problem-solving and Approximate Solutions
AI learning is full of unscripted problems. Confronting these issues and generating approximate solutions is key to learning how to respond to real-life situations. Joining online communities such as StackOverflow and GitHub can open up avenues for you to problem-solve collaboratively, and also to learn from the responses of your peers and more experienced professionals in the field.
Familiarize with AI Algorithms
An integral part of learning AI image creation is familiarizing yourself with its critical algorithms. Start by learning the most used AI algorithms and understanding their strengths and weaknesses. A few to start with include Decision Trees, Neural Networks, Logistic Regression, and Support Vector Machines.
Understanding Generative Adversarial Networks (GANs)
GANs are one of the most revolutionary concepts in AI. Initially proposed by Ian Goodfellow in 2014, GANs have become a primary tool for generating synthetic data. Devote a significant amount of study time to understand the architecture of GANs, their variants, and their diverse usage. The knowledge gained about GANs will be a significant asset when you start creating AI images.
By following these steps, you will not only amass a wealth of knowledge and skills, but you’ll also apply and grow in the field of AI. Continuous learning, patience, and practice are the keys to mastering any subject, AI included.
By perusing this comprehensive guide, you, as ambitious learners, are stepping into an exciting era where art and artificial intelligence intersect in the most brilliant ways. Advanced comprehension of stable diffusion, prompt creation for artificial intelligence images, and practical implementation of these prompts have been our key targets throughout.
We’ve equipped ourselves with the virtues of practicality, deep understanding, and self-improvement, paving our way forward in this technologically vibrant future. As we harness the power of generative adversarial networks and various algorithms, we are not only refining our own skills but are also contributing towards an AI-driven world teeming with endless possibilities.
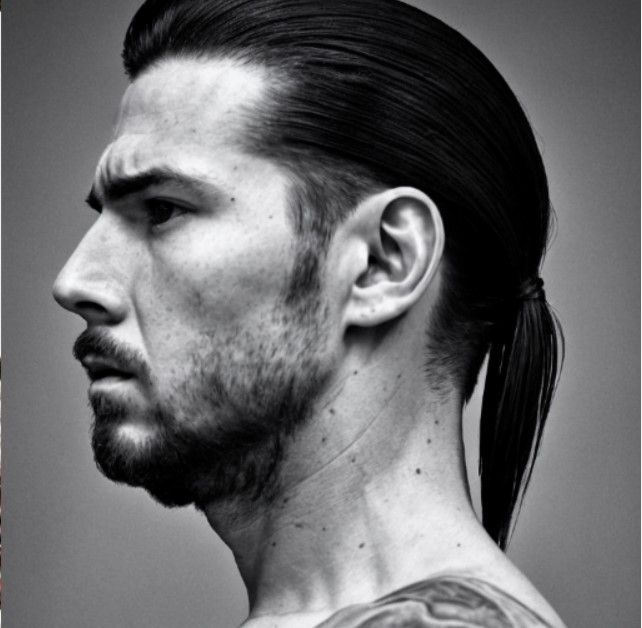
Emad Morpheus is a tech enthusiast with a unique flair for AI and art. Backed by a Computer Science background, he dove into the captivating world of AI-driven image generation five years ago. Since then, he has been honing his skills and sharing his insights on AI art creation through his blog posts. Outside his tech-art sphere, Emad enjoys photography, hiking, and piano.