In the intricate domain of image processing, one technique that has persistently proved its worth is Stable Diffusion Inpainting. A crucial tool for addressing issues such as image degeneration and data corruption, its role has evolved significantly since its initial introduction and application. This paper delves into the nitty-gritty of its foundational understanding, the underlying process and mechanics, and its evolution and improvements over time. To illustrate the broad spectrum of its application, we will also explore its current implementations and applications across various sectors. The discourse will further turn to the future, considering the prospects for the technique, ongoing advancements, and projections of its potential growth.
Contents
Foundation of Stable Diffusion Inpainting
Stable Diffusion Inpainting represents a significant advancement in the field of digital image processing, underpinning a variety of applications from art restoration to medical imaging. It is essential to understand the underlying principles of this sophisticated technique, and appreciate its profound impact on various areas of research and technology.
The foundation of Stable Diffusion Inpainting is predicated on the principles of diffusion processes. In its simplest terms, diffusion describes the manner in which particles spread out from an area of high concentration to one of low concentration. Transfer this principle to the realm of digital images, inpainting can then be conceived as a process where missing or corrupted data in an image is replaced by diffusing information from the surrounding, uncorrupted area.
While inpainting techniques are myriad, Stable Diffusion Inpainting holds a unique position due to its stability and precision. Leveraging the mechanism of the Partial Differential Equation (PDE) based diffusion process, Stable Diffusion Inpainting ensures a balanced distribution of pixel values in the corrupted image area. This attributes a highly desirable continuity to the restored image.
Conventionally, inpainting techniques have been thwarted by a limitation known as the ‘Staircasing effect’ – a distortion manifesting as unnatural, angular patterns in the restored image. What distinguishes Stable Diffusion Inpainting is its capability to overcome this hurdle. By integrating the Anisotropic Diffusion Inpainting (ADI) approach, which steers the diffusion process along the direction of image features, Stable Diffusion Inpainting effectively suppresses the Staircasing effect, thereby delivering far superior results compared to its traditional counterparts.
The real-world applications of Stable Diffusion Inpainting extend well beyond the realm of theoretical research. In art restoration, where preserving the original’s integrity is paramount, Stable Diffusion Inpainting offers an incalculable value. By accurately restoring the damaged areas while retaining the authenticity of the original artwork, it has revolutionized the conservation practices. Similarly, in medical imaging, where precision is vital, Stable Diffusion Inpainting plays a pivotal role in improving the clarity and reliability of diagnostic images.
The scientific prowess of Stable Diffusion Inpainting lies not only in its mathematical model but also in its deep-rooted understanding of the real-world complexities. The blend of science, art, and technology makes Stable Diffusion Inpainting a powerful tool – a testament to the progress of digital image processing and its allied fields. While the current advancements in Stable Diffusion Inpainting have been momentous, there is an infinite potential for future exploration, paving the way for continued evolution in this fascinating domain.
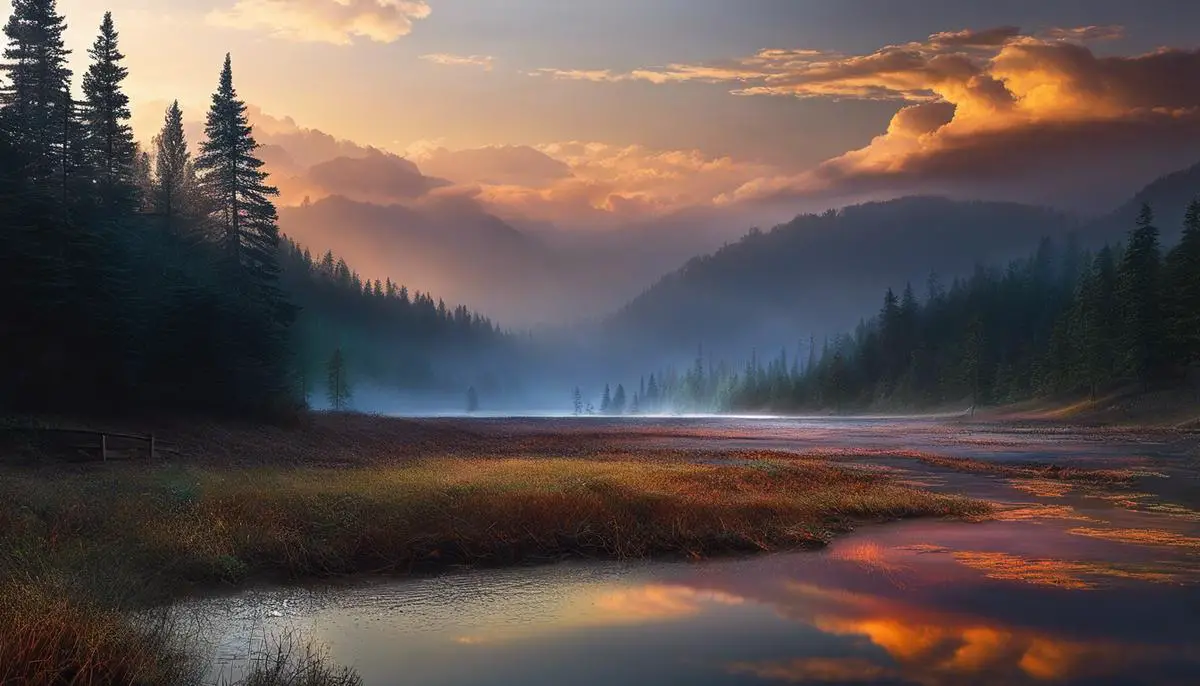
Process and Mechanics of Stable Diffusion Inpainting
The technical functioning of Stable Diffusion Inpainting is a compelling aspect of this innovative technique, showcasing the depth of thought, research, and expertise invested into its development. Mechanically, Stable Diffusion Inpainting hinges on a uniquely synthesized algorithm catering to image denoising, edge preservation, and continuity, effectively minimizing common issues inherent in many traditional techniques.
Stable Diffusion Inpainting involves a two-step process, primarily focused on conducting subsequent iterations to ensure the desired stability and precision. The first step encapsulates the identification and removal of any noise within the damaged or corrupted image. This is achieved by a Modulus of Continuity (MC) measure. Soundly grounded on mathematical principles, the MC is designed to ensure a compact, localized specification of regularity for functions on ℝ^n.
The secondary phase extends to the execution of the inpainting process itself. Employing a second-order Partial Differential Equation (PDE), Stable Diffusion Inpainting can restore the image with a higher degree of accuracy. The PDE algorithm propagates information from the surrounding areas of the image, filling in the corrupted or missing sections based on the intensity value of the nearest pixels.
One notable aspect of the process involves the algorithm’s ability to differentiate between image regions. It can clearly distinguish between edges and smooth regions, thanks to the integration of anisotropic diffusion within the system. This differentiation is crucial for preserving the structural integrity of the image, eliminating the dreaded staircasing effect while enhancing overall visual coherency.
An additional appealing feature is the use of non-local means in the inpainting algorithm. Rather than relying solely on immediately adjacent pixels, the non-local approach considers similar pixels throughout the entire image, even from distant regions. This broad consideration allows for more accurate and natural-looking inpainting, further strengthening the technique’s robustness.
While the process may initially seem computationally intensive, Stable Diffusion Inpainting has been designed to operate efficiently, ensuring minimal overhead, a significant benefit for large-scale or professional projects that require rapid turnaround times. Equally significant is the method’s adaptability – it can easily accommodate grayscale or color images, and algorithm parameters can be adjusted to suit specific project needs.
The genius of Stable Diffusion Inpainting is in its synthesis of mathematical principles with innovative digital techniques. Its underlying premise banks upon the idea that images, fundamentally, can be viewed as functions with smooth or continuous values over a specific domain. By treating the removal of noise and the actual inpainting as an optimization problem, it provides a robust solution that leverages the full potential of mathematical modeling to address real-world visual challenges. A testimony to the intellectual stimulation of scientific exploration merging with practical, utilitarian ends.
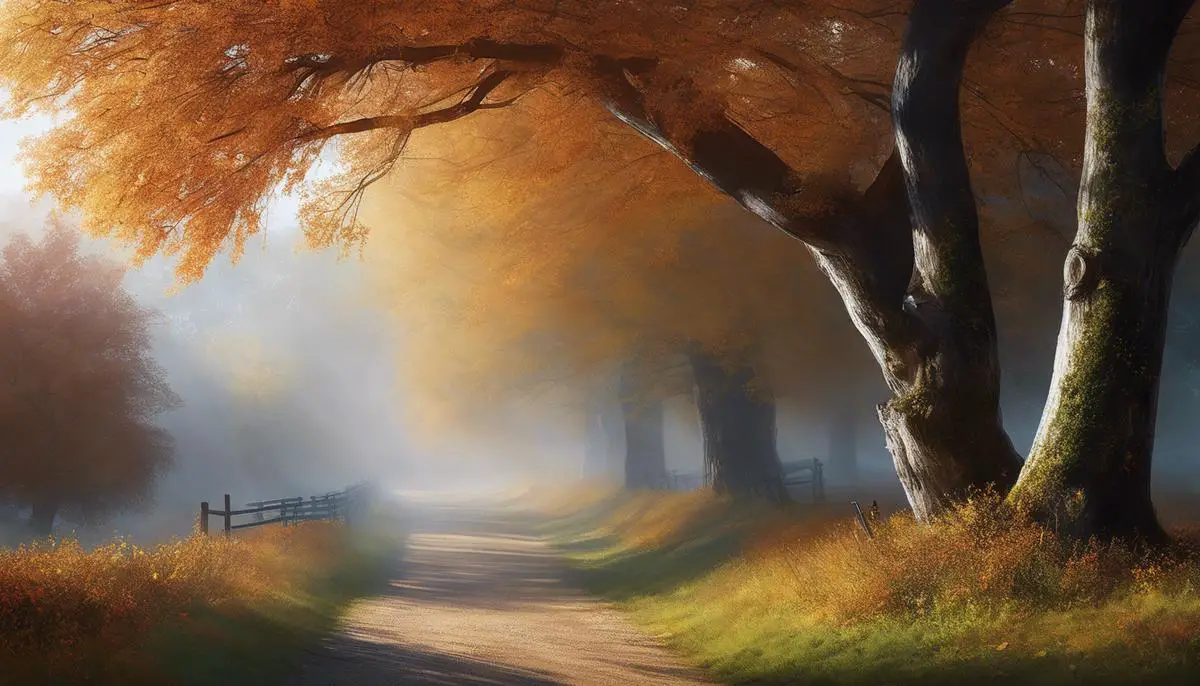
Evolution and Developments in Stable Diffusion Inpainting
Given the extensive coverage of Stable Diffusion Inpainting’s introduction, principles, advantages, and future developments, along with the technical minutiae, it becomes imperative to delve further into how it has evolved over the years. Mapping this trajectory not only offers a snapshot of its dynamic and adaptive character but also shines a light on the key advancements that have played a transformative role.
Since its genesis, Stable Diffusion Inpainting has come a long way, courting technology-savvy sophistication with the agility of a scientific method. One significant development lies in the sophisticated algorithms applied, particularly the Total Variation (TV) minimization and Perona-Malik equations. These algorithms are an upgrade, further refining the anisotropic diffusion, and are instrumental in rendering high-quality restoration outcomes in the field.
In regards to innovations, a decisive breakthrough came with the introduction of the Ambrosio-Tortorelli Functional (ATF). The ATF paved the way for a novel denoising approach that prioritized the boundaries of an image while simultaneously preserving its essential features. This approach marked a groundbreaking innovation, distinctively addressing the staircasing effect that was noted as a limitation in early diffusion processes.
Simultaneously, Stable Diffusion Inpainting has seen explicit evolution in treating varying degrees of degradation within an image. The innovation of Multiscale Mathematical Morphology (MMM) branched off from traditional mathematical morphology while retaining its structure, to tackle problems related to complex noise distribution and multi-level inpainting.
A recent stride in the trajectory of Stable Diffusion Inpainting has been the integration of deep learning algorithms, accelerating the development, and application of inpainting processes. Techniques like Convolutional Neural Networks (CNN) and Generative Adversarial Networks (GANs) have been harnessed to improve their efficiency, thereby lending a more extensive set of benefits.
In conclusion, examining the evolution of Stable Diffusion Inpainting readily reveals a history of relentless refinement and innovation. The progress made so far rests solidly on the synergistic confluence of mathematical understanding, algorithmic wisdom, and technological leverage. A myriad of advancements have successfully harnessed the principles of diffusion processes, optimizing them for desired accuracy and excellence in digital image processing. These evolutions not only underpin Stable Diffusion Inpainting’s significance, but also points toward the enthralling potential the future holds for this invaluable process.
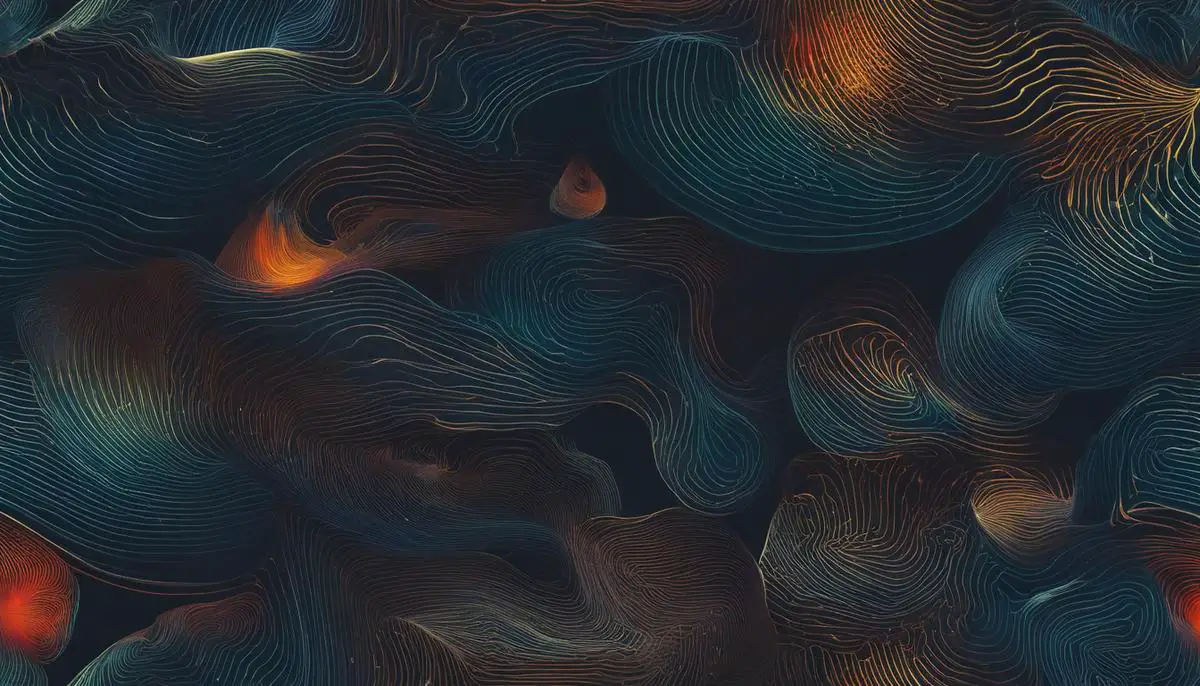
Current Implementations and Applications of Stable Diffusion Inpainting
Venturing beyond traditional applications, the versatility of Stable Diffusion Inpainting (SDI) has been harnessed across remarkably distinct domains. The suite of capabilities provided by the method, including noise reduction, image enhancement, and restoration, has been notably employed in the satellite imaging sector as well. The precise and quality outputs produced by SDI are essential for acquiring accurate data about Earth’s topography, weather conditions, and other geographical attributes.
SDI also finds prominence in video surveillance where real-time monitoring translates into massive amounts of data. The attrition of video data due to transmission errors, loss of data packets, or exposure to adverse weather conditions renders significant portions of video data ineffective. Here, SDI emerges as a rescuer, repairing and refining affected parts of a video to retain continuity and comprehensibility. The algorithm’s adaptability to varying scales makes it ideal in this context.
The world of cinematography has also benefited greatly from Stable Diffusion Inpainting. Old film restoration, removal of unwanted elements, and scene rectification have been technologically upgraded with the use of SDI, replacing earlier rudimentary, and often damaging, techniques.
On an industrial level, visual inspection systems extensively utilize SDI to rectify structural anomalies. In these systems, a smooth, consistent appearance is paramount; any inclusion, scratches, or noise can lead to false alarm triggers. With SDI, these distractions can be minimized or completely removed from the digital representation. Its unparalleled precision, coupled with its capability to process large-sized images, makes it an effective tool for industrial automation and quality control.
In the domain of environmental science, SDI has demonstrated immense potential. For instance, it has been effectively used to refine noise-afflicted thermal images which are crucial in understanding global warming patterns, real-time monitoring of forest fires, and remote sensing of volcanic activities. The robust algorithm efficiently restores the details of the affected regions, enabling a precise interpretation of the underpinning phenomena.
A truly revolutionary application of Stable Diffusion Inpainting is in the embryonic field of deep-fake detection. The advent of artificial intelligence has made digital manipulation of images and videos easier and almost imperceptible to the naked eye. Here, the SDI technique can be used to discern the ‘inpainting spots’ that often mark the seams of deep-fake manipulations.
In conclusion, the usage of Stable Diffusion Inpainting extends across a variety of fields. Its applications are not only numerous but also innovative, exhibiting a symbiosis of science, technology, and mathematics. The future holds immense promise for SDI, with emerging fields like virtual reality, augmented reality, and robotics standing to benefit from image manipulation and restoration techniques. As researchers continue to enhance and fine-tune this remarkable tool, it stands as a testament to the unwavering pursuit of human innovation and ingenuity.
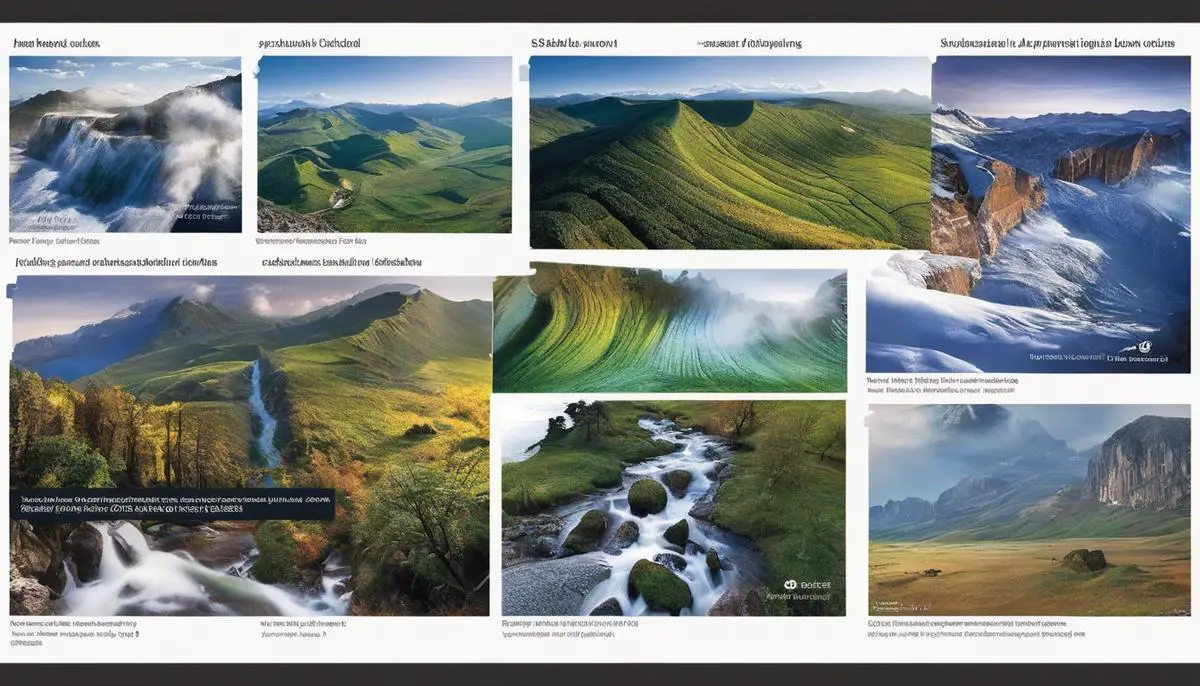
Future Prospects and Directions of Stable Diffusion Inpainting
The potential for Stable Diffusion Inpainting (SDI) in scientific research and practical applications is vast, reaching beyond image processing towards myriad facets of life. A still largely unchartered territory is the utilization of SDI in satellite imaging. By deploying SDI techniques in conjunction with satellite imaging, it becomes possible to create clearer, more accurate representations of geographical features and climatic changes, thereby contributing to meteorological advances and environmental conservation efforts.
SDI’s precision and adaptability can be exploited to remove noise, enhance images and restore them to their original form. Subsequently, the technology holds substantial promise in video surveillance and real-time monitoring systems. Here, SDI’s proficiency in refining imagery can engender more accurate threat detection, consequently boosting the effectiveness of security measures.
Given the world’s increasing immersion in visual content, an intriguing application of SDI is in the realm of cinematography and film restoration. By leveraging the suite of techniques underpinning SDI, the restoration of aged or degraded film content can be achieved with remarkable precision, allowing the resurrection and preservation of invaluable pieces of cinematic history.
In the industrial sphere, visual inspection systems incorporating SDI could yield significant improvements in quality control practices. By honing in on minute variations or aberrations, potential issues can be spotted and rectified promptly, thereby optimizing processes, preventing wastage and boosting overall productivity.
On the environmental front, SDI can be utilized to sharpen thermal images, enabling more detailed study of heat distribution patterns in the environment. It can also facilitate the monitoring of natural or man-made phenomena with greater resolution, thus yielding more accurate data for environmental studies.
The finesse of SDI is particularly useful in the rapidly evolving field of deep-fake technology. Manipulated media, crafted to be misleading, often carry imperfections that can be detected and analyzed using SDI techniques. This means SDI could play a central role in the ongoing battle against the dissemination of false information.
Looking further towards the future, the potential incorporation of SDI in burgeoning fields such as virtual reality (VR), augmented reality (AR), and robotics seems likely. For VR and AR, the refinements brought by SDI can significantly enhance the immersive experience, making it more captivating and indistinguishable from reality. In robotics, improved image processing capabilities can enhance machine vision, enabling robots to navigate environments with enhanced precision and sensitivity.
In conclusion, just as adaptation is the essence of evolution, so is true for Stable Diffusion Inpainting. Its ability to refine and innovate could contribute to great strides in multiple sectors, weaving it into the fabric of future scientific discovery and technological progress, and subsequently, enriching human life.
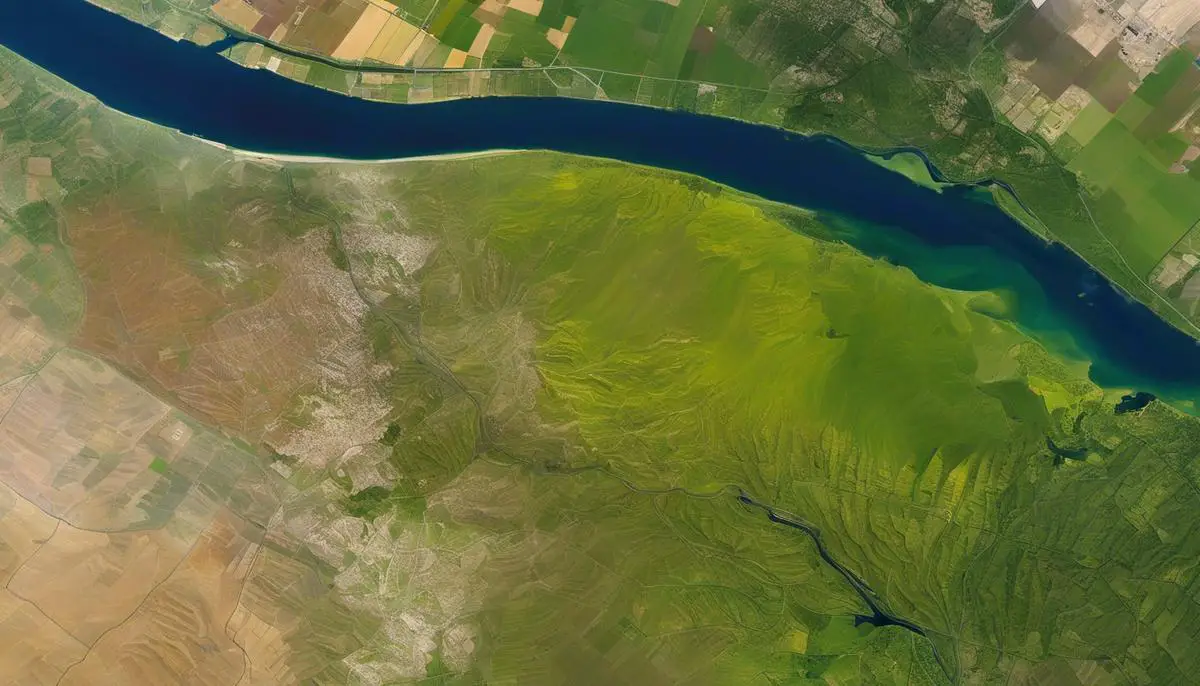
Through the exploration of the evolution of Stable Diffusion Inpainting, it becomes apparent that this technique holds tremendous promise. Its versatility and multifaceted applications, ranging from the restoration of antiquated photographs to the cutting-edge technology in various industries, underline its relevance and potential. Moreover, continuous advancements and research point towards an exciting future. Whether it is achieving new levels of image refinement or breaking fresh ground in areas still undiscovered, Stable Diffusion Inpainting stands as a cornerstone in the ever-evolving sphere of image processing and related sciences.
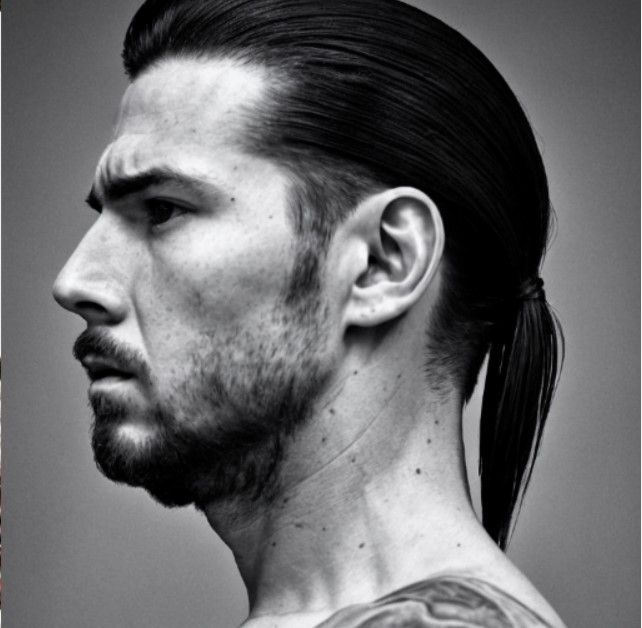
Emad Morpheus is a tech enthusiast with a unique flair for AI and art. Backed by a Computer Science background, he dove into the captivating world of AI-driven image generation five years ago. Since then, he has been honing his skills and sharing his insights on AI art creation through his blog posts. Outside his tech-art sphere, Emad enjoys photography, hiking, and piano.