In the ever-evolving realm of digital imaging and information technology, one aspect that consistently garners significant attention is Image Restoration, more so when high-resolution image synthesis comes into play. This critical field of study intersects computer science, artificial intelligence, and graphic design, propelling endless possibilities and solutions to previously intractable challenges. The myriad applications spanning scientific research, academia, and real-world scenarios underscore its significance in today’s technology-driven landscape. Through exploring fundamental principles, sophisticated techniques, practical implementation, and peeking into potential future advancements, we delve into the transformative power of high-resolution image synthesis in image restoration, charting a course of understanding that is both comprehensive and profound.
Contents
Foundations of Image Restoration
In the in-depth exploration of modern science and technology, image restoration encompasses a significant area of interest.
This pivotal process allows for the improvement and recuperation of images which may be degraded due to several factors, making it a keystone in various domains.
Image restoration provides a robust means to recover and enhance the quality of images. Various factors such as noise, blurriness, and interferences can often deplete the quality of an image, rendering it unreadable or unclear. Such a process then operates to eliminate or reduce these deplorable factors and, as a result, significantly increases the overall readability and understanding of images.
A noteworthy application of image restoration lies within the realm of medical imagery. In procedures like magnetic resonance imaging (MRI) or computed tomography (CT), for instance, it is common for images to suffer from a degradation of quality due to patient movement or hardware functionality. Image restoration, in these instances, is invaluable as it aids in providing a clearer, detailed picture, thereby improving disease diagnoses and patient treatment plans.
Astrophysics is another domain where image restoration is indispensable. The images gathered from telescopes are often blurred and distorted due to atmospheric turbulences and instrumental noise. Using image restoration techniques, astrophysicists can significantly enhance these distant celestial images. This process, therefore, plays a critical role in our understanding of the universe and the various phenomena occurring in it.
On the technological front, image restoration finds utility in surveillance systems, where clear images are paramount for accurate identification and tracking. It also bears significance in driver assistance systems, virtual reality, and augmented reality applications, where the restoration of images can substantially improve system functionality and user experience.
Furthermore, image restoration holds key importance in the realm of historical document preservation. Old texts, maps, and photographs that have faded over time can be rejuvenated using image restoration techniques, a process critical in preserving our cultural heritage and elucidating past events and practices.
While each application may require variants of methods and algorithms depending on the specific cause of degradation, the primary objective of image restoration remains invariably consistent – to significantly improve the quality of the original image, augmenting its utility and value.
In the grand scheme of the scientific realm, image restoration plays a critical role, facilitating not only the accessibility but also the utility of image-based data. Consequently, it has an impact on the advancement in medical technology, astronomical discoveries, surveillance, and the preservation of historical artifacts.
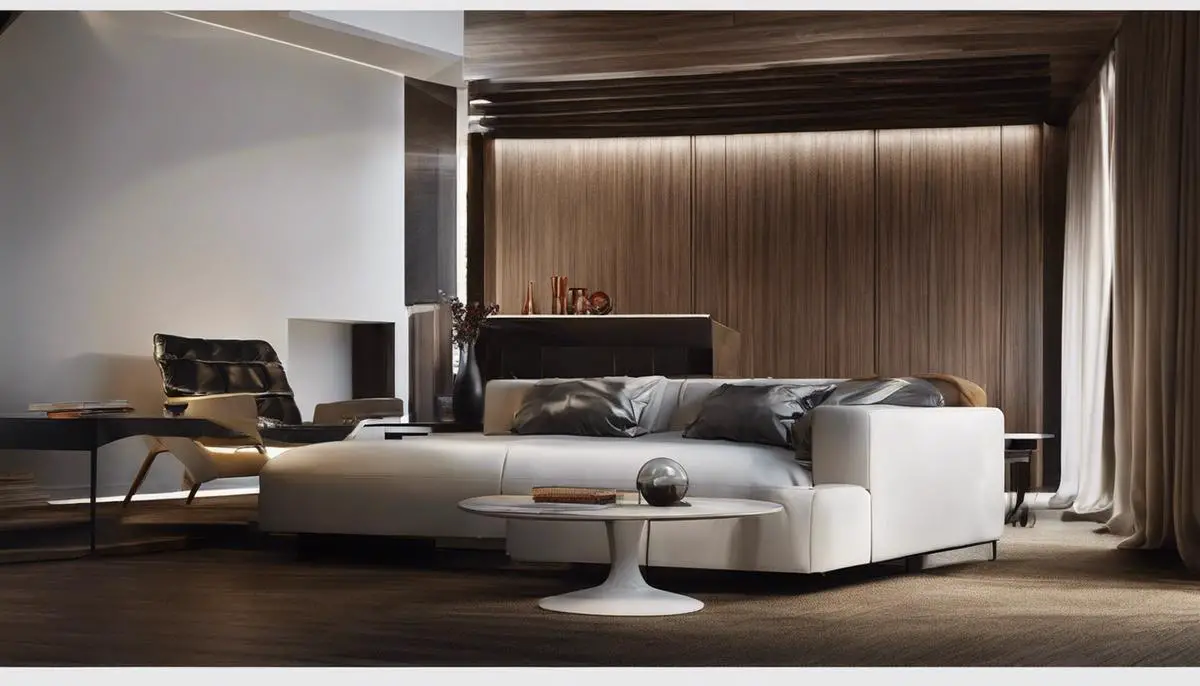
Principles and Techniques in High-Resolution Image Synthesis
A comprehensive understanding of high-resolution image synthesis requires a deep dive into the key principles and methods that are inherent to its functioning. While image restoration presents ways to recover and enhance degraded image quality, a complementary aspect of this process is image synthesis, which builds entirely new images that are nonetheless realistic and consistent with the observable world. Image synthesis finds its applications in a myriad of fields, encompassing computer graphics, artificial intelligence (AI), virtual reality, and beyond.
To delineate the process of image synthesis, it’s crucial to understand the role of Generative Models first. These are essentially algorithms that learn from an existing dataset and generate new instances based on this learning. Primarily, image synthesis leverages Generative Adversarial Networks (GANs) for creating synthetic images.
GANs operate in a two-part structure. The generator network creates images and presents them to the discriminator network, which attempts to differentiate these new images from a set of actual, real-world images. The process of feedback and learning from the discriminator encourages the generator to refine the output, constantly tweaking the next set to be more realistic.
Another central paradigm of image synthesis is accomplished through Convolutional Neural Networks (CNNs). CNNs, specially designed to process image data, make use of a mathematical operation called convolution in at least one of their layers. Convolution replaces the input pixels with linear combinations of themselves, much like a blending operation. This has the effect of applying a filter that’s particularly adept at identifying complex features in images. Applying this process to image synthesis allows the creation of detailed and tactically sound artificial images.
Increasingly evident is the flexibility of CNNs and GANs, applied together, to yield amazing results in tasks such as transforming day images to night, synthesising high-resolution images from sketches, and even producing photorealistic images from textual descriptions. This can significantly enhance details in virtual reality, augment surveillance practices, and even create realistic virtual characters in video games.
The combination of image restoration and image synthesis has profound implications for our interaction with visual information in the world. High-resolution image synthesis, especially, holds vast potential – from painting a more complete picture of the universe to potentially unlocking new levels in disease diagnosis, it stands at the forefront of scientific advancements. Knowledge and exploration in this field possess the capacity to shape and revolutionize our future, whether in practical applications or novel scientific pursuits. Infusing the intricate science behind these processes with the relentless curiosity pushing us further only suggests that the possibilities are indeed limitless.
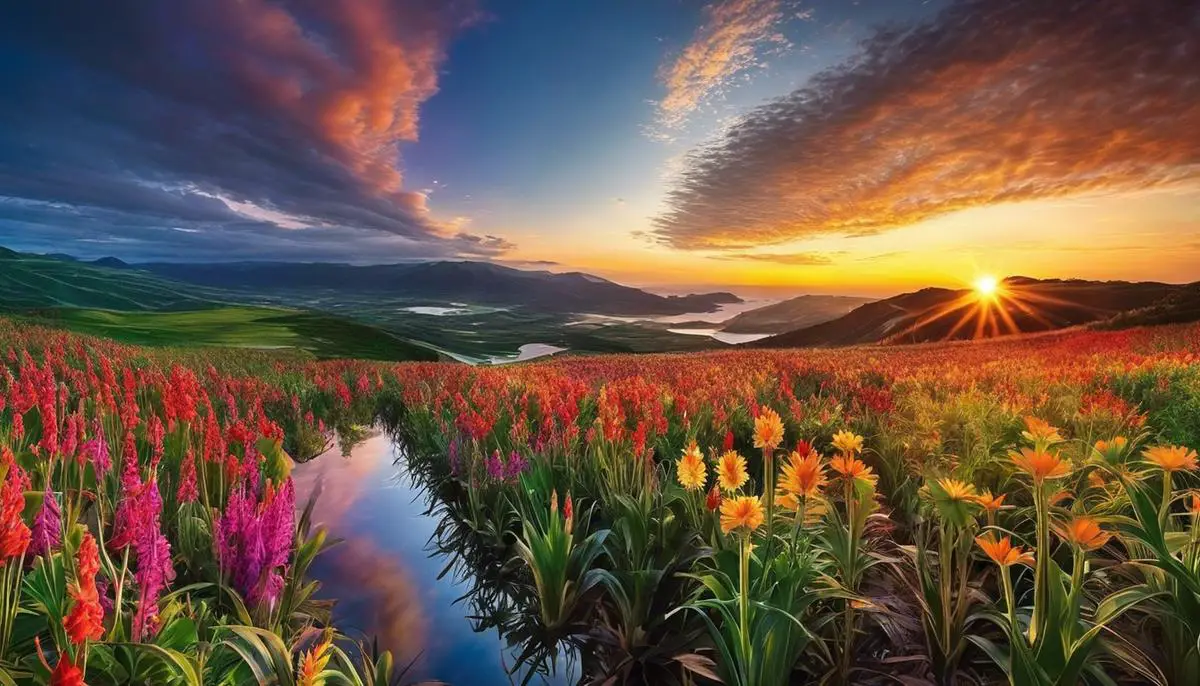
Implementation of High-Resolution Image Synthesis in Image Restoration
Plunging deeper into the techniques of Image Restoration, this article addresses a key aspect: implementing High-Resolution Image Synthesis. This technique primarily revolves around restoring and enhancing the quality of degraded images, using state-of-the-art methods such as Generative Models, specifically Generative Adversarial Networks (GANs), and Convolutional Neural Networks (CNNs).
Generative Adversarial Networks, or GANs, are a type of artificial intelligence algorithms used in high-resolution image synthesis. It consists of two parts: the generator, which creates new images, and the discriminator, which determines if those images are authentic or artificial. By having these two aspects ‘competing’ against each other, the system refines its ability to create high-quality syntheses. This fascinating ‘adversarial’ relationship facilitates the process of image restoration by aiding the generation of detail-rich, high-definition images.
Conversely, Convolutional Neural Networks (CNNs) play a significant role in classifying and interpreting images. These networks have layers that act like filters, designed to amplify certain features within an image whilst suppressing others. This allows the distinct attributes of an image to be prominently featured, enabling more nuanced image restoration.
The implementation of CNNs and GANs in high-resolution image synthesis offer robust frameworks for image restoration. For instance, the efficient conversion of day images to night and the synthesis of high-resolution images from sketches are prime examples depicting the unparalleled capabilities of these networks. They capably manage complex transformations, adding or removing details while maintaining the overall structure of the image, allowing for the restoration even when original details might be lacking or distorted.
The power of these models not only lies in their ability to generate photorealistic images from textual descriptions but also in unlocking new paradigms in image restoration. Hence, the implications of image restoration and synthesis stretch far beyond just enhancing degraded imagery. These technologies introduce new ways of creating digital art, improving computer graphics, and even assisting scientific progress, say in accurately visualizing complex geological formations for environmental science studies or prototyping in 3D printing.
Practical applications and scientific advancements in image synthesis continue to remodel computational photography and digital image processing landscapes across diverse sectors. Industrial design, advertising, film, gaming, fashion – the list is continually expanding. Each restored or synthesized image invites exciting opportunities for advancements in science, technology, and art, signaling an exciting juncture in the journey of artificial intelligence and image processing research.
In conclusion, high-resolution image synthesis fuels progress in the realm of image restoration. The techniques utilized, particularly the strength of generative models like GANs and CNNs, illuminate the astounding power of artificial intelligence to restore, enhance, and synthesize images, marking a meticulous yet exciting pursuit in the vast sphere of scientific exploration.
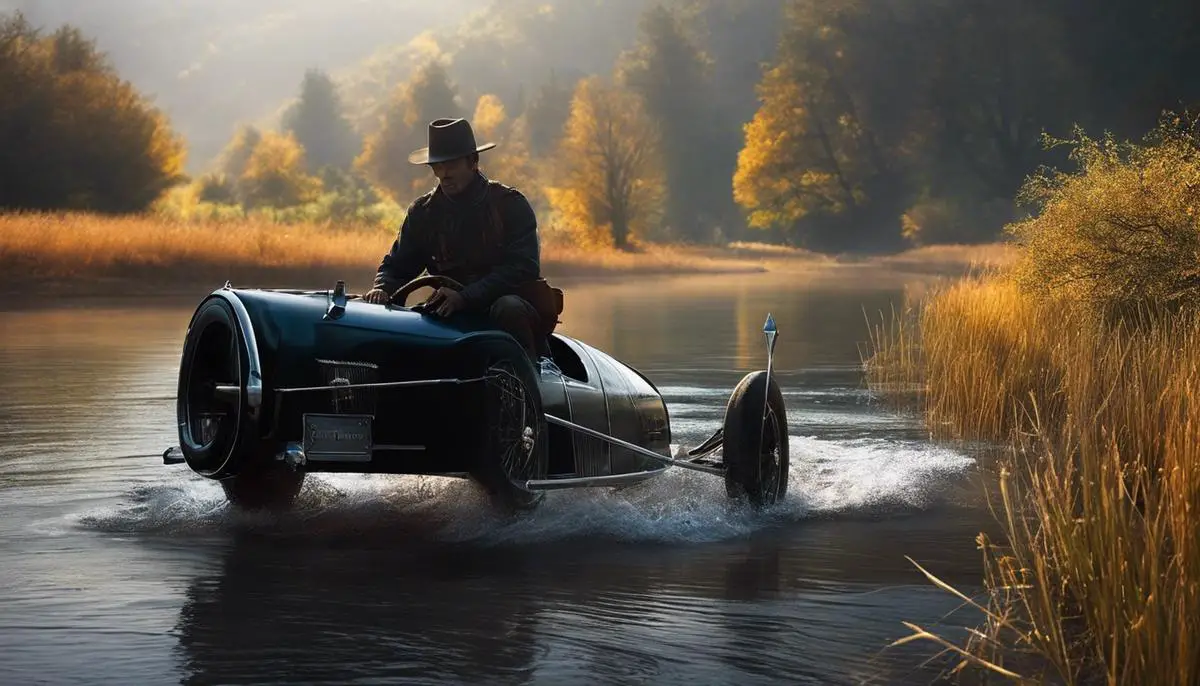
Challenges & Future Directions in High-Resolution Image Synthesis for Image Restoration
Commensurate with the impressive strides the scientific community has made in the field of image restoration and high-resolution image synthesis, there continues to be an array of substantial challenges to battle, as well as promising future directions to explore. A comprehensive understanding of these contemporary obstacles and prospects is crucial for both scientists and technologists who wish to further research in this pivotal domain of study.
One significant challenge that hampers progress in high-resolution image synthesis for image restoration is the problem of scale. High-resolution images contain significantly more information and detail than their low-resolution counterparts do. Therefore, the computational demands for processing these images, whether for restoration or synthesis, escalate exponentially with increasing resolution. This substantial computational load requires more advanced hardware capabilities and sophisticated algorithms for efficient processing — a substantial hurdle given present technology.
The second challenge lies in overcoming the inevitable discrepancies that occur during the transformation from a low-resolution, or degraded, image to a high-resolution one. These discrepancies result in perceptible deviations in the final image output, creating an unnatural appearance that fails to realistically represent the original, pristine image. Mastering the nuances of eliminating these imperfections is critical for achieving a truly successful image restoration and a major challenge in the realm of high-resolution image synthesis.
Dovetailing with the above challenges, a major hurdle is to generate high-quality and realistic textures in the high-resolution details during the restoration process. Mere amplification of the spatial resolution does not guarantee the preservation or enhancement of texture details, which is particularly crucial for specialist applications, such as medical imaging, astrophysical imaging, and historical document preservation where detailed texture is vital.
As daunting as these challenges may seem, they serve as a foundation for novel and exciting research directions in the realm of high-resolution image synthesis for image restoration. Advances in artificial intelligence and machine learning, particularly deep learning, hold considerable promise for addressing these challenges. For instance, the crafting of sophisticated deep learning algorithms, such as improved GANs, super-resolution CNNs, or hybrid models, could revolutionize the way high-resolution images are synthetized and restored.
Moreover, the future direction of research is not restricted to purely technical terrain. As developments in this field intertwine more deeply with human lives, concerns about ethical implications, privacy, and security will need to burgeon as prominent areas of exploration. Work on creating robust frameworks for ethical decision-making, data privacy, and secure networks will form a key part of future research in image restoration and synthesis.
Furthermore, the ever-increasing use of high-resolution image synthesis in diverse fields, such as surveillance systems, driver assistance systems, virtual reality, augmented reality, industrial design, advertising, and film, offers intriguing avenues to explore and opens doors for interdisciplinary research.
However, the greatest scope for advancement could well be the integration of high-resolution image synthesis techniques with other rapidly developing fields—consider, for example, marrying high-resolution image restoration to developments in 3D printing or complex geological visualization. Such intersections would represent a truly fertile ground for generating synergy that could transform how we see, interpret, and display the world around us. The much-anticipated amalgamation of cutting-edge spheres of digital innovation holds the promise of staggering scientific advancements in the years to come.
In conclusion, the challenges in high-resolution image synthesis for image restoration are monumental but surmountable, and the future directions are as vast as they are diverse. The scientific exploration of these issues is far from complete, and the promise of what lies ahead undeniably exhilarating. The fruition of this formidable quest promises a future endowed with scientific and technological mastery over visual perception and representation — a future that is worth passionately pursuing.
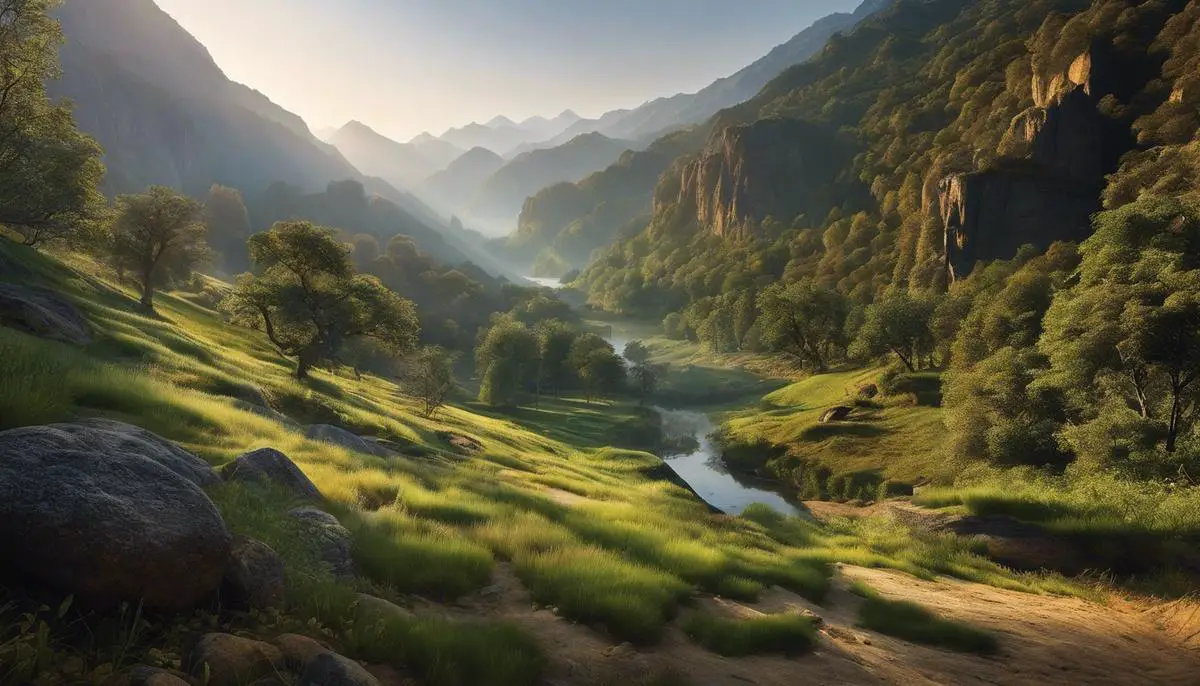
High-resolution Image Synthesis and Image Restoration are exciting areas that simultaneously challenge and enthrall professionals, driving progress and innovation in multitude directions. Current technological constraints, while indeed posing difficulties, also pave the way for new opportunities, strategies, and resolutions to emerge. Moreover, the incessant demand for high-quality image renewal makes this field both vital and dynamic. The future glittering with possibilities, a combination of technical acumen, capacity for innovation, and the unwavering willingness to confront challenges head-on will shape the direction and success of the high-resolution image synthesis for image restoration. Thus, the journey towards mastery in this fascinating confluence of art and technology is as thrilling and rewarding as the power and potential it heralds for our digital future.
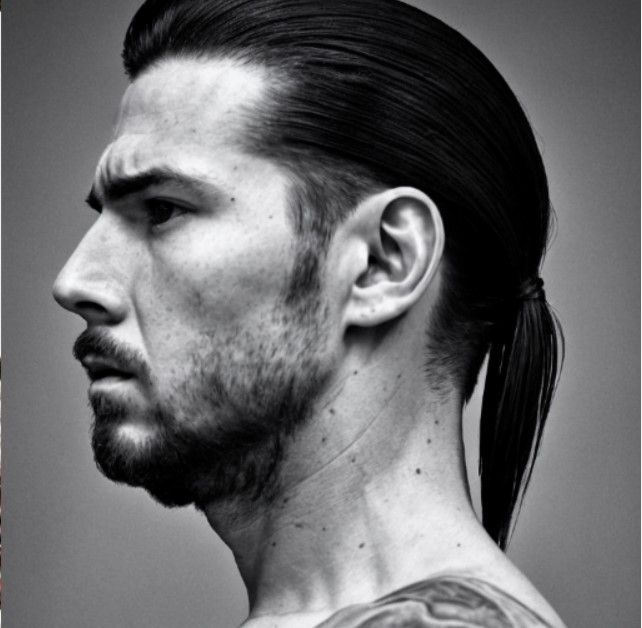
Emad Morpheus is a tech enthusiast with a unique flair for AI and art. Backed by a Computer Science background, he dove into the captivating world of AI-driven image generation five years ago. Since then, he has been honing his skills and sharing his insights on AI art creation through his blog posts. Outside his tech-art sphere, Emad enjoys photography, hiking, and piano.