Widely regarded as one of computer science’s most fascinating frontiers, Artificial Intelligence (AI) image generation combines intricate algorithms, creativity, and practical applications to engender a stupendous revolution in many sectors.
Leveraging the power of advanced algorithms, this system can fabricate highly realistic images, significantly influencing areas such as technology, design, and medicine. This intriguing form of AI amalgamates several areas of study, avant-garde techniques, including Generative Adversarial Networks (GANs) and autoencoders, to facilitate the generation of strikingly authentic images.
This paper delves into the multifaceted aspects of AI image generation, the methods that drive it, the inspiring innovations, and the pertinent ethical and legal deliberations. It further extrapolates on the potential future trajectories of this pioneering technology.
Contents
Understanding AI Image Generation
Defining AI Image Generation
AI image generation refers to the process where artificial intelligence (AI) systems generate new images that were not previously existing. This is accomplished through a type of AI known as Generative Adversarial Networks (GANs). The primary purpose of this system is to create realistic and high-quality images that can be incorporated into various applications, ranging from entertainment to professional environments.
The Technical Aspects of AI Image Generation
Under the hood of AI image generation are Generative Adversarial Networks, a class of machine learning frameworks invented by Ian Goodfellow and his colleagues in 2014. It consists of two neural networks: a generator network and a discriminator network.
The generator network produces images while the discriminator network determines if the images generated are real (existing in the training set) or fake (produced by the generator). This duel leads to the generator improving its work, attempting to create images that the discriminator cannot distinguish from the real ones.
Typically, the generator begins the image creation process through a vector in a latent space. This process often involves generating pixels randomly and then refining them. On the other hand, the discriminator employs convolutional neural networks to classify the veracity of the image. The process continues until the generator evolves in its capability to produce realistic images that can deceive the discriminator.
Potential Applications of AI Image Generation
The capabilities of AI image generation have been seen in various sectors, reflecting its vast potential. In the field of technology, it aids in the development of video games and virtual reality (VR), where it generates realistic characters and environments, enhancing the user experience. Similarly, in the design industry, AI image generation can help in creating realistic designs and mockups, thereby accelerating the work process and reducing human effort.
The potential applications extend as well into medicine, where AI image generation can help in creating ‘synthetic’ patients’ digital records, including medical imaging like MRIs and CT scans. This can help medical researchers study diseases and develop treatments without infringing on patients’ privacy.
Moreover, in sectors like real estate and architecture, AI image generation can assist architects in visualizing design concepts more realistically to their clients. This technology can also be utilized in producing deepfakes and synthetic media, though it generates ethical and legal issues.
Exploring the Advances in AI Image Generation
The technological landscape of Artificial Intelligence (AI) is continuously evolving. Especially in the dimension of image generation, the introduction and refinement of Generative Adversarial Networks (GANs) have paved the way towards more significant innovations.
These advancements are routinely focusing on aspects like enhancing the overall quality and resolution of the generated images, addition of new functionalities in GANs, reduction in training time, and modifying GANs to accomplish specific tasks.
Aside from these technical developments, the ethical consequences and societal ramifications of AI image generation are also under in-depth research. This scrutiny specifically involves the potential misuse of the technology, such as deepfakes and their more nefarious variant – deepfake pornography.
Therefore, AI image generation is an exciting yet challenging sphere of AI research. It warrants constant monitoring and comprehension concerning its technological growth and its overall impact on the social, ethical, and regulatory segments of society.
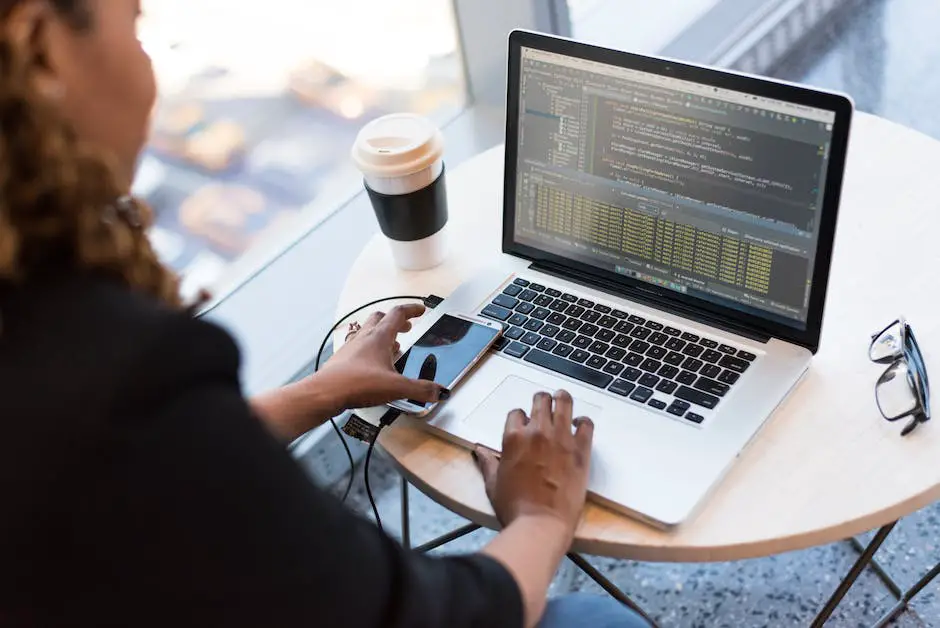
AI Image Generation Techniques
An Overview of Generative Adversarial Networks (GANs)
For years now, Generative Adversarial Networks, commonly referred to as GANs, have stood as a major game-changer in the AI image generation. Operating on an intriguing principle, GANs employ a dual neural network system consisting of a ‘Generator’ and a ‘Discriminator’.
The Generator creates images and submits them to the Discriminator, which has already been trained to differentiate between authentic and synthetic images. This clever setup allows the Generator to refine its capability of producing more realistic images via encouragement received from the feedback of the Discriminator.
Through this engaging adversarial process, GANs are capable of achieving incredible feats in image generation like creating non-existent human faces, manipulating the weather in external photographs, or even turning a simple doodle into a high-resolution image. Despite these exciting capabilities, training GANs remains a complex task.
The reason being – these networks need a meticulous balance in terms of learning rates, structural designs, and numerous hyperparameters. Moreover, GANs might encounter ‘mode collapse’, a situation where the Generator produces images with restricted diversity.
Autoencoders for Image Generation
Autoencoders, another primary method used for AI image generation, are neural networks that aim to copy their inputs to their outputs. They work by encoding the input into a compressed representation, and then decoding this representation back into the original format. For image generation, autoencoders can be trained to learn robust representations of images, which can then be sampled and decoded into novel images.
The advantage of using autoencoders is that they can capture the latent space of the input data in a more structured way than GANs, thus aiding in the generation of images that are visually closer to the original data. However, the challenge with autoencoders lies in controlling the generation process, as random sampling from the learned distribution often results in blurry or unrealistic images.
Exploring Other Techniques
Beyond GANs and autoencoders, recent years have witnessed a surge of various methods like Variational Autoencoders (VAEs), Modular Generative Adversarial Networks (MGANs), and Transformer-based models, all focusing on improving the quality and diversity of generated images. For instance, VAEs overcome some limitations of autoencoders by ensuring a smooth and well-defined latent space via a variational approach, while MGANs aim to improve the scalability and controllability of GANs.
The Impact on Image Quality and Variety in AI Generation
In the realm of artificial intelligence, various methodologies have made remarkable contributions to the quality and variety of images generated. Notably, Generative Adversarial Networks (GANs) have offered revolutionary results, producing images scarcely distinguishable from genuine photographs.
In the same regard, the ability of autoencoders and Variational Autoencoders (VAEs) to encapsulate structured representations has paved the way for a more diversified and controlled image generation. These techniques have proven invaluable in subfields such as computer graphics, photo-editing, and even in increasingly popular areas like virtual reality.
On the flip side, there are concerns surrounding the utilization of these techniques to create incredibly authentic content such as deepfakes, raising alarm for potential misuse. Hence, as we work on refining AI image generation methods, it becomes equally crucial to devise safeguards to detect and preclude any misuse of this advancing technology.
Latest Innovations in AI Image Generation
Image Synthesis in the Age of AI
In the expansive domain of image synthesis, the pivotal role of Artificial Intelligence cannot be overstated. One of the most noteworthy achievements in this area has been the formation and application of Generative Adversarial Networks (GANs).
GANs have brought about a paradigm shift in the field, with their unique ability to create high-quality, strikingly realistic images. Accomplishing this entails the training of two neural networks: a ‘generator’ network that produces images, and a ‘discriminator’ network that differentiates between real and fake images. Both networks are conditioned in a competitive environment, which encourages the generator to progressively produce more realistic images.
Advancements in GANs
Recent advancements in GANs have taken image generation to the next level. DeepArt and DeepDream are revolutionary projects that demonstrate art generation through AI, transforming simple images into artwork resembling the styles of famous painters. Features of these advancements include style transfer, where the style of one image can be applied to another image, leading to an entirely new image creation.
Innovations in Image-to-Image Translation
Image-to-image translation with GANs presents another groundbreaking research area in the AI image generation field. This refers to transforming an image from one domain (e.g., a daytime landscape) into an image from another domain (e.g., a nighttime landscape). Notable solutions in this field include Pix2Pix and CycleGAN, which has been used for tasks like transforming horses into zebras, or converting monochrome images to color, among others.
Progress in 3D Image Generation
AI has also made strides in the generation of 3D images, which is crucial for several applications, including gaming, virtual reality, and medical imaging. GANs, combined with Convolutional Neural Networks (CNNs), have successfully been used to generate high-resolution, textured, 3D models from 2D images. Efforts made by researchers at Google AI revealed their method for training GANs to generate 3D models from common 2D images.
Super-Resolution Image Generation
Super-resolution techniques using AI, particularly Deep Learning, have also shown remarkable progress. Super-resolution is the process of enhancing the resolution of an image, and this is immensely important for areas like satellite imaging, medical imaging, intelligence surveillance, and many more. SRGAN is a GAN-based network developed for super-resolution purposes. It takes a low-resolution image and upscales it while maintaining image quality.
Future of AI Image Generation
The future of AI image generation is promising, with research efforts focused on improving the realism, efficiency, and versatility of the generated images. Approaches are being refined to address present limitations, such as the issue of ‘mode collapse’ in GANs where the network ends up generating very similar images. Techniques like improved training algorithms and incorporation of reinforcement learning are being delved into to overcome these issues.
Indeed, the present era can be characterized as a time where image generation is much more than just displaying random pixels—it’s a manifestation of the profound capabilities of AI.
The pervasive AI technologies today are capable of generating top-notch, lifelike images, transforming existing photos, enhancing resolutions, and even conjuring 3D models. These tasks are accomplished with such efficacy that they’re making exceptional contributions to both the scientific and commercial markets. The relentless advancements in these technologies indicate a future wherein AI image generation will further blur the lines between reality and creativity.
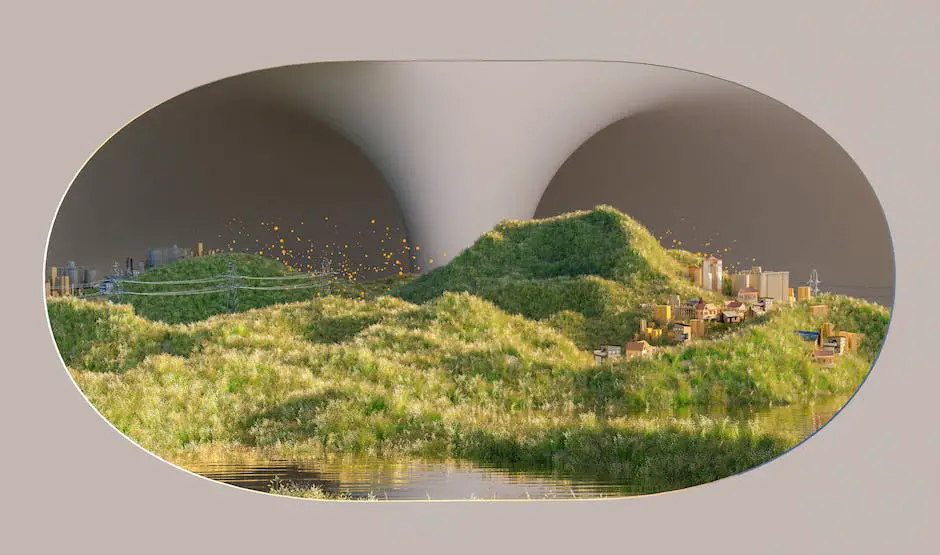
AI-Generated Images: Case Studies
Case Study 2: The Inception of NVIDIA’s AI Image Generation
As we delve deeper into the domain of AI image generation, we encounter a notable pioneer – NVIDIA’s Generative Adversarial Networks (GANs), and more specifically their StyleGAN series. Through their extensive research and development, NVIDIA has implemented an array of machine learning models capable of spawning photorealistic images. This process is facilitated by learning from an extensive repertoire of pre-existing images.
NVIDIA’s initial image generation approach employing GANs required their algorithm to fabricate a ‘latent’ space. This space can be imagined as an intricate mathematical domain where each point corresponds to a potential image.
Over time, the AI discerns to attribute certain points to distinct visual features, functioning as a kind of ‘genetic code’ for images. As a result, each of these individual points can be linked to specific features such as the color of the hair, facial expressions, or the existence of eyewear.
The outcome of NVIDIA’s research was nothing short of extraordinary. The groundbreaking StyleGAN model managed to churn out images of completely nonexistent individuals that were nearly indistinguishable from actual human photographs.
NVIDIA’s subsequent iterations of StyleGAN, including StyleGAN2 and StyleGAN2 ADA, not only rectified previous issues but also generated more high-quality and diverse images, demonstrating their ability to harness even insufficiently large datasets.
The repercussions of NVIDIA’s impactful research aren’t limited to academic circles. For instance, in the realm of entertainment, AI-generated images hold promise in manifesting more realistic characters in video games or enhancing special effects. In the design industry, this technology could fuel the development of tools that could create endless variations of a product for customer review.
Case Study 2: OpenAI’s DALL-E and CLIP
Another prominent case study comes from OpenAI. They employed a very different approach to AI image generation through the development of DALL-E and CLIP models. DALL-E, a variant of the highly successful GPT-3 text model, could generate images from textual descriptions, while CLIP provided understanding and translation capacity between texts and images.
In stark contrast to the GANs employed by NVIDIA, DALL-E and CLIP were not just about generating images from noise. These models could understand context and correlate it with image creation. For instance, when asked to generate ‘an armchair in the form of an avocado’, the models would understand the text description and generate a unique image fulfilling the given criteria.
The results generated by DALL-E and CLIP were surprising for the AI community. These models generated images that met the given descriptions yet were creatively more imaginative, such as an armchair with avocado-texture upholstery or an armchair shaped like an avocado halve.
The DALL-E and CLIP project’s implications are immense. Such a system could revolutionize the creation of digital art, provide novel methods for brainstorming product designs, or even lead to the generation of educational illustration aids.
Case Study 3: An Exploration into Google’s DeepDream
An intriguing perspective on AI-based image generation can be derived from Google’s innovative DeepDream project. This software is distinctive because, unlike other image-generating AI, it is designed to augment and amplify the features it identifies in pre-existing images, leading to a unique and somewhat psychedelically-enhanced visual output.
Google embarked on the DeepDream project with the intention of gaining a deeper understanding of how neural networks comprehend and classify features in images. The process involves inputting a pre-existing image, after which the AI identifies and repeatedly emphasizes the recognized features throughout subsequent layers.
Interestingly, the initial DeepDream images caused a stir due to their uncanny inclusion of unexpected elements, for instance, eyes, animals, and architectural features in landscapes and cloud imagery. Evidently, the software, which was extensively trained utilizing wildlife images, effectively incorporated these learned features into unrelated landscape photographs in surreal ways.
Similar to the previous case studies, the concepts behind DeepDream have been widely adopted and significantly influenced beyond the confines of Google’s labs. For instance, it has been utilized to create unique works of art and even made an impact on fashion design collections. It also serves as a significant tool for researchers, allowing them to visualize the inner workings of these multifaceted algorithms.
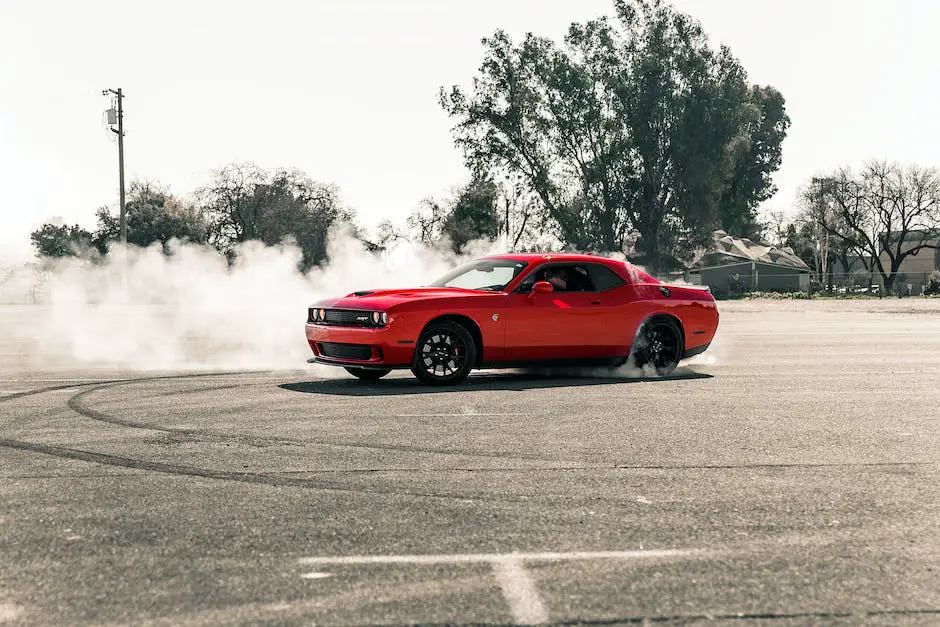
Ethics and Legalities in AI Image Generation
AI Image Generation: A New Frontier Marred by Potential Threats
The sphere of AI image generation has witnessed substantial growth, ushering in transformative changes across diverse industries, ranging from the realms of entertainment and gaming to vital sectors like security and healthcare.
Strategic methodologies such as Generative Adversarial Networks (GANs) or transfer learning have empowered AI to generate novel image content, carry out image morphing, and even draft photorealistic outcomes from rudimentary illustrations. Nevertheless, despite these impressive strides, it’s pivotal to acknowledge the consequential ethical, legal, and privacy concerns that emerge with these advancements.
Deepfakes and Information Manipulation
Deepfakes represent one of the most concerning phenomena associated with AI image generation. These are synthetic media in which a person’s likeness is replaced with someone else’s. With this technology, creating convincing and high-resolution fake videos or images that seem very real is becoming easier.
The potential for abuse is tremendous, from creating non-consensual pornography to fabricating political scandals. Deepfakes can damage reputations, promote disinformation, and even provoke conflict. It is a stark reminder of how AI can be a double-edged sword – its same capabilities that can entertain and engage us can also be used to deceive and harm us.
Copyrights and Intellectual Property Rights
AI image generation also raises complex issues related to copyrights and intellectual property rights. For instance, if an AI system generates an image, who owns the copyright? Is it the developer of the AI, the user who provided the input, or perhaps there’s no copyright claim at all since it wasn’t “created” in the traditional sense?
Plus, what happens if an AI generates a copyrighted or trademarked image unknowingly? Legal systems around the world are scrambling to keep up with these questions, wrestling with outdated legal frameworks that didn’t anticipate these issues.
Privacy Concerns
Privacy is another significant concern with AI image generation. Most AI systems are trained on vast datasets of images from the internet. If these include personal photos, there may be significant breaches of privacy, even if inadvertently so.
Moreover, technologies such as facial recognition and deepfakes can invade privacy by allowing unauthorized use of one’s likeness. A future where anyone’s image can be convincingly inserted into arbitrary contexts is a potentially frightening one.
Mapping the Path of AI Image Generation
To respond to growing concerns over deepfakes and misuse of personal data, the AI research community is pursuing various strategies. A key consideration is the development of AI systems capable of detecting deepfake images, which would ultimately provide a technological answer to a challenge spurred by technology.
Simultaneously, the establishment of legal and ethical norms for AI-derived content is being considered, with an aim to safeguard creative authenticity and uphold respect for personal privacy.
An alternative strategy involves advancing AI systems capable of generating images that do not rely on comprehensive personal data, therefore enhancing privacy protections. Direct user data policy changes could also be a potential solution, incorporating explicit data usage parameters designed to mitigate risks associated with privacy violations.
Indeed, AI image generation offers an exciting landscape with huge prospective gains. Yet, like all technologies, it must be controlled and monitored to prevent misuse. As we navigate and test the capabilities of AI, it is paramount to ensure its development and application are conducted in a manner that complies with ethical, legal and societal norms.
The Future of AI Image Generation
The State of the Art in AI Image Generation
At present, the field of AI is revolutionizing how we create digital imagery. Leveraging robust algorithmic processes, AI now has the capacity to yield highly realistic images that are virtually indistinguishable from authentic photos. The prime technique used to achieve this is the Generative Adversarial Networks (GANs), where a pair of AI systems operate in tandem: one generates an image, while the other evaluates its quality, fostering constant enhancement.
This technology has found useful applications in a variety of sectors. For example, in gaming, AI facilitates the construction of complex and minutely detailed game environments. Within the entertainment industry, AI powers the generation of animations and special effects, and even aids in the creatation of entirely digital characters.
Anticipated Trends in AI Image Generation
It is anticipated that advances in AI image generation will only escalate in the near future. One expected trend is the increasing use of AI in digital arts, especially in movie-making and video gaming industries, where realistic imagery is crucial.
This may involve further enhancement of graphics and animations, as well as creation and manipulation of digital characters. In the retail sector, customers may be able to ‘see’ how they would look in certain clothes without trying them on, simply through AI-generated images.
Another major trend is the further integration of AI image generation into technology design, such as developing more realistic virtual or augmented reality experiences. The details and accuracy of AI-generated images will also likely improve, making them even more indistinguishable from real photographs.
Potential Challenges and Solutions
While the advancements in AI image generation are impressive, they are not without their challenges. As the technology continues to grow and become more sophisticated, so does the potential for misuse. Deepfakes, or AI-generated fake videos and images, have raised concerns due to their ability to create disinformation and undermine personal reputations.
Addressing this issue will require a multi-faceted approach. Legislation may need to evolve to regulate the use and distribution of AI-generated images. Additionally, AI may also be employed to counter deepfakes—for instance, by developing algorithms that can detect AI-manipulated images and videos.
Impact on Various Industries
The impact of AI image generation will likely be broad, touching industries beyond gaming and entertainment. In healthcare, AI could be used to generate accurate and interpretable imaging from raw medical data, aiding in early and accurate diagnosis. In architecture and interior design, designers could generate realistic designs, allowing clients to visualize their plans in striking detail.
In education, AI-generated images could create highly detailed rendered environments, aiding in distance learning and virtual field trips. The automotive industry could use this technology for designing and virtually testing car models. The consequences of AI image generation are vast, promising unprecedented improvement and convenience in various facets of personal and professional life.
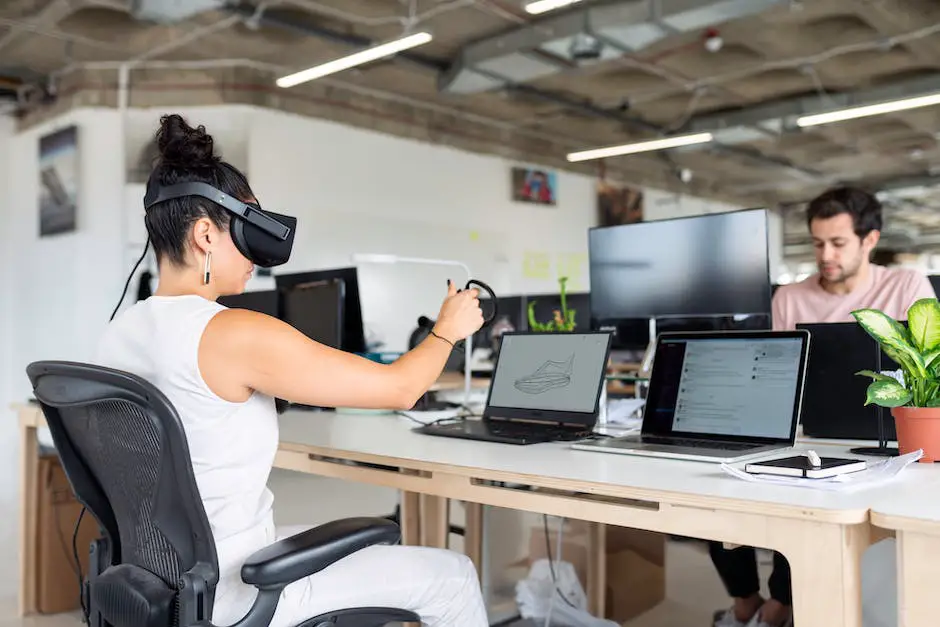
As we look towards an incalculable plethora of opportunities lying ahead in the realm of AI image generation, it’s imperative to embrace its transformative potential to rejig sectors like gaming and entertainment, among others.
However, simultaneously, we must not lose sight of the pertinent challenges, ethical quandaries, and the robust, proactive solutions needed to navigate the course of this powerful technology. From changing the way we interact with digital media to revolutionizing critical sectors like healthcare, AI image generation has just begun unveiling its limitless potential.
With ongoing innovations and dedicated research, the future of AI Image Generation is teeming with unprecedented possibilities, promising an amalgamation of technological prowess and creativity like never before.
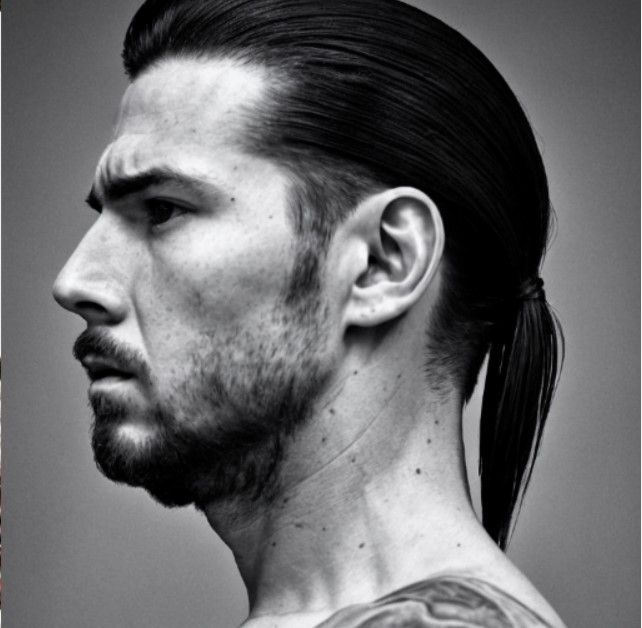
Emad Morpheus is a tech enthusiast with a unique flair for AI and art. Backed by a Computer Science background, he dove into the captivating world of AI-driven image generation five years ago. Since then, he has been honing his skills and sharing his insights on AI art creation through his blog posts. Outside his tech-art sphere, Emad enjoys photography, hiking, and piano.