Within the ever-evolving world of digital image processing, incredible strides were taken towards advancing and refining the concept and application of image-to-image diffusion. As technological developments continue to reshape our understanding and utilization of digital imagery, the phenomenon of img2img diffusion has emerged as a key player.
This exploration delves into the intricacies of img2img diffusion, unpacking its fundamental concepts, unveiling its application in various sectors, and critically discussing its current limitations and forecasted improvements. Throughout this journey, we affirm the value of img2img diffusion in our digital future, offering a comprehensive approach to understanding its complexities and potentials.
Contents
Understanding Img2Img Diffusion
Img2img Diffusion in Image Processing: A Crucial Pursuit of Excellence
Img2img diffusion, also known as image-to-image diffusion, stands as a pivotal area of research within the expansive realm of image processing. This technique is primarily premised on altering an original image through a carefully controlled image diffusion procedure, consequently achieving a specific target image.
Image diffusion techniques sketch their foundations from the theory of partial differential equations. This mathematical-based foundation is particularly essential in enabling the delineation of solutions that not only respect the structure of the images being processed, but also maintain salient visual details within the target image.
Img2img diffusion is composed of a sequence of Gaussian diffusion processes. In essence, the original image undergoes a cascade of adjustments where each recursive step contributes to the spatial transformation of the image, guiding it one step closer to the target image.
Exploration into img2img diffusion is a critical venture as it can significantly contribute to the enhancement of myriad applications in image processing. This can range from medical imaging—where img2img diffusion can help differentiate between normal and abnormal tissues—to the field of computer vision—where this diffusion can be deployed to clear image noise and enhance edge detection.
One of the most noteworthy aspects of img2img diffusion is its consummate ability to preserve the integrity of the original image. Despite the application of various alterations to achieve the desired outcome, this method inherently ensures that the fundamental structure of the original image remains intact.
This characteristic is a vital prerequisite for specific areas of application, such as forensic imaging and heritage preservation, where interfering with the original form of the image is strictly forbidden.
As research into the domain of img2img diffusion gains momentum, it also invites challenges that require existential solutions. One of these pertains to the ambiguity that can arise during the diffusion process. While the method ensures the preservation of original image integrity to a large extent, there can be instances where the process produces multiple plausible outputs that maintain an equal resemblance to the original image.
Creating a clear yardstick to measure the success of the diffusion process, and thereby reduce such ambiguity, is a challenge that research in this field aims to overcome.
Img2img diffusion emerges as a game-changer in image processing, promising enhancements in image modification that form key to many sectors from medicine to forensics. Through continued research, it is inevitably a mission that holds the torch aloft for the future of image processing, blending the elegance of mathematics, the precision of computing, and the aesthetics of visual perception, igniting the effervescent curiosity inherent in the field of digital imaging.
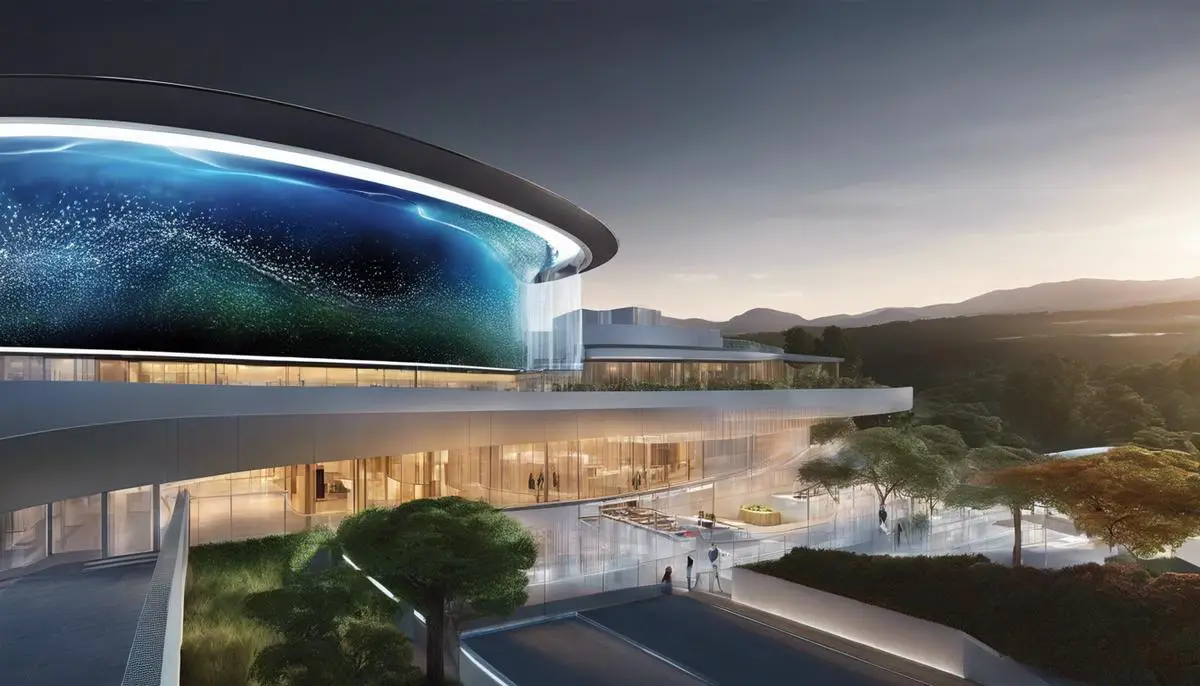
Techniques and Algorithms in Img2Img Diffusion
Img2img diffusion, a fast-evolving field within image processing, relies heavily on a plethora of state-of-the-art algorithms and methodologies. Two of the principal techniques powering img2img diffusion are the Perona-Malik diffusion model and the edge-based diffusion model, both employing partial differential equations to perform subtle, deliberate alterations to the original image.
The Perona-Malik diffusion model stands out for its proficiency in simultaneously reducing noise while preserving edge features within images, a significant improvement over previous models burdened by susceptibility to undesirable side effects such as staircase effect or over-blurring. Contrary to the common approach of globally treating all pixels identically, this model introduces a shift. It factors in local information in each pixel, adjusting the diffusion amount according to its context, mitigating the loss of important details.
Consider the image as a terrain map, with pixel intensity representing altitude. The diffusion process in the Perona-Malik model can be likened to rain slowly eroding this landscape. But instead of uniformly eroding all surfaces, it preferentially erodes lower gradient areas (flat regions) while safeguarding high-gradient zones (edges). This neatly translates to noise reduction without significant data loss, an eloquent solution to a complex problem.
On parallel lines, the edge-based diffusion model introduces some novelty. Rather than focusing on pixel intensity, this model beautifully leverages edge direction information within the image for diffusion. Essentially, the diffusion process now has an overall purpose of bolstering the edge features, promoting an image clarity unseen with previous models. It radically changes the image by guiding diffusion along the edge direction instead of across, which restrains the blurring effect on the edges, thereby retaining all integral components of the original image.
In practice, these methodologies are implemented algorithmically. A random walk algorithm has been notably effective in this regard. In the context of image diffusion, this Monte Carlo method operates by hopping from pixel to pixel, with the direction and step size determined probabilistically by their intensities. Stepping on a brighter pixel implies a larger step, hence speeding up the diffusion process and demonstrating ample efficiency.
Moreover, innovative techniques leveraging convolutional neural networks have begun to emerge. Training these networks on vast image sets and allowing them to learn to mimic diffusion processes has led to breakthroughs, blurring the line between classic physics-based diffusion and data-centric artificial intelligence methods.
Undeniably, the landscape of img2img diffusion is influenced by a robust collection of algorithms and techniques, all ushering this realm of image processing to a promising future.
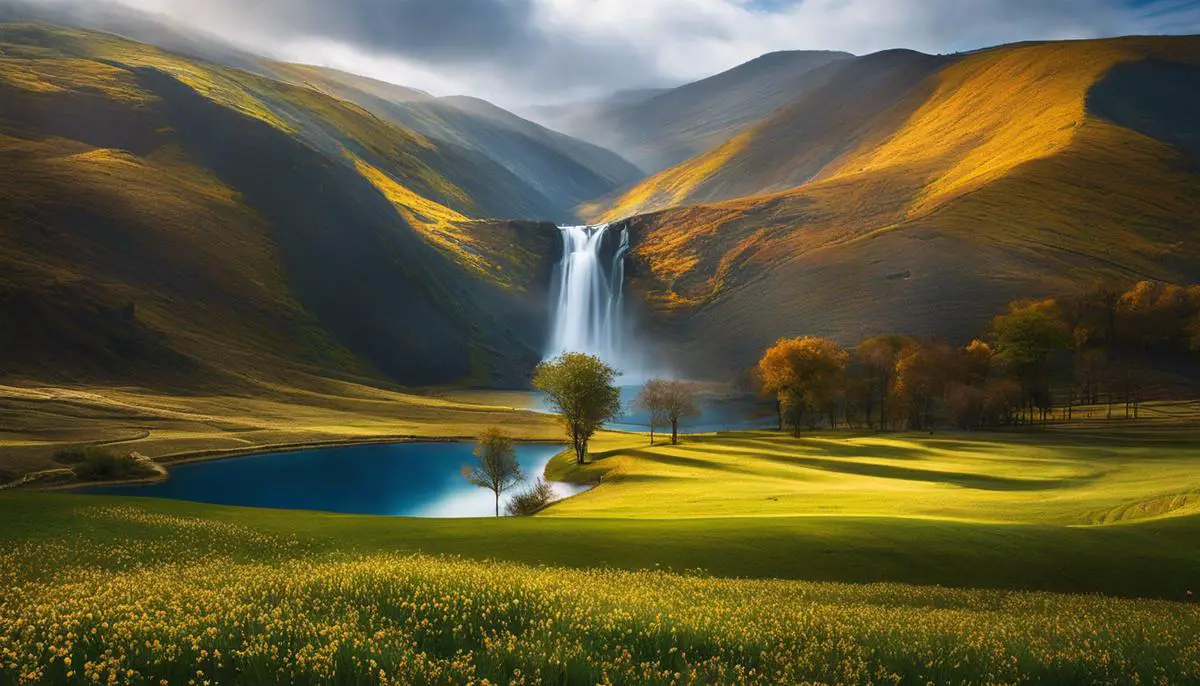
Emerging Trends in Img2Img Diffusion
Emerging research in the realm of img2img diffusion has made significant progress recently, particularly in the domain of diffusive image reconstruction. Theoretical and practical expansions in the field have contributed to advancements in current methodologies, enabling improvements in image-to-image diffusion technology.
Recent seminal work has introduced the concept of learned diffusions. By training convolutional neural networks algorithmically and teaching the constructs to mimic the diffusion process, not only does this provide an unexpected tool to handle img2img diffusion, but it also demonstrates how artificial intelligence can be seamlessly integrated with traditional physics-based methodologies.
Going further, a novel edge-based diffusion model has been created that provides more precise results than the Perona-Malik model. This new model utilizes information about edge directions and diffuses alongside the edges, rather than across them. This process adheres to the principle of “preserving edges while reducing noise”, an essential factor in image processing.
This goes hand in hand with the concept that the diffusion process is fundamentally a random walk algorithm. New research developments have gleamed insight from the model of rain erosion, allowing for diffusion to be conceptualized similarly. Within this paradigm, the wind (or the edges of the image) guide the path of the rain (or the movement of the pixel values), carving a path that is both random and guided simultaneously. Interesting refinements have been made to this method, with the use of Monte Carlo simulations playing a key role in the probabilistic determination of each step in the process.
Furthermore, studies have extensively focused on the preservation of original image integrity, an essential aspect of img2img diffusion. When considering the objective goal of reducing noise while simultaneously preserving the edges of an image, the delicate balance between local and global thresholds comes into play. This area has seen meaningful advancements by integrating local information and context during pixel diffusion and enhancing the authenticity of the result.
The trend of imbuing convolutional neural networks into the img2img diffusion procedure has displayed immense potential. Combining AI and physics-based methodologies in image diffusion has amplified the capacity of systems to interactively learn and modify diffusion processes. Training these networks through machine learning algorithms has shown a promising direction for future advancements in image processing technology.
Indeed, the exciting prospects in this domain reflect not only the rich interplay between mathematical constructs, such as partial differential equations and Monte Carlo methods, but also open-collaboration between traditional and futuristic approaches, such as AI.
Emerging trends and recent advancements in img2img diffusion technology impressively represent an intersection where mathematical precision, innovative technology, and luminary scientific thought converge. These groundbreaking discoveries and developments consistently continue to reach new heights in enhancing, innovating and alleviating the challenges faced in image processing.
An unfinished story, the tale of img2img diffusion continues to unfurl. With unexplored territories on the horizon, the future of this fascinating field certainly promises a blend of challenge, exhilaration, curiosity and awe-inspiring revelations.
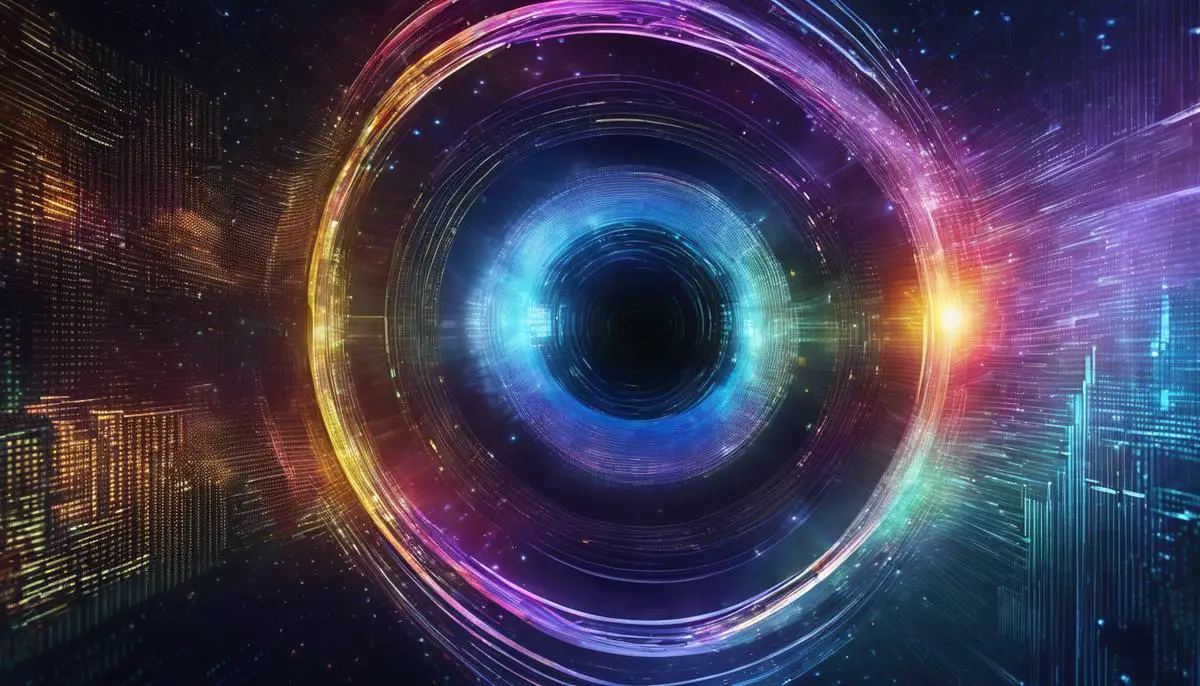
Challenges and Solutions in Img2Img Diffusion
While the field of img2img diffusion has seen considerable progress, it still presents distinct challenges that necessitate innovative solutions. These difficulties primarily arise from complexities surrounding computational feasibility, optimization of the diffusion coefficients, preservation of high-dimensional data, and striking an optimal noise-reduction balance.
Computational feasibility, a recurring hurdle in many domains of data science, likewise presents difficulties within img2img diffusion. The underlying mathematical complexities present in img2img diffusion algorithms, compounded by their iteration-based nature, often lead to high computational burdens. While advancements in parallel computing and GPU-accelerated algorithms offer new pathways to deal with these issues, the requirement for high performance computing resources continues to pose a significant obstacle to comfy execution of img2img diffusion processes in resource-constrained environments.
Then there is the difficulty of optimizing diffusion coefficients, an integral aspect of the img2img diffusion process. Striking a balance between sharpening important features in an image and minimizing noise is a delicate task. Non-optimal coefficients can resulted in over-smoothed images, losing important features or under-smoothed images accentuating noise. Research aimed at developing adaptive methods to automate the process of coefficient determination is currently ongoing, but more progress is needed to fully address this challenge.
Thirdly, there is the issue of high-dimensionality data. As imaging technologies continue to evolve, higher dimensional data, including 3D imagery and time-series are increasingly being incorporated into image processing tasks. Handling this high-dimensional data in img2img diffusion processes is non-trivial due to increased complexity and scale of data. Although new algorithms and frameworks are being developed to accommodate high-dimensional data, seamless implementation continues to be a significant challenge.
Lasty, the inherent tumultuous tension between noise reduction and preservation of edge features exists within img2img diffusion. Whilst diffusion processes are adept at smoothing images and consequently reducing noise, this inadvertently risks blurring edge features. This poses a substantial difficulty in sectors such as medical imaging, where these detailed edge features carry pivotal information.
Addressing these challenges requires an infusion of interdisciplinary research and advancements. More sophisticated and efficient algorithms are needed to handle computational complexities, while adaptive, dynamic techniques should be explored to optimize diffusion coefficients.
Furthermore, new methods that can handle the complexity and scale of high-dimensional data will increase the generalizability of img2img diffusion. Lastly, attention must be dedicated to developing innovative diffusion methods that strike a balance between reducing noise and preserving crucial edge features.
The field of img2img diffusion continues to advance rapidly, with ongoing research working relentlessly to surmount these challenges, shedding light on the promising future of img2img diffusion technology.
Application of Img2Img Diffusion in various fields
In addition to the already emphasized spheres of image processing, the application scope of img2img diffusion extends far beyond. One such groundbreaking domain is cartography and geographic information systems (GIS).
Img2img diffusion serves as an integral cog in the machinery of satellite image processing that powers the creation of detailed modern maps. It provides improved reduction of noise and distortions while efficaciously preserving edge features in topographical patterns—enhancing the intelligibility and precision of satellite-generated topographies.
Moreover, the arena of astronomy hugely benefits from img2img diffusion. Deep-space imaging, characterized by sparse light and cosmic background noise, mandates optimal noise reduction without compromising on intricate stellar formations. By tweaking the diffusion coefficients to match these unique circumstances, img2img diffusion advances the analysis of astronomical data—contributing to the discovery of new galaxies, exploration of black holes, and study of cosmic microwave background radiation.
In another context, the field of arts and restoration experiences an encouraging upshot from img2img diffusion. Aging artefacts and valuable artistic pieces often suffer from deterioration, cracks, or noise interference. By applying img2img diffusion, these distortions can be counteracted while preserving the integrity of original artistic details, effectively aiding in the digital restoration and conservation of cultural heritage.
The technique has also proved impactful in the realm of forensics—and more specifically, in facial recognition for security and criminal investigations. The technology successfully reduces noise and unwanted artifacts in CCTV footage or still images, while preserving the critical edge features necessary for accurate facial recognition—a resource of paramount importance within law enforcement agencies.
In terms of impact, img2img diffusion is fundamentally altering the way these fields have traditionally processed image data—enhancing quality, reducing noise, and ensuring the preservation of crucial details. This has facilitated the amelioration of diagnostic methodologies in medical imaging, enriched astronomical studies, expedited the charting of Earth’s geography, and optimized facial recognition technology in forensics.
Reflecting on the future of this technology, promising research is underway. Reinforcement learning, a frontier in AI technology, is being integrated with established img2img diffusion frameworks to further enhance the adaptability and efficacy of the diffusion process. This has potentialities ranging from real-time video enhancement to the creative generation of art based on advanced AI algorithms.
Deep learning methodologies, including convolutional neural networks, promise to boost img2img diffusion’s efficiency in high-dimensional landscapes. By building neural networks that can more effectively mimic, learn, and improve upon traditional diffusion algorithms, the process of handing complex image data might be significantly expedited. The iterative exploration and fine-tuning of these network architectures by dedicated researchers will only advance our understanding and proficiency in img2img diffusion—launching us towards countless more innovative applications in the foreseeable future.
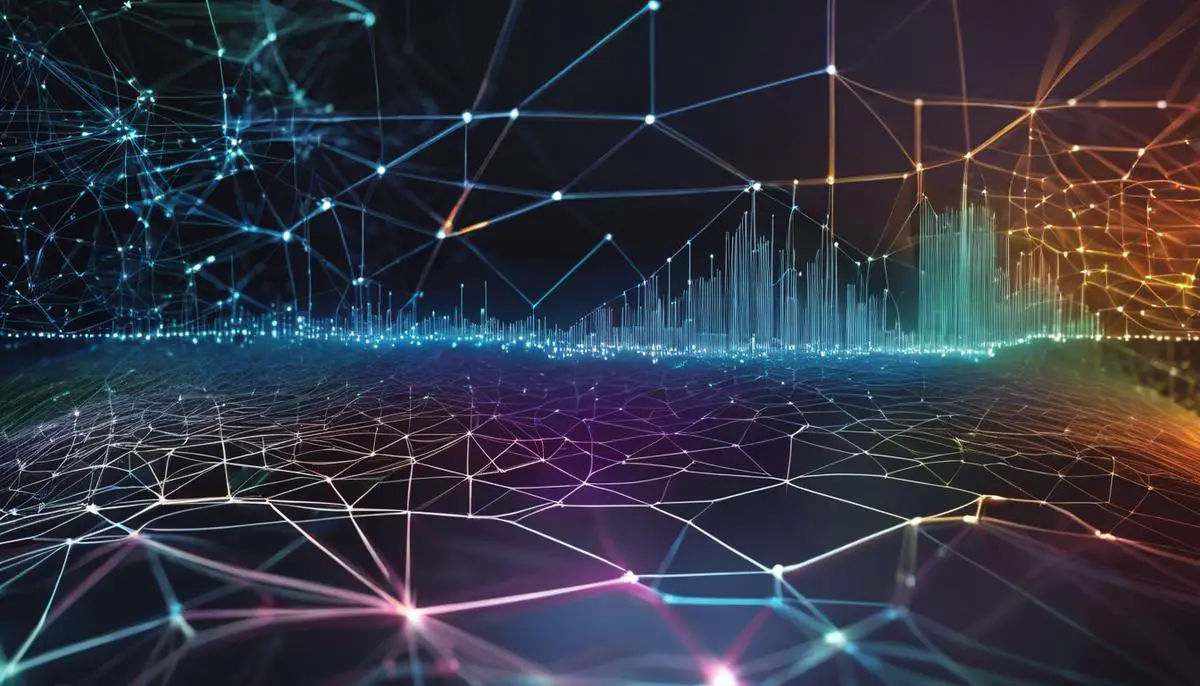
Harnessing the full potential of img2img diffusion is a task that continually stretches the boundaries of digital image processing. This exploration delved into the depth of this fascinating topic, demystifying algorithms, discussing evolving trends, and presenting solutions to persisting challenges.
Moreover, by shedding light on the prolific application of img2img diffusion across different fields, the implications for future advancements and adaptations are profound. As we navigate the evolving future of digital technology, img2img diffusion stands as a testament to the ingenious human capacity to adapt, innovate, and conquer previously unimaginable frontiers.
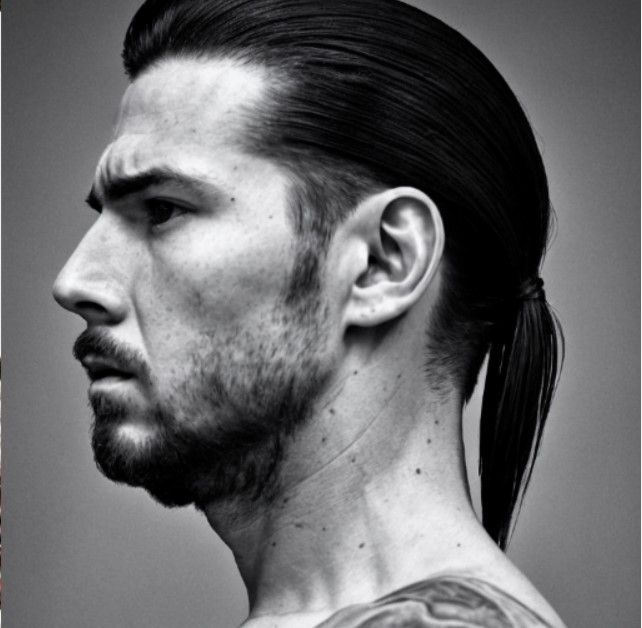
Emad Morpheus is a tech enthusiast with a unique flair for AI and art. Backed by a Computer Science background, he dove into the captivating world of AI-driven image generation five years ago. Since then, he has been honing his skills and sharing his insights on AI art creation through his blog posts. Outside his tech-art sphere, Emad enjoys photography, hiking, and piano.