In the world of digital images and photography, one of the most challenging issues to address is image noise. Image noise not only degrades the quality of the digital visual but also hampers the overall process of image processing.
With the advancement of technology and the necessity for clear, crisp images especially in fields like healthcare and satellite imaging, there’s an increasing demand for effective noise reduction techniques. This exploration dives deep into Stable Diffusion, one such highly effective noise reduction method, elucidating its underlying principles, functionality, latest developments, and real-world applications in various domains.
Contents
Basics of Image Noise and Its Impact
Understanding Image Noise
Digital image noise is often described as unwanted random variations in brightness or color in an image. In simpler terms, these are the visually disturbing patterns you sometimes see in digital images, digital photographs, and video frames. Image noise can be attributed to different sources like the camera sensor’s image acquisition process, data transmission errors, or unwanted interference.
Effects of Noise on Image Quality
Digital image noise greatly affects the readability and visibility of an image. Hence, noise creates distractions and disturbances, reducing the overall image quality. It limits an image’s dynamic range and disrupts the accurate extraction of meaningful features from the image, posing a problem in image analysis, modeling, and interpretation.
The Need for Noise Reduction Techniques
In light of the damage that noise causes to images, it becomes obvious why noise reduction or denoising techniques are essential in digital image processing. Noise reduction is designed to enhance the visibility of an image by reducing or entirely eliminating the noise content without disturbing the original image content.
Noise reduction techniques find extensive applications in various fields such as medical imaging, satellite imaging, multimedia, forensic image analysis, and many others. The ultimate goal of these techniques is to preserve the meaningful attributes of an image while enhancing its aesthetic and analytical quality.
Reducing Noise through Stable Diffusion
Stable Diffusion, based on the principle of anisotropic diffusion, considers an image data set’s anisotropic properties. Unlike scale-space filtering, which averages out the neighboring pixels and sometimes results in edge sharpness and fine detail loss, Stable Diffusion aids in preserving essential features of the image.
In this method, variations in image brightness levels are evaluated and a diffusion process is employed to lessen these disparities. Stable Diffusion carries distinct advantages over traditional Gaussian noise reduction methods, including the retention of image details and enhancement of image quality that could otherwise be somewhat compromised with the use of simpler, low-pass filter techniques.
By incorporating the edge-stopping function, Stable Diffusion curtails diffusion across image edges, thereby preserving edges and other high-frequency components precisely. This aspect establishes Stable Diffusion as a potent instrument for noise reduction in digital imagery. It ensures maintenance of image quality while simultaneously eliminating noise.
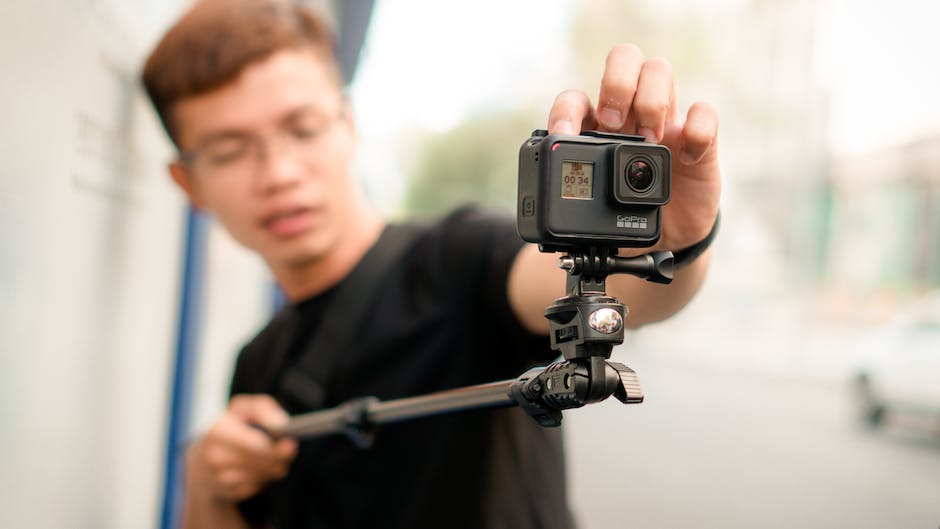
Introduction to Stable Diffusion
Grasping the Concept of Stable Diffusion
Stable Diffusion signifies a process that diffuses an image leading to a reduction in its inherent noise, while simultaneously safeguarding its prominent features. This method is categorized under the broad concept of partial differential equations (PDEs).
For a comprehensive understanding of stable diffusion, it’s critical to understand the idea of anisotropic diffusion, which is the pillar of the process. The method iteratively quelches insignificant details and augments relevant image structures.
Mathematical Interpretation of Stable Diffusion
The mathematical basis of stable diffusion lies in the heat equation, also known as anisotropic diffusion equation. This equation describes how the diffusion process evolves over time, similar to how heat diffuses through a medium.
By applying this equation to an image, the concept of heat over time becomes the concept of image intensity at a location. The equation involves taking the gradient of the image and diffusing it along the image’s gradient direction. This prevents a high change in image intensity, thus preserving edges and important image details while reducing random noise.
The Process of Stable Diffusion
In implementing stable diffusion, the image is first exposed to a single or several iterations of image blurring. This is done using the convolution operation, which combines points in the image with others around it. The result is a softened version of the original image, where high-frequency details are reduced.
The next step involves subtracting the blurred image from the original to create a “detail” image capturing the high-frequency elements, essentially the noise and edges. The detail image is then exposed to the stable diffusion equation, where the diffusion process reduces the noise present. The diffused detail image is then recombined with the blurred image, which effectively reduces the image’s noise while preserving its key features.
Advantages of Stable Diffusion
Stable diffusion technique’s primary advantage is that it reduces the random noise in an image while effectively preserving the edges and other significant details. This is contrasted with other common noise reduction techniques, such as Gaussian blur, which indiscriminately blurs all details whether they are important features or just noise.
Stable diffusion also allows for more user control over the noise reduction process. By adjusting parameters such as the number of diffusion iterations and the magnitude of diffusion, a user can customize the level of noise reduction to their needs. This technique can provide impressive noise reduction results on a variety of image types, making it a widely utilized tool in image processing.
Exploring the Applications of Stable Diffusion
Stable diffusion finds its relevance beyond basic image processing and is highly valued in various specialized sectors. For instance, in the realm of medical imaging, it serves as a critical tool to enhance the visibility of major features like tumors or blood vessels by minimizing the background noise. This plays a key role in facilitating better diagnosis and treatment planning.
Similarly, in the niches of remote sensing and satellite imaging, the role of stable diffusion to clarify geographical features is irreplaceable. It achieves this by mitigating environmental noise caused by atmospheric interference or sensor inaccuracies, resulting in more accurate mapping and surveillance. It is also worth mentioning that in the digital photography landscape, stable diffusion aids in minimizing sensor noise and simultaneously preserving essential image details, thus enabling the production of premium quality photographs.
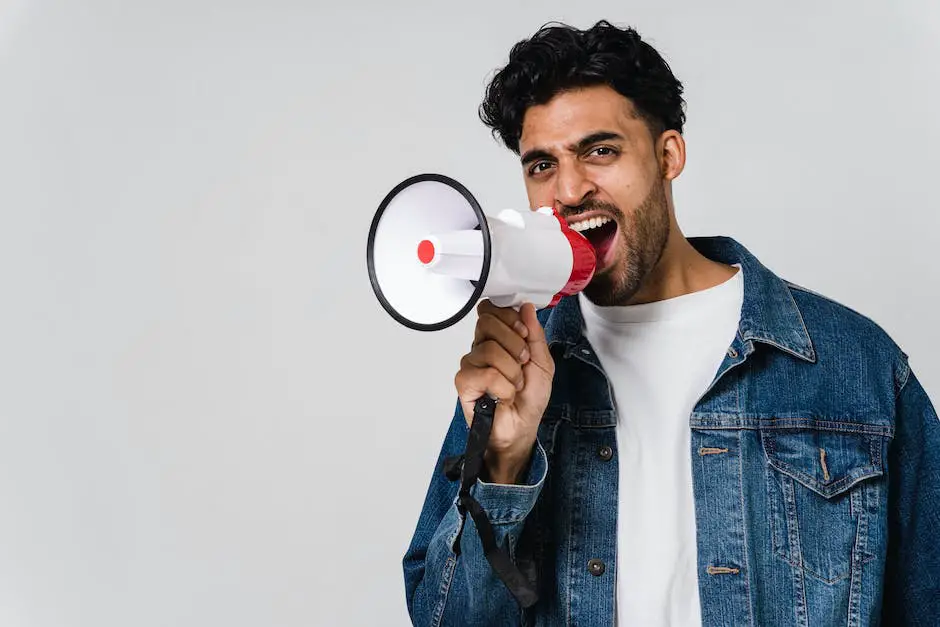
Stable Diffusion in Action: Noise Reduction Process
Digging Deeper into the Concept of Stable Diffusion
The concept of stable diffusion, also commonly referred to as anisotropic diffusion, encapsulates a sophisticated methodology. It employs partial differential equations to produce a uniformly smoothed image, while safekeeping the edges. It gains its ‘stable’ reputation because of the distinct conduction coefficient function it uses, which effectively curtails noise but avoids any potential image blurring.
How Stable Diffusion Functions
The functionality of stable diffusion hinges on the diffusion process that depends on the gradient of the image. Pixels in regions with high intensity differences, such as edges, have a lower diffusion rate; contrarily, pixels in areas with minimal intensity differences have a higher diffusion rate. This selective diffusion helps in smoothening the image while preserving its edge features.
The concept numerically, it scrutinizes the image and determines the gradient at each pixel. Greater gradients imply sharp changes, i.e., edges, and smaller gradients indicate smooth areas. The diffusion factor, determined by these gradient values, permits less diffusion at edges and more diffusion in smoother areas.
Stable Diffusion for Noise Reduction
The use of stable diffusion in noise reduction in images is due to its edge-preserving characteristics. Traditional filters, such as mean and median filters, tend to blur edges during the de-noising process. However, using stable diffusion, image details and edges can be preserved while reducing noise. It allows for selective diffusion based on image features, which leads to a more effective noise reduction without sacrificing important image information.
Practical Implementation of Stable Diffusion
Implementing stable diffusion for noise reduction involves a couple of iterations over the image. For each iteration, the gradient of the image is computed and the diffusion factor is determined. Pixels in the image are then updated based on the diffusion factor, allowing for selective smoothing of the image.
Real-world Example of Stable Diffusion
In real-world application, stable diffusion finds use in medical imaging. Noise presence can drastically impact the diagnosis, thus, stable diffusion is implemented for noise reduction. It helps in the preservation of important features, such as edges of tumors or lesions, while reducing random noise in the image. This reveals clearer, high-quality images that lead to more accurate diagnostic procedures.
Stable Diffusion Through Numerical Simulation
Numerical simulations of stable diffusion involve using matrix computation to simulate the diffusion process. As intensity gradients are calculated, the diffusion factor for each pixel can be determined. This simulation process assists in understanding how stable diffusion works step by step and can be used to demonstrate the efficacy of this method in real-world applications.
In essence, stable diffusion presents a highly effective mechanism for reducing noise within images. Its distinctive ability to simultaneously maintain crisp edges and diminish distractive noise makes it an apt solution for tasks requiring high levels of detail preservation, such as medical imaging.
Through the lens of numerical simulations, the methodologies and benefits intrinsic to this process are brought to light, demonstrating its ability to carry out noise reduction interventions in a more illustrative and efficient manner. Its inherent capacity to safeguard crucial edge information distinguishes it from conventional filters, heralding it as a more versatile and trustworthy paradigm in the realm of image noise reduction.
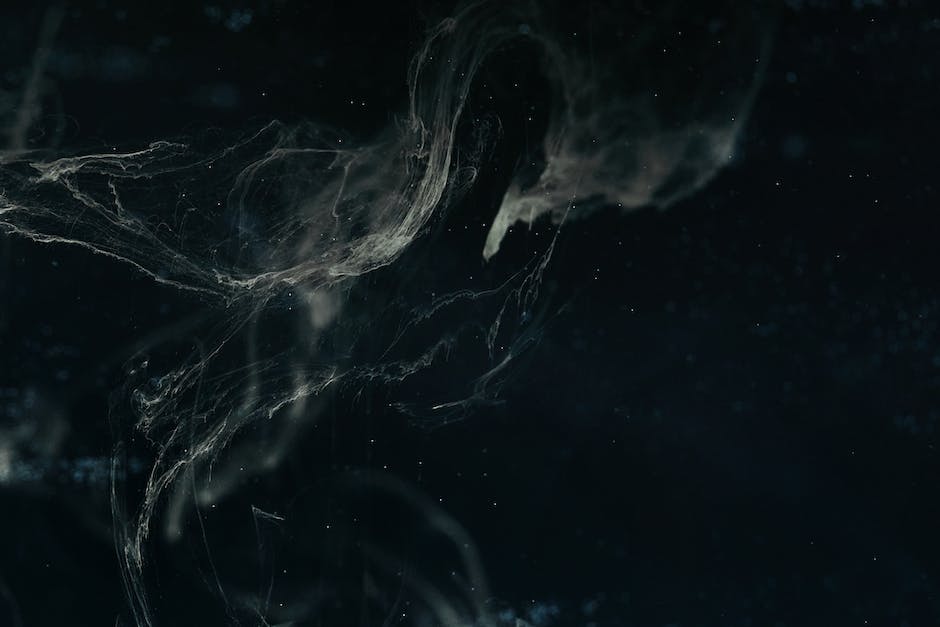
Advanced Techniques and Innovations in Stable Diffusion
Deciphering Stable Diffusion
Stable diffusion is a mathematical model deployed across a variety of spheres, image processing being a notable example. This method employs the use of a partial differential equation (PDE) aiming to diffuse an image in such a way that it manages to gradually minimize noise without compromising on edges and fine details. Traditional models of diffusion, like the Gaussian blur, tend to diminish edges over time, a critical shortcoming that stable diffusion efficiently counteracts. By establishing stable boundary conditions for image diffusion, noise is significantly diminished without forfeiting vital image content.
Advancements in Stable Diffusion Techniques
There has been a profound shift in the advancements of stable diffusion in the past years. Researchers have been exploring methods to enhance the process and further decrease the noise in images. Focusing on considerably innovative techniques in recent times, one of the methods that have been advanced is the concept of anisotropic diffusion where the diffusion coefficient is a function of pixel intensity gradient.
This anisotropic diffusion functions differently depending on the noise and edges in an image. The diffusion process is more vigorous in low contrast, high noise areas, whereas, in high contrast, it diffuses slowly preserving the edge details.
Recent Research and Applications in Stable Diffusion
Recently, there has been an increasing interest in the applications of stable diffusion in various image processing fields such as medical imaging, computer vision, and remote sensing. Stable diffusion techniques have been applied to enhance ultrasound images, helping in early detection of anomalies which less advanced techniques might miss. Also, in remote sensing, these techniques have been used to improve the quality of scanned images to identify geographical features precisely.
One of the most pioneering research published on stable diffusion was by Perona and Malik, who introduced a diffusion process that relies on the conductivity coefficient being a function of local gradient magnitude. This way, the edge-preserving capacity can be controlled with a higher degree of precision compared to traditional methods.
Advantages of Stable Diffusion in Noise Reduction
The advantages of using these advanced stable diffusion methods for noise reduction are numerous. The major benefit is their ability to preserve significant details in an image unlike classical noise reduction methods. These techniques work efficiently in maintaining textures, as well as the sharpness of edges while reducing random noise to a significant level. By preserving these key image details, the enhanced images lead to better outcomes in derivative works, be it in academic research, medical diagnosis, or remote sensing applications.
The groundbreaking methods of advanced stable diffusion techniques have proven to be an effective tool for noise reduction in images, finding many applications across various domains. The potential and wide-ranging uses of these methods indicate an optimistic future in the sectors of image processing and noise reduction.
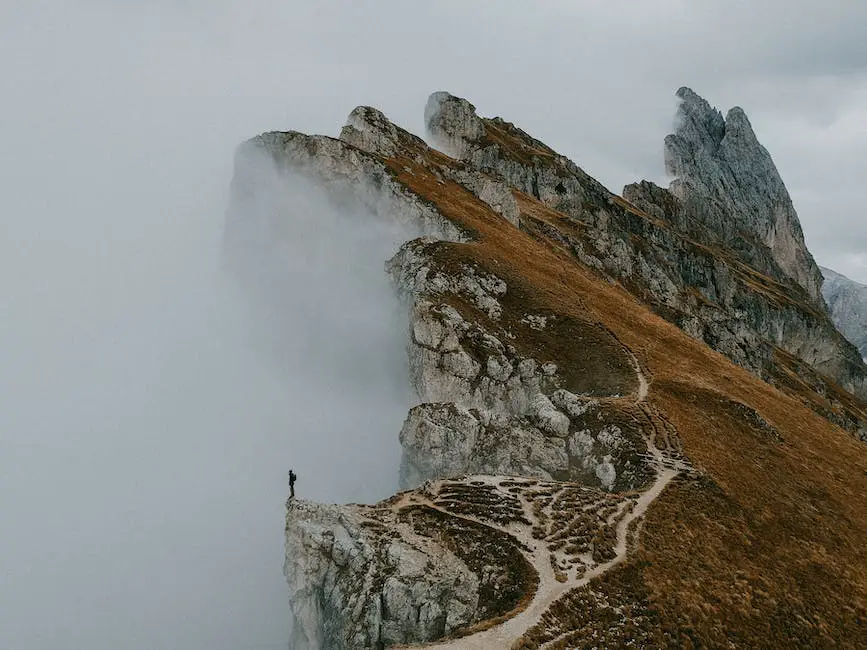
Practical Applications of Noise Reduction Using Stable Diffusion
Delving Deeper into the Concept of Noise in Images
When referring to imaging, noise is seen as random fluctuations in an image’s brightness or color information. This can significantly compromise the overall quality of the image. Different factors such as errors during data transfer or capture, the environment in which the image is captured, and the limitations of the sensor can be potential causes of noise. This noise in images typically manifests as Gaussian noise, Poisson noise, salt-and-pepper noise, and speckle noise.
Noise Reduction Using Stable Diffusion
Diffusion is a technique used in image processing aimed at reducing noise and other image inaccuracies. Stable diffusion, in particular, presents an effective method for reducing image noise. It works by simulating the process of heat transfer or diffusion on an image. The image is viewed as a heat distribution surface, where the image intensity at each point represents the temperature. The diffusion process aims to achieve a stable state, where no more heat transfer is possible. This process results in smoother transitions between different sections of the image and overall improved image quality.
Application in Healthcare: Medical Imaging
The application of stable diffusion in medical imaging is highly significant. High-quality imaging is essential for accurate diagnosis and treatment planning. Noise reduction using stable diffusion can enhance the visibility of fine details in medical images such as MRI scans, X-rays, or ultrasound images. For example, in MRI scans, stable diffusion can help reduce the speckle noise typically present due to the magnetic field variations, improving the visibility of tissues and organs.
Application in Satellite Imaging
Noise reduction using stable diffusion is valuable in satellite imaging as well. Satellite images often contend with issues such as atmospheric interference and sensor limitations that introduce noise, affecting their clarity and accuracy. Stable diffusion can be used to reduce this noise and enhance the image’s overall quality. Such improved images are crucial for meteorology, geology, mapping, resource exploration, and other areas reliant on accurate satellite data.
Application in Photography
In the field of photography, noise reduction using stable diffusion can help enhance photographs’ visual appeal and clarity. This technique can help eliminate graininess caused by high ISO settings, low light conditions, or limitations of the camera sensor, resulting in clearer, sharper images. It can be particularly useful in long exposure photography, where noise can become more prominent.
The Role of Stable Diffusion and Its Impact
In summary, stable diffusion plays an integral role in reducing image noise and improving image quality across various sectors. It helps reveal finer details in images that could otherwise be obscured due to noise, leading to more accurate data interpretation and decision making in essential fields like healthcare and geology. By reducing noise and enhancing image quality, stable diffusion has revolutionized how we capture, process, and perceive images.
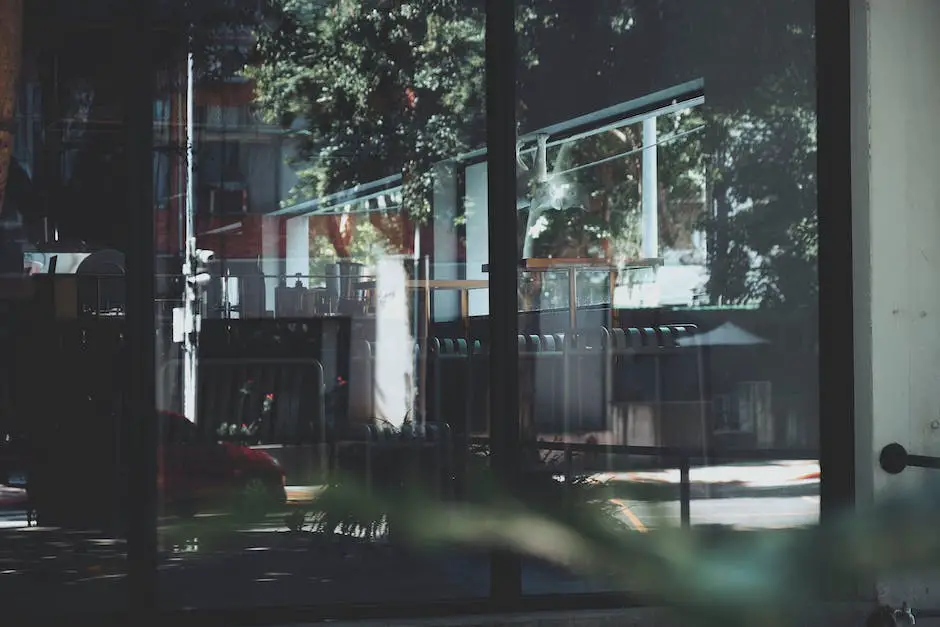
The journey explored herein, reflects the potential of Stable Diffusion as a tool for noise reduction in digital images, an asset that enhances multi-disciplinary fields. From understanding the basics of image noise and its effects to discussing the process detail of noise reduction using stable diffusion, the expedition only establishes and reiterates the invaluable contribution Stable Diffusion holds.
The more we explore, understand and innovate, the closer we are to achieving near perfect digital imagery. Through continuous research and development in this direction, the day isn’t far when achieving the highest image clarity irrespective of the noise present, becomes the norm of the day, contributing significantly to the advancement of technology and human life.
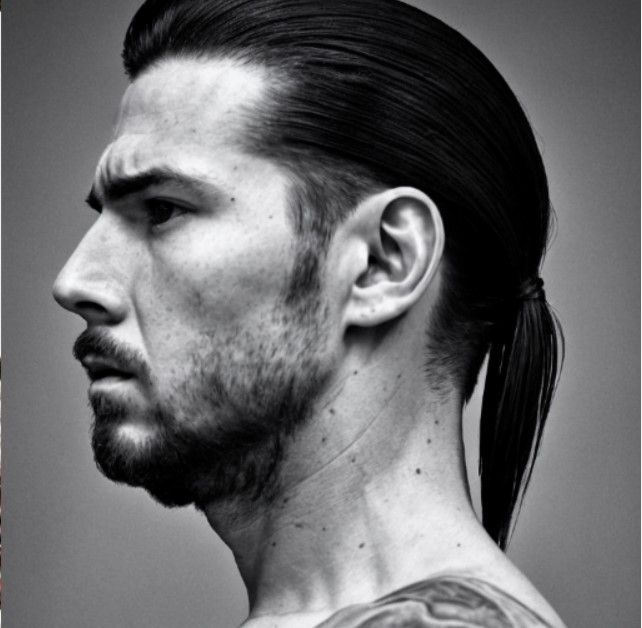
Emad Morpheus is a tech enthusiast with a unique flair for AI and art. Backed by a Computer Science background, he dove into the captivating world of AI-driven image generation five years ago. Since then, he has been honing his skills and sharing his insights on AI art creation through his blog posts. Outside his tech-art sphere, Emad enjoys photography, hiking, and piano.