Latent Diffusion Models (LDMs) have emerged as a powerful technique, revolutionizing the way we approach image synthesis and modification. LDMs offer unparalleled flexibility, efficiency, and quality, making them a significant advancement in the field of AI Imagery.
In this article, we embark on a deep dive into LDMs, unravelling their inner workings, exploring their applications, and demystifying the transformative impact they have on the creation of visually compelling images.
Contents
Understanding Latent Diffusion Models (LDMs)
Sequential Application of Denoising Autoencoders
LDMs leverage the sequential application of denoising autoencoders to decompose the image formation process. By iteratively removing noise and refining the image, LDMs achieve state-of-the-art synthesis results surpassing traditional methods.
This dynamic approach allows for superior image quality and enhanced visual fidelity, setting LDMs apart as a powerful tool in AI Imagery.
Image Modification without Retraining
One of the most fascinating aspects of LDMs is their ability to modify images directly without the need for retraining. Unlike traditional methods that require extensive retraining for each modification task, LDMs offer the convenience of inpainting, super-resolution, and other image modifications with ease.
The Stable Diffusion technique employed by LDMs enables efficient image modification, providing creative minds the freedom to shape and enhance images effortlessly.
Advantages of Latent Diffusion Models (LDMs)
Computational Efficiency and Resource Optimization
Powerful image synthesis often demands extensive computational resources. However, LDMs have stepped up to the challenge by introducing a novel approach to training.
By leveraging the latent space of pretrained autoencoders, LDMs retain exceptional quality and flexibility while significantly reducing the computational requirements. This breakthrough makes high-quality synthesis accessible even with limited computational resources, opening doors to a wider range of applications.
Enhanced Visual Fidelity and Realism
LDMs strike a delicate balance between complexity reduction and spatial downsampling, resulting in enhanced visual fidelity and realism.
By training diffusion models in the latent space, LDMs preserve intricate details and capture the underlying patterns, leading to visually compelling and realistic images. The latent space modeling in LDMs allows for the generation of high-resolution, visually appealing imagery, transforming the way we perceive and interact with AI-generated content.
Applications of Latent Diffusion Models (LDMs) in AI Imagery
Unconditional Image Generation
LDMs excel in unconditional image generation, allowing for the creation of diverse and visually stunning images. The sequential application of denoising autoencoders in LDMs unleashes the creative potential of AI Imagery enthusiasts, providing them with a powerful tool to generate images beyond the limitations of traditional methods.
Inpainting and Image Restoration
With LDMs, the process of inpainting and image restoration becomes seamless. The Stable Diffusion technique employed by LDMs enables efficient and effective removal of unwanted elements from images or restoration of missing parts, resulting in visually coherent and realistic outcomes.
Super-Resolution and Enhanced Image Quality
LDMs offer significant advancements in super-resolution, allowing for the generation of high-quality, detailed images from low-resolution inputs. By leveraging the power of denoising autoencoders, LDMs enhance image quality and restore missing details, enabling the creation of visually stunning, high-resolution imagery.
Conclusion about Latent Diffusion Models
In this deep dive into Latent Diffusion Models (LDMs), we have unveiled the inner workings and explored the transformative impact they have on the field of AI Imagery. LDMs offer a powerful approach to image synthesis and modification, providing unparalleled flexibility, efficiency, and quality.
By leveraging the sequential application of denoising autoencoders, LDMs have unlocked new possibilities in unconditional image generation, inpainting, super-resolution, and more. As we continue to push the boundaries of AI Imagery, LDMs stand at the forefront, revolutionizing the way we create, modify, and experience visually compelling images.
Frequently Asked Questions (FAQ)
Q1: What are Stable Diffusion models?
A1: Stable Diffusion models are a groundbreaking technique in AI Imagery that decompose the image formation process into sequential applications of denoising autoencoders. They offer superior image synthesis results by minimizing semantically meaningless information and enhancing visual fidelity.
Q2: How do Latent Diffusion Models (LDMs) differ from traditional models?
A2: Latent Diffusion Models (LDMs) introduce a new approach to image synthesis and modification. Unlike traditional models, LDMs allow for direct image modification without retraining and achieve high-quality results with reduced computational requirements.
Q3: What is the advantage of training LDMs in the latent space?
A3: Training LDMs in the latent space of pretrained autoencoders enables retaining exceptional quality and flexibility while reducing computational requirements. This breakthrough makes high-quality synthesis accessible even with limited resources.
Q4: What are the applications of LDMs in AI Imagery?
A4: LDMs have a wide range of applications, including unconditional image generation, inpainting, super-resolution, and image restoration. They empower AI Imagery enthusiasts to create diverse and visually stunning images with enhanced visual fidelity.
Q5: How do LDMs enhance visual fidelity and realism?
A5: LDMs strike a balance between complexity reduction and spatial downsampling, preserving intricate details and capturing underlying patterns. This leads to visually compelling and realistic images, transforming the way we perceive and interact with AI-generated content.
Q6: Can LDMs generate high-resolution images?
A6: Yes, LDMs can generate high-resolution images by leveraging their latent space modeling and the sequential application of denoising autoencoders. They excel in super-resolution tasks, producing detailed and visually appealing imagery from low-resolution inputs.
Q7: Are LDMs suitable for image modification tasks?
A7: Absolutely. LDMs enable efficient and effective image modification without the need for retraining. They excel in tasks like inpainting, where unwanted elements can be removed, and missing parts can be restored seamlessly.
Q8: How do LDMs contribute to computational efficiency in AI Imagery?
A8: LDMs optimize computational resources by training in the latent space and reducing the computational requirements. This allows for high-quality synthesis even with limited resources, making LDMs a valuable tool for AI Imagery professionals.
Q9: What is the future of Stable Diffusion Research?
A9: Stable Diffusion Research is an evolving field, with ongoing innovations and advancements. Researchers are exploring novel techniques, conditioning mechanisms, and ensemble models to push the boundaries further and open up new frontiers in AI Imagery.
Q10: How do LDMs contribute to the creative potential of AI Imagery enthusiasts?
A10: LDMs unlock the creative potential by providing a powerful tool for image generation and modification. With LDMs, AI Imagery enthusiasts can explore new avenues, create visually stunning images, and shape their artistic vision with ease.
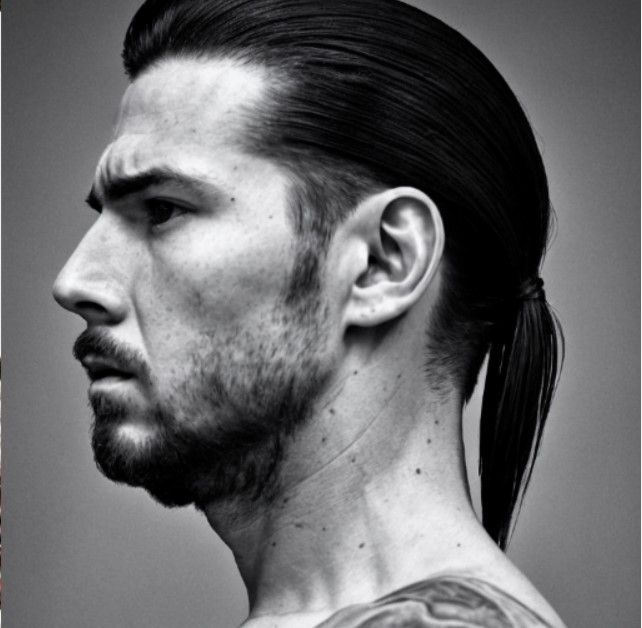
Emad Morpheus is a tech enthusiast with a unique flair for AI and art. Backed by a Computer Science background, he dove into the captivating world of AI-driven image generation five years ago. Since then, he has been honing his skills and sharing his insights on AI art creation through his blog posts. Outside his tech-art sphere, Emad enjoys photography, hiking, and piano.